Meltwater Sentiment Analysis: Key Insights for Businesses
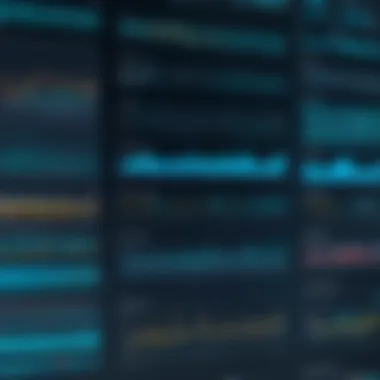
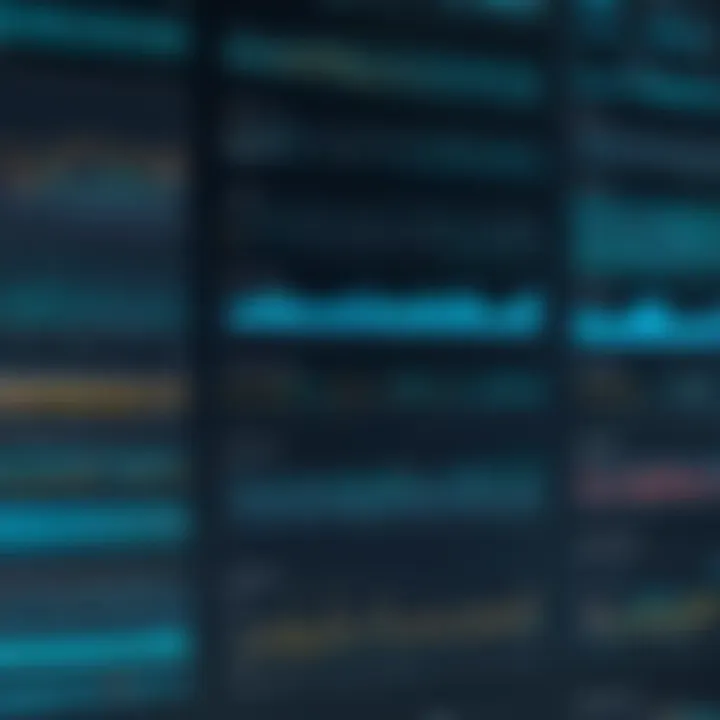
Intro
In today's fast-paced digital world, sentiment analysis plays a crucial role in understanding public opinion. Meltwater, a leader in media intelligence, offers advanced sentiment analysis tools that help organizations derive valuable insights from vast data sets. This analysis is not merely about understanding positive or negative sentiments; it delves deeper into customer perceptions, emotions, and trends. Businesses can significantly benefit by integrating Meltwater's technology into their strategic decisions.
By evaluating the data collected from various channels, companies track brand performance and market trends. They can detect shifts in public sentiment almost in real-time, allowing them to react promptly. This capacity for swift analysis helps organizations stay competitive amidst constantly evolving market dynamics. The subsequent sections will explore the features, methodologies, and impact of Meltwater sentiment analysis.
Preamble to Meltwater Sentiment Analysis
Understanding how public opinion affects businesses has never been more critical. Meltwater sentiment analysis plays a significant role in this domain. It allows organizations to harness data-derived insights that inform strategies and decisions. As market dynamics evolve, the importance of grasping public sentiment turns paramount. Companies that utilize sentiment analysis can better navigate challenges and capitalize on opportunities.
Definition of Sentiment Analysis
Sentiment analysis refers to the process of determining whether a piece of text expresses positive, negative, or neutral sentiment. It involves the use of Natural Language Processing (NLP) tools and techniques to analyze written data. This analysis can come from various sources, such as social media, news articles, or customer reviews. The strength of sentiment analysis lies in its ability to provide insights on public perception, offering a semblance of the general attitude towards a brand or topic.
Understanding sentiment allows organizations to track changes in public opinion over time. It also provides insights that go beyond surface-level perceptions, enabling a more nuanced understanding of consumer attitudes. The methodologies undertaken in sentiment analysis can produce different results based on the algorithms and approaches used.
Overview of Meltwater
Meltwater is a media intelligence and analytics company specializing in monitoring public sentiment across various platforms. It collects data from a multitude of sources, including social media and news. Meltwater's tools enable organizations to gauge how their brands are perceived and how their competitors are viewed in the marketplace.
The company provides a suite of services that helps businesses understand market trends and consumer behavior. By leveraging modern technologies, Meltwater distills vast amounts of data into actionable insights. Organizations can customize their analyses based on industry-specific parameters. This flexibility contributes to the value of Meltwater's insights as they adapt to changing market needs.
Data Sources for Sentiment Analysis
Understanding the data sources for sentiment analysis is vital. This provides the foundation upon which insights and decisions are made within organizations. By examining diverse platforms where public sentiment is expressed, businesses can derive meaningful conclusions about consumer attitudes. The right data source can highlight trends, capture the mood, and present opportunities for engagement.
Social Media Platforms
Social media has become a prominent channel for public expression. Platforms such as Facebook, Twitter, and Instagram allow users to share thoughts, opinions, and feelings extensively. This real-time interaction presents a rich dataset for sentiment analysis.
The primary benefit of using social media data is its volume and immediacy. Organizations gain insights into how consumers react to campaigns, news, or events almost instantly. Additionally, social media amplifies voices, especially from influencers or trending topics, which can shift public perception rapidly. However, analyzing this data comes with challenges. Textual data can be noisy, filled with slang, abbreviations, and emojis, which complicates understanding sentiment accurately.
Some key aspects to consider when using social media data include:
- Volume of Data: Large amounts of user-generated content are available for analysis.
- Timing: Insights can be obtained in near real-time, allowing for swift business decisions.
- Sentiment Volatility: Trends may change quickly; therefore, continuous monitoring is essential.
News Articles and Blogs
News articles and blogs represent another critical layer of sentiment analysis data sources. Content from reputable news sources can give a broader view of public sentiment, especially around significant events or crises. Blogs often capture niche opinions that may not be evident on mainstream media.
Articles can portray emotional sentiments that are not merely headlines. By analyzing tone, language patterns, and context within the articles, organizations can gauge public sentiment effectively. Using text from news and blogs allows for the understanding of how narratives shift over time. However, the challenge is discerning bias in reporting and ensuring a balanced view is represented.
Considerations for gathering news data include:
- Authority and Bias: Assess the credibility of articles.
- Contextual Depth: Articles often provide background and context essential for comprehensive analysis.
- Topic Relevance: Ensuring the articles are relevant to the specific sentiment being measured.
Customer Reviews and Feedback
Customer reviews play a crucial role in sentiment analysis, particularly for product and service evaluations. Textual feedback from platforms like Amazon or Yelp can reveal much about customer satisfaction and brand perception.
Reviews are generally more straightforward, as they often express clear positive or negative sentiments. This data is vital for understanding how products are performing in the market. Additional feedback loops can emerge from analysis, informing businesses of potential areas for improvement. However, challenges like fake reviews or biased ratings must be addressed.
For collecting and analyzing customer feedback, consider:
- Clarifying Questions: Implementing structured feedback forms can help gather more detailed insights.
- Rating Variability: Analyzing variances in numerical ratings provides context that text alone may not.
- Consumer Trends: Continuous analysis can surface emerging trends in customer preferences over time.
"Data is the new oil. It’s valuable, but if unrefined, it cannot really be used."
Methodologies in Sentiment Analysis
In the realm of Meltwater sentiment analysis, the methodologies employed play a pivotal role. These methodologies provide the framework through which vast amounts of data can be interpreted. Businesses rely on these methods to derive actionable insights from consumer opinions and market sentiment. Understanding these methodologies is crucial for effective brand management and strategic planning.
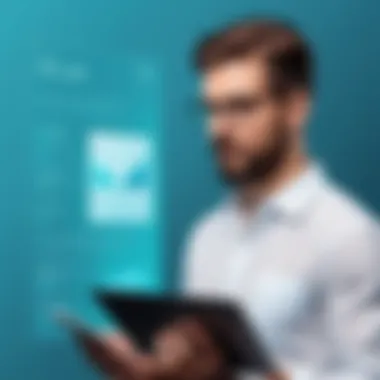
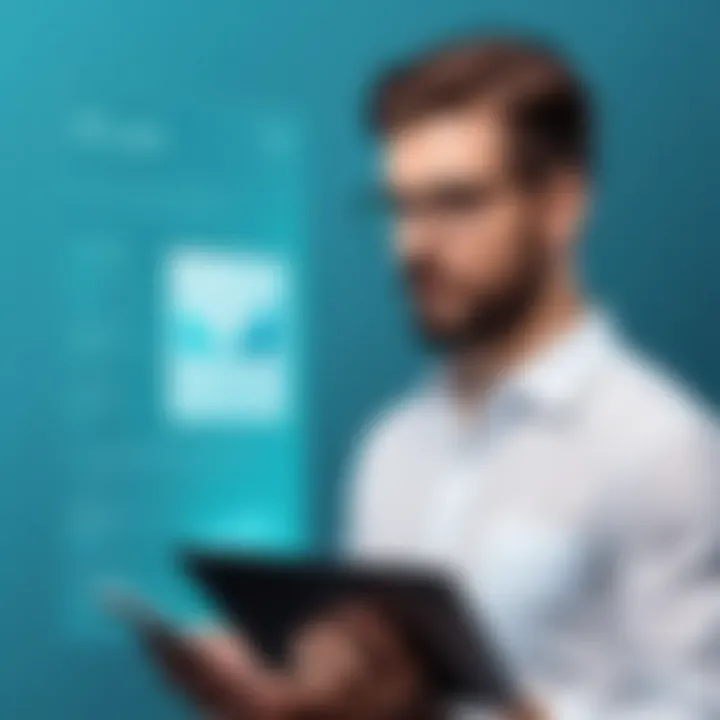
Natural Language Processing Techniques
Natural Language Processing (NLP) techniques are fundamental in sentiment analysis. They bridge the gap between human language and computer understanding. NLP encompasses a variety of processes, from parsing text to sentiment classification. By employing techniques such as tokenization, stemming, and parsing, organizations can extract meaningful data from unstructured text information.
For instance, tokenization divides text into individual words or phrases, making it easier to analyze. Stemming reduces words to their root form, enhancing consistency in data interpretation. These techniques allow for the identification of sentiment in sentences effectively, whether positive or negative.
Machine Learning Algorithms
Machine learning algorithms are another significant dimension of sentiment analysis. These algorithms learn from historical data to predict outcomes based on new input. Various types of machine learning methods, including supervised and unsupervised learning, can be applied to sentiment analysis.
In a supervised learning context, labeled datasets are used to train models, which then predict the sentiment of new, unlabeled instances. Examples of popular algorithms include Logistic Regression, Support Vector Machines, and Decision Trees. Conversely, unsupervised learning can uncover patterns in data without pre-existing labels. Clustering techniques, such as K-means, can categorize sentiments effectively without explicit direction.
Employing machine learning for sentiment analysis allows businesses to scale their analysis. This capability is essential for processing the immense volume of data generated on social media and other platforms.
Lexicon-Based Approaches
Lexicon-based approaches rely on predefined lists of words and their associated sentiments. In this methodology, each term in the list is assigned a score reflecting its emotional weight. A comprehensive sentiment lexicon can include thousands of words, categorized by sentiment polarity—positive, negative, or neutral.
When analyzing text, the approach tallies the scores of the words present. This method is straightforward and interpretable. It can be particularly effective in scenarios where the context is clear. However, it does have limitations. It struggles with ambiguous language and contexts in which sentiment is more nuanced.
Despite its limitations, lexicon-based approaches are useful tools in certain situations. They provide quick insights and are easy to implement as a baseline for more complex methods.
In summary, methodologies in sentiment analysis are essential for navigating the complexities of consumer feedback. You can not fully grasp sentiment without engaging with these techniques. By leveraging NLP, machine learning, and lexicon-based methods, organizations can develop a robust sentiment analysis framework.
Key Metrics in Sentiment Analysis
Understanding the key metrics in sentiment analysis is crucial for extracting meaningful insights from the vast amounts of data collected. These metrics provide a quantitative assessment of public sentiment, allowing organizations to make informed decisions. Two primary metrics often highlighted are polarity scores and subjectivity scores.
By measuring these aspects, businesses can better grasp public opinion and respond accordingly. The implications of these metrics affect marketing strategies, customer relationship management, and overall brand positioning. Consequently, organizations leverage these scores to not only analyze current sentiment but also predict future trends in consumer behavior.
Polarity Scores
Polarity scores play a vital role in quantifying the sentiment expressed in textual data. This metric is typically measured on a scale, often ranging from -1 to +1. A score of -1 indicates a strongly negative sentiment, while a score of +1 signifies a strongly positive sentiment. A score close to zero reflects neutrality.
Polarity scores offer several benefits:
- Clarity: They simplify the understanding of sentiment trends. A quick glance at the scores can reveal shifts in public opinion.
- Actionable Insights: These scores help marketing teams identify potential issues or highlight positive sentiments, allowing for timely interventions.
- Data-Driven Strategy: By consistently monitoring polarity scores, businesses can refine their strategies based on real-time feedback.
However, one must be cautious about potential pitfalls, such as over-relying on these scores without considering the context. For instance, sarcasm or cultural references might distort the numerical value. Therefore, it process of interpreting these scores requires nuanced understanding.
Subjectivity Scores
Subjectivity scores complement polarity scores by assessing how objective or subjective a statement is. This metric typically ranges from 0 to 1, where a score closer to 1 indicates a higher level of subjectivity, and a score closer to 0 indicates objectivity.
This distinction is significant for several reasons:
- Content Analysis: Understanding if a statement is subjective allows businesses to gauge customer emotions versus factual information.
- Market Understanding: High subjectivity in consumer feedback can indicate emotional engagement, critical for crafting personalized marketing messages.
- Enhancing Clarity: By combining polarity and subjectivity scores, organizations can create a clearer picture of public sentiment. This can lead to more targeted and effective campaigns.
In summary, both polarity and subjectivity scores are essential metrics in sentiment analysis. They provide organizations with valuable insights into public sentiment, enabling informed decision-making and strategic planning. In a rapidly changing market landscape, understanding these metrics can define a brand’s success.
Applications of Meltwater Sentiment Analysis
The applications of Meltwater sentiment analysis are essential in bridging the gap between data and actionable insights for organizations. In a landscape where consumer opinions can shift rapidly, understanding sentiment becomes crucial. Sentiment analysis allows companies to stay ahead of trends and maintain alignment with public perception. This involves leveraging algorithms and methodologies to analyze large volumes of data from various sources—social media, news articles, and customer feedback—to extract meaningful sentiment indicators.
Brand Health Monitoring
Brand health monitoring is a critical application of Meltwater sentiment analysis. It enables organizations to evaluate their reputation and brand sentiment over time. By analyzing mentions across various platforms, companies can determine how they are perceived by their audience. This ongoing assessment helps in identifying both positive and negative sentiment trends.
For instance, if a notable decline in positive sentiment is detected after a product launch, a company can respond quickly to address issues. Regular tracking supports proactive management of brand reputation. Benefits include:
- Spotting Trends: Companies can capture emerging trends in consumer sentiment that may impact brand perception.
- Competitor Benchmarking: Sentiment analysis can compare a brand's performance against competitors, revealing strengths and weaknesses.
- Informed Decision Making: Data-driven insights empower executives to make adjustments in strategy.
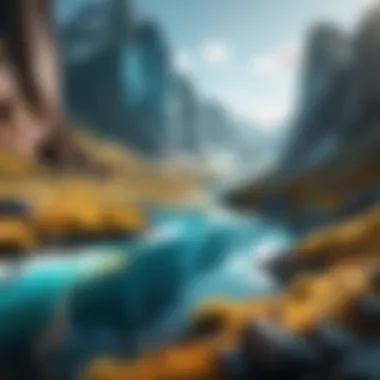
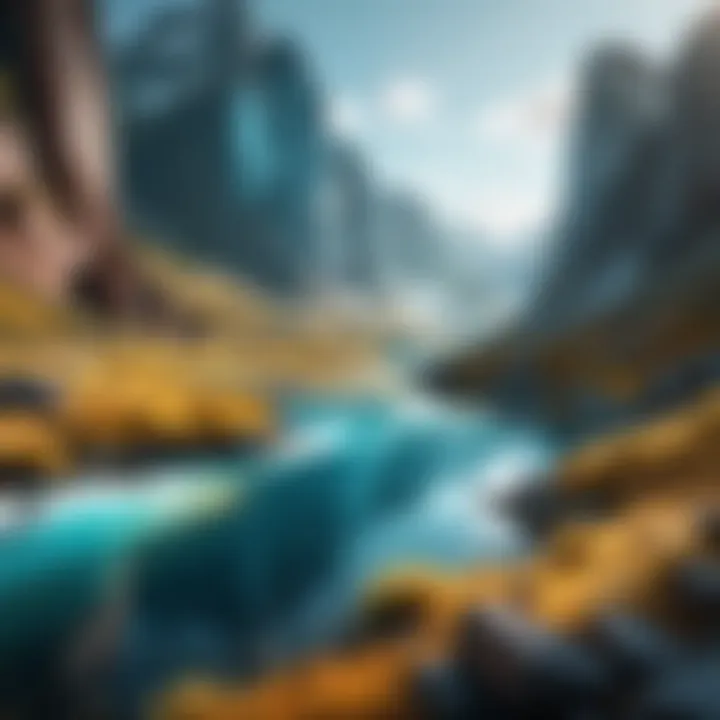
Market Research Insights
Market research insights derived from sentiment analysis offer an additional layer of understanding about consumer preferences and behaviors. By analyzing sentiment around specific products, services, or campaigns, organizations can make more informed decisions about market positioning. This form of analysis can highlight consumer concerns or preferences that traditional market surveys might overlook.
Key components to consider include:
- Feedback Analysis: Sentiments derived from customer reviews can guide product development or marketing strategies.
- Target Audience Understanding: Identifying demographic sentiment patterns assists in tailoring messages for specific audiences.
- Actionable Insights: This level of analysis can lead directly to actionable strategies that fulfill consumer demands.
Crisis Management Strategies
Crisis management strategies can significantly benefit from Meltwater sentiment analysis. By monitoring sentiment in real-time, organizations can identify potential crises before they escalate. Rapidly recognizing negative sentiment patterns allows for timely responses, often salvaging a brand's reputation.
This proactive approach includes:
- Immediate Response: Quick identification of negative sentiment enables brands to respond effectively and mitigate potential damage.
- Root Cause Analysis: Analyzing the sources of negative sentiment helps in understanding consumer issues more deeply.
- Reputation Recovery: Continuous monitoring post-crisis supports the recovery process, ensuring that sentiment improves over time.
"Sentiment analysis is not just about measuring sentiment; it's about understanding it to drive strategic actions."
In summary, the applications of Meltwater sentiment analysis empower businesses to monitor their brands continuously, gain market insights, and navigate crises with agility. These capabilities form a robust foundation for enhancing strategic decision-making, particularly in an era where consumer sentiment influences corporate success significantly.
Case Studies of Successful Sentiment Analysis
Examining real-world cases where sentiment analysis has significantly impacted business strategies can provide invaluable insights. Case studies serve not only to illustrate the application of Meltwater sentiment analysis but also to underline its effectiveness in diverse contexts. By analyzing how different companies utilize sentiment analysis to drive decisions, we can grasp the breadth of its potential benefits.
Examples from Fortune Companies
Fortune 500 companies often have access to vast amounts of data, making them prime candidates for implementing advanced sentiment analysis techniques. For example, Coca-Cola employs Meltwater sentiment analysis to monitor public perception of their brand. By tracking sentiment across various platforms, Coca-Cola can identify emerging trends and respond proactively.
- Real-Time Insights: Monitoring social media mentions allows Coca-Cola to gather real-time feedback, enabling a quicker response approach.
- Crisis Management: In situations where public opinion trends negatively, the company can act swiftly to mitigate damaging narratives.
- Brand Optimization: Positive sentiment patterns help inform marketing strategies, shaping campaigns that resonate more effectively with target audiences.
Another prominent example is Ford Motor Company. Through Meltwater’s tools, Ford analyzes customer sentiments relating to vehicle models and brand initiatives. This analysis helps the company target specific demographics more effectively.
- Targeted Marketing: Ford tailors marketing strategies based on insights into customer preferences drawn from sentiment data.
- Product Development: Feedback derived from sentiment analysis informs enhancements in vehicle design and features.
Insights from Startups
Startups face unique challenges in establishing themselves in competitive markets. Utilizing sentiment analysis can provide these smaller enterprises with a competitive edge. For instance, a tech startup that focuses on wearable health technology uses Meltwater sentiment analysis to understand users' feedback on their products.
- User-Centric Improvements: By analyzing customer reviews and social media mentions, the startup quickly identifies features that users appreciate and those that require improvement.
- Brand Building: As the startup builds its brand, it can leverage positive sentiments to develop marketing campaigns tailored to specific audiences.
Another startup, focused on eco-friendly products, utilizes sentiment analysis to gauge consumer attitudes toward sustainability. Understanding how their brand is perceived helps them craft more compelling narratives that align with customer values.
- Value Alignment: Insights from sentiment analysis facilitate the alignment of brand messaging with consumer expectations and preferences.
- Market Positioning: By identifying gaps in sentiment, the startup can position itself more effectively within a niche of environmentally conscious consumers.
These case studies highlight how both large corporations and agile startups benefit from Meltwater sentiment analysis. Companies can drive strategic decisions that align with public sentiment, ultimately enhancing their market positioning and fostering stronger customer relationships.
Challenges in Sentiment Analysis
Sentiment analysis can be a powerful tool, but it is not without its challenges. As organizations increasingly rely on this technology, understanding these challenges becomes essential. Recognizing the hurdles in effectively interpreting sentiment data allows teams to prepare and adapt their methodologies. This is crucial for ensuring that data-driven insights accurately reflect public opinion and can inform business strategy.
Dealing with Ambiguity
Ambiguity in language presents one of the greatest challenges in sentiment analysis. Words can have different meanings based on context, which complicates interpretation. For example, the term "light" can refer to something that is not heavy or a source of illumination. In sentiment analysis, it is crucial to extract the intended meaning behind such words.
Natural Language Processing (NLP) techniques aspire to clarify this ambiguity. Employing advanced algorithms can help disambiguate terms based on their contexts. Overall, understanding how words interact and form sentences is vital. It helps to develop a more accurate sentiment analysis that truly reflects the sentiment of a piece of text.
Sarcasm Detection
Sarcasm can skew sentiment analysis results significantly. Detecting sarcasm requires an understanding of not just words but also tone and cultural context. A statement like "Oh great, another rainy day!" might be positive on the surface, while the underlying sentiment is negative.
Creating effective detection models for sarcasm is an active area of research. Current methodologies often rely on training datasets containing sarcastic comments. However, due to the subjective nature of sarcasm, there isno guarantees that these models will always function accurately. This adds another layer of complexity to sentiment analysis, necessitating continuous refinement of these tools.
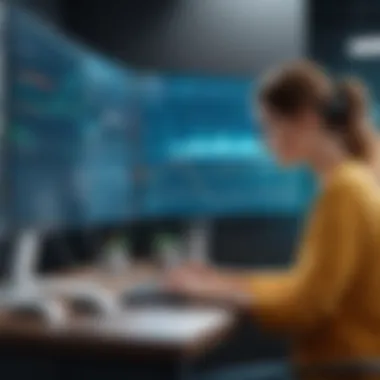
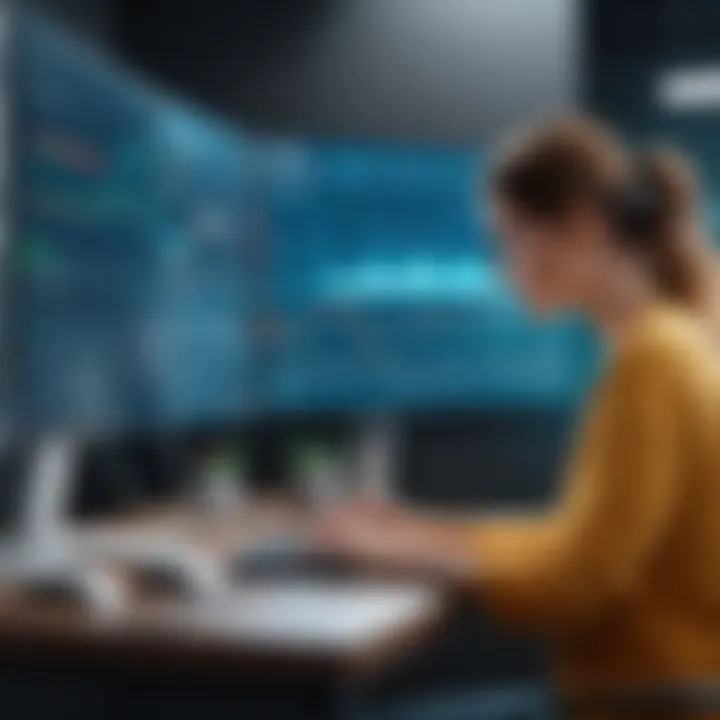
Language Variability
Language is dynamic and constantly evolving, making it difficult for sentiment analysis tools to keep up. Terms that may be trending today could lose relevance tomorrow. Additionally, dialects and regional phrases can further obscure the analysis process.
Incorporating updates into sentiment models is necessary for maintaining accuracy. This could involve regularly refreshing training datasets to encompass recent vernacular. Organizations must remain vigilant to adapt their tools to these ongoing changes.
Ultimately, addressing these challenges is essential for any organization seeking to leverage sentiment analysis effectively. By understanding ambiguity, sarcasm, and language variability, practitioners can fine-tune their approaches. This will lead to more accurate insights that are critical for successful decision-making in today’s data-driven environment.
The Future of Sentiment Analysis
The field of sentiment analysis is evolving rapidly. As businesses increasingly rely on data to make informed decisions, understanding the future of sentiment analysis becomes essential. This section explores the advancements in artificial intelligence and machine learning, potential ethical concerns, and the integration with other analytical tools. Each aspect is pivotal for shaping how organizations harness sentiment data moving forward.
Advancements in AI and
Artificial Intelligence (AI) and Machine Learning (ML) are transforming sentiment analysis drastically. Both technologies enable improved data processing speed and accuracy. For instance, AI can streamline the evaluation of vast amounts of text data from multiple sources, revealing deeper insights into public sentiment towards brands or products.
Recent advancements include sophisticated neural networks that can capture nuances in language. This is especially relevant when dealing with complex sentiments. ML algorithms are capable of learning from past data, allowing for refined sentiment classification over time. The implementation of deep learning techniques improves the system's understanding of context, enabling better handling of sarcasm and varied expressions of sentiment.
Benefits of these advancements include:
- Enhanced accuracy in sentiment classification
- Speed in processing data
- Ability to uncover hidden patterns in sentiment trends
Potential Ethical Concerns
As sentiment analysis becomes more prevalent, ethical concerns emerge. Organizations must navigate the challenges that come with collecting and interpreting data on public sentiment. The use of personal data without consent raises significant moral questions. Data privacy laws are increasingly stringent, and organizations must ensure compliance.
Bias in data sets can lead to skewed analysis results. If the training data reflects societal biases, the sentiments predicted may reinforce stereotypes. Businesses must acknowledge and mitigate these risks by refining data collection methods.
Considerations regarding ethics include:
- Ensuring transparency in data usage
- Safeguarding individual privacy
- Addressing potential biases within datasets
"Organizations must prioritize ethical considerations in their data-driven strategies to maintain trust and integrity in their operations."
Integration with Other Analytical Tools
The future of sentiment analysis lies not only in its standalone capabilities but also in how it integrates with other analytical tools. Combining sentiment analysis with business intelligence (BI) tools can provide a comprehensive view of consumer sentiment relative to market trends. For example, integrating sentiment data with financial analytics can reveal how public perception influences stock performance.
Furthermore, sentiment analysis can complement customer relationship management (CRM) systems. Using insights from sentiment analysis, organizations can tailor their communications and improve customer experiences.
Benefits of integration include:
- Enhanced decision-making through comprehensive data views
- Improved ability to adapt to consumer sentiment shifts
- Streamlined workflows for data management
In summary, the future of sentiment analysis holds promise, but it also demands careful consideration of ethical implications and the importance of effective integration with other systems. Organizations that adapt to these trends will likely gain a competitive edge in understanding and influencing public sentiment.
Ending and Implications
The conclusion and implications section serves as the capstone of this article, summarizing the essential insights gained from the discussion on Meltwater sentiment analysis. Understanding how sentiment analysis works, along with the methodologies, applications, and the challenges it faces provides a comprehensive view that is critical for businesses today. This analysis goes beyond mere data collection; it involves extracting actionable insights that can inform strategic decisions.
Meltwater sentiment analysis enables organizations to grasp public opinion and track brand perception effectively. Insights gained can steer marketing strategies, product development, and customer service improvements. A robust understanding of sentiment analysis allows companies to react promptly to market changes, enhancing their competitive edge in a dynamic landscape.
"Sentiment analysis is not just about managing impressions; it is about proactively understanding the pulse of the consumer."
As businesses face constant scrutiny and rapid shifts in consumer attitudes, the value of sentiment analysis becomes increasingly pertinent. Practitioners must consider the implications of their findings and use these insights to guide their actions.
Summation of Key Points
- Importance of Data Sources: Diverse data sources enrich sentiment analysis, offering varied insights.
- Methodologies Matter: The choice of methodologies significantly affects the quality and accuracy of sentiment analysis results.
- Challenges: Understanding the limitations and challenges of sentiment analysis is essential for accurate interpretation.
- Future Potential: The potential of sentiment analysis continues to grow with advancements in AI and machine learning.
In sum, the key points discussed emphasize the critical role Meltwater sentiment analysis plays in navigating contemporary market dynamics. Organizations that leverage these insights can achieve more informed decision-making and build stronger connections with their audience.
Recommendations for Practitioners
To effectively utilize Meltwater sentiment analysis, practitioners need to adopt several best practices:
- Invest in Training: Ensure team members are well-versed in the methodologies and tools used in sentiment analysis.
- Leverage Multiple Data Sources: Utilize data from social media, news, and customer feedback to gain comprehensive insights.
- Focus on Real-Time Analysis: Employ tools that allow for real-time sentiment analysis to stay ahead of market changes.
- Analyze Context: Understand the context behind sentiments to avoid misinterpretation of data, especially in cases of sarcasm or ambiguity.
- Integrate Findings with Strategy: Use the results from sentiment analysis to directly influence marketing strategies and product development.
By implementing these recommendations, organizations can maximize the benefits of Meltwater sentiment analysis, ultimately leading to better engagement with customers and enhanced business outcomes.