Exploring Data Analytics Services Companies
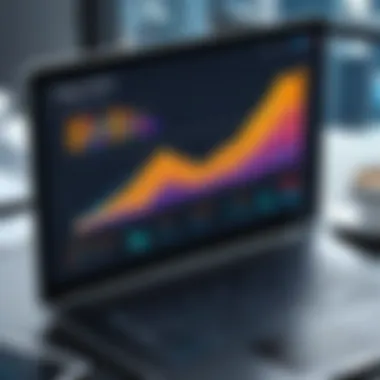
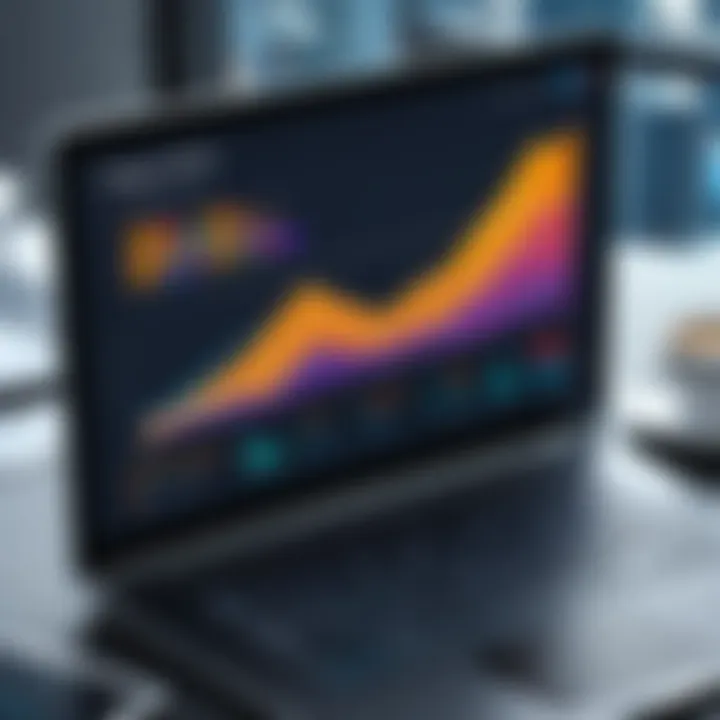
Intro
In today's data-driven landscape, understanding analytics services is vital for any organization aiming to improve its decision-making processes and optimize operational efficiency. Data analytics has established itself as a cornerstone of modern business intelligence, transforming raw data into actionable insights.
This article will elaborate on key features and capabilities of data analytics services companies, inform about their performance and reliability, and give a glimpse into the future of data analytics. Such knowledge is crucial for software developers, IT professionals, and students who are looking to deepen their understanding of this evolving field.
Features and Capabilities
Overview of Key Features
Data analytics services typically encompass a broad range of features that can address diverse organizational needs. Some of the prominent attributes include:
- Data Visualization: Tools that convert complex data sets into visual formats, enhancing comprehension and facilitating better decision-making.
- Predictive Analytics: Techniques used to forecast future trends based on historical data, enabling companies to prepare for potential outcomes.
- Real-Time Analytics: The ability to analyze data as it is collected, providing immediate insights that can influence ongoing operations.
- Self-Service Analytics: This allows non-technical users to perform analysis without deep technical knowledge, democratizing access to important data.
These features are essential for organizations that wish to remain competitive and informed in their strategic planning.
User Interface and Experience
A user-friendly interface is critical in making analytics tools accessible to a wider audience. The best platforms focus on clarity, and ease of navigation, as well as robust capabilities. An intuitive dashboard that displays key metrics and insights is pivotal. Good design enhances not just the user experience but also the overall effectiveness of data-driven decision-making processes.
On platforms like Tableau and Microsoft Power BI, for instance, users are greeted with customizable dashboards. Users can manipulate the data visually, making it easier to derive insights without needing extensive technical expertise.
Performance and Reliability
Speed and Efficiency
The speed of data processing can be the difference between seizing an opportunity and missing it. Leading analytics services companies invest heavily in technology that ensures rapid analysis of vast data sets. Solutions such as Apache Spark and Amazon Redshift are known for their speed and efficiency in handling large-scale data.
Organizations rely on performance metrics to gauge how well their analytics tools function. Faster responses lead to quicker actions, which are crucial in fast-paced business environments.
Downtime and Support
A reliable support system is fundamental for any analytics service. Companies must assess the downtime of analytics tools and the quality of customer support offered. A robust support system can significantly reduce the impact of any downtime experienced.
The ideal service will include 24/7 support with well-documented resources, including user guides and troubleshooting options. This builds a safety net for organizations venturing into complex analytical endeavors.
"Understanding the infrastructure behind analytics services is just as crucial as using the data itself. A strong foundation allows for better insights and informed decisions."
In summary, choosing the right data analytics service requires a detailed consideration of its features, performance, and reliability. This groundwork sets the stage for maximizing the value derived from analytics, ultimately enhancing business capabilities.
Preamble to Data Analytics Services
Data analytics services have emerged as a fundamental aspect of modern business operations. Organizations continuously generate vast amounts of data every day, and the need to extract actionable insights from this data is crucial. Firms that understand the significance of data analytics can enhance their decision-making processes and streamline operations. The services provided by data analytics companies help transform raw data into meaningful information, supporting strategic planning and execution.
Definition of Data Analytics
Data analytics can be defined as the process of inspecting, cleansing, transforming, and modeling data to discover useful information, draw conclusions, and support decision-making. It involves various techniques that help in understanding datasets and gaining insights. This field encompasses multiple methodologies, including statistical analysis, machine learning, and predictive modeling.
Importance in Today's Business Landscape
In today's highly competitive market, the relevance of data analytics cannot be overstated. Here are a few key reasons why data analytics is essential:
- Informed Decisions: It provides firms with the ability to make data-driven decisions rather than relying on intuition or guesswork.
- Operational Efficiency: By analyzing workflows and identifying inefficiencies, analytics can lead to improved processes, reducing costs.
- Customer Insights: Organizations gain a better understanding of their customers, allowing for tailored marketing strategies and enhanced customer experiences.
- Predictive Capabilities: Companies can forecast trends and consumer behavior, enabling proactive rather than reactive strategies.
"Organizations that leverage data analytics often outperform their peers in both revenue and efficiency."
Overall, understanding the role and definition of data analytics services is essential for businesses looking to thrive in this data-driven era. Companies that effectively utilize these services will likely hold a competitive edge in the evolving market landscape.
Types of Data Analytics Services
Understanding the various types of data analytics services is crucial for businesses aiming to leverage data for strategic advantage. Each type serves distinct purposes and offers unique insights that cater to different organizational needs. From shaping decision-making processes to guiding operational strategies, data analytics services are indispensable in today's data-driven landscape. In this section, we will delve into the four primary types of data analytics services: Descriptive Analytics, Predictive Analytics, Prescriptive Analytics, and Diagnostic Analytics. This exploration highlights their functions, benefits, and role in enhancing business intelligence.
Descriptive Analytics
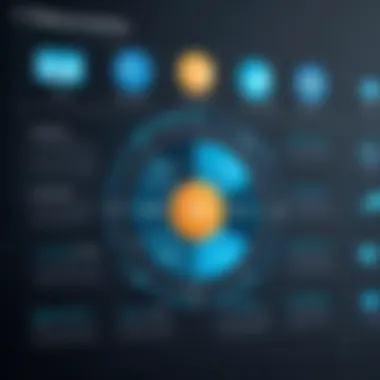
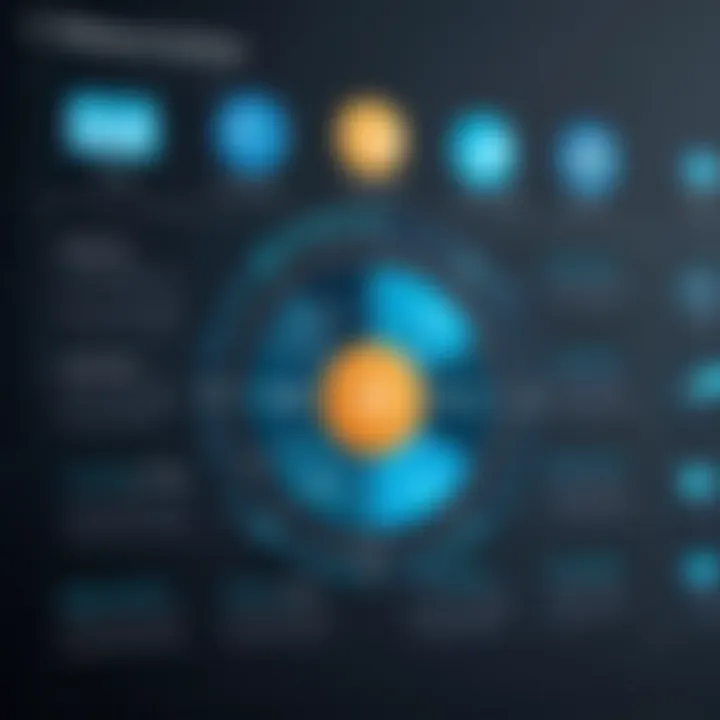
Descriptive analytics plays a key role in data interpretation by summarizing historical data to identify trends and patterns. This type of analytics provides insights into what has happened in the past. Organizations can use descriptive analytics to analyze operational performance, customer behavior, and market trends. The process incorporates data aggregation and visualization techniques which make the information easier to comprehend.
A few common applications include reporting sales figures, assessing financial performance, and evaluating customer satisfaction metrics. By understanding past performance, businesses can make informed decisions and set strategic goals accordingly. Utilizing tools such as Google Analytics or Tableau can aid in developing effective descriptive reports.
Predictive Analytics
Predictive analytics extends beyond mere observation of historical data. It involves using statistical algorithms and machine learning techniques to forecast future events. Businesses can predict customer behavior, sales trends, and potential market shifts by identifying patterns through predictive models.
This type of analytics is critical for organizations to anticipate challenges and opportunities. Effective predictive analytics relies on advanced data visualization tools and historical data processed through algorithms. The successful implementation can enable companies to remain proactive in their market approach, ensuring they are prepared for any possible outcomes.
Prescriptive Analytics
Prescriptive analytics stands as the most advanced form of data analytics. It not only predicts potential outcomes but also recommends actions to achieve desired results. This type of analytics integrates optimization and simulation techniques to provide specific recommendations.
In industries where timely decision-making is critical, such as finance or healthcare, prescriptive analytics can substantially enhance operational efficiency. Organizations can employ these insights to improve supply chain management, enhance customer experience, and optimize resource allocation. Software solutions like IBM Watson and SAS can facilitate this level of analytical capability.
Diagnostic Analytics
Diagnostic analytics answers the question "why did this happen?" By digging deeper into data, it helps to identify the causes of past performance issues. This type relates closely to both descriptive and predictive analytics and seeks to provide contextual understanding behind the trends observed.
Using statistical techniques, diagnostic analytics allows businesses to explore correlations and relationships among variables. As such, it supports organizations in troubleshooting and remedying issues that have previously occurred. Implementing tools and techniques offered by platforms such as Microsoft Power BI or Qlik can enhance diagnostic capabilities.
Key Benefits of Data Analytics Services
Data analytics services bring numerous benefits to businesses, affecting operational performance and overall strategy. Understanding these benefits is essential for organizations aiming to leverage data for competitive advantage. In this section, we will focus on three primary advantages: enhanced decision-making, improved operational efficiency, and data-driven strategy development.
Enhanced Decision-Making
One critical benefit of data analytics services is enhanced decision-making. Organizations gather vast amounts of data, but the real challenge lies in designing actionable insights from that data. Data analytics allows companies to sift through information effectively. This informed approach enables managers and executives to make evidence-based decisions rather than relying on intuition.
For example, businesses can analyze customer behaviors, market trends, and operational inefficiencies to formulate strategies. Having a clear view of data leads to more precise forecasting, risk mitigation, and resource allocation. By utilizing advanced analytics tools, businesses can identify patterns and trends that help in aligning decisions with organizational goals.
Improved Operational Efficiency
Another significant aspect of data analytics is its ability to improve operational efficiency. By analyzing workflows and processes, companies can pinpoint bottlenecks and inefficiencies in their operations.
Data analytics services also often utilize automation, which reduces manual effort and error rates. As a result, operational processes become more streamlined, allowing teams to focus on core functions. Organizations can minimize costs and boost productivity through proper analysis of performance metrics and key performance indicators.
Additionally, integration with cloud computing technologies provides real-time insights, empowering employees to respond swiftly to changes in circumstances.
Data-Driven Strategy Development
Lastly, data analytics services foster data-driven strategy development. This approach can transform the way organizations define and implement their business strategies. In a rapidly changing market, having adaptable and robust strategies is essential.
Companies employing data analytics can effectively segment their markets, understand customer preferences, and gauge competitive dynamics. This knowledge helps teams create targeted marketing campaigns, develop better products, and effectively allocate budgets. Data-driven strategies promote a culture of continuous improvement, ensuring organizations stay relevant in their respective industries.
In summary, the key benefits of data analytics services—enhanced decision-making, improved operational efficiency, and data-driven strategy development—create a solid foundation for organizations to thrive in a competitive landscape. Companies that recognize and harness these benefits will likely outperform their competitors in the long term.
"In the world of data analytics, knowledge truly is power; understanding your data is the key to succeeding in any market."
To delve deeper into the mechanisms through which organizations can harness the power of data analytics, we will explore leading companies in the field in the subsequent sections.
Leading Data Analytics Services Companies
Understanding leading data analytics services companies is critical in the evolving landscape of business intelligence. These companies not only spearhead innovation but also shape how organizations harness data to gain insights. The top players in this sector provide advanced solutions that enhance decision-making, improve operational efficiency, and crystalize strategies based on data-driven insights. By examining these key players, businesses can discern which services align with their needs to optimize performance.
Overview of Market Leaders
The realm of data analytics is populated by various robust companies that are paving the way for effective data utilization. The market leaders demonstrate strong capabilities in extracting value from data.
- IBM: Known for its comprehensive suite of analytical tools, IBM excels in serving large enterprises with its Watson Analytics platform. Businesses tap into predicting outcomes and uncovering trends.
- SAS: With its focus on advanced analytics, SAS delivers strategies that are insightful and predictive in nature. Their software options cater to various industries, enhancing their reach and effectiveness.
- Tableau: A pioneer in data visualization, Tableau empowers users to craft story-driven visualizations from complex datasets.
These players not only enhance business intelligence but also stimulate competition and innovation across the analytics market.
Specific Company Profiles
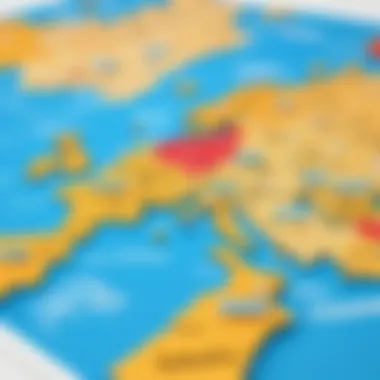
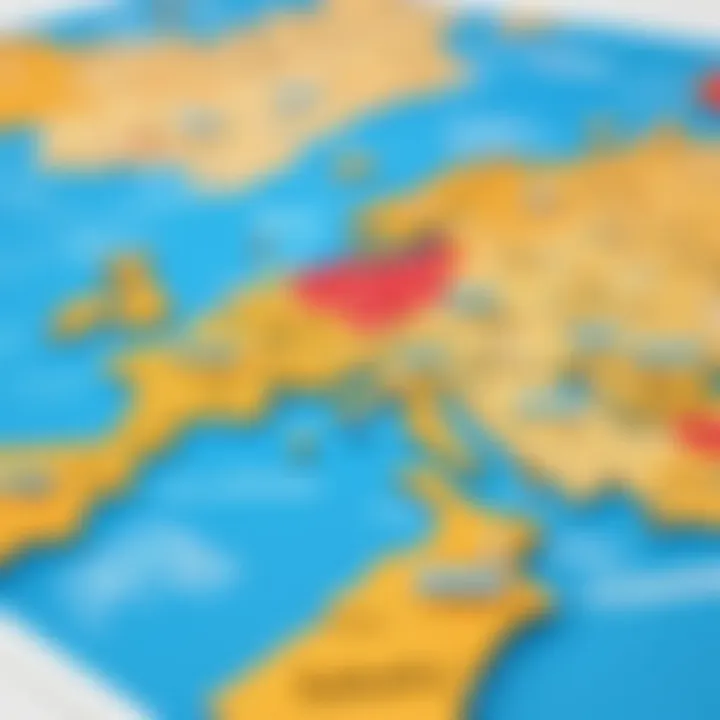
Company A
Company A specializes in cloud-based data analytics solutions, providing seamless integration with existing infrastructure. Their platform is designed to simplify data access and interpretation. A notable characteristic is their real-time analytics feature, which allows businesses to react swiftly to market changes. This makes Company A a popular choice due to its flexibility in deployment and user-centric design. Unique aspects include an intuitive interface, although there are concerns about data storage limits depending on the service tier.
Company B
Company B stands out with its focus on machine learning applications. They provide tailored analytics services that adapt to each client's unique data environment. The key feature here is the predictive modeling capabilities, which empower businesses to anticipate trends and opportunities. Their popularity stems from delivering significant ROI through optimized analytics processes. A potential downside may be the steep learning curve associated with their more complex features.
Company
Company C is notable for its commitment to data security and privacy measures. In an era of increasing data regulations, they offer robust protections, making them a trustworthy partner for sensitive industries such as healthcare and finance. Their primary selling point is the strong adherence to compliance standards and high-level encryption. However, some users report that this focus on security can result in slower data processing speeds compared to competitors.
"The blend of data analytics and strategic insight from leading companies fosters a competitive edge in the market."
Through these profiles, a clearer picture emerges of how different companies contribute uniquely to the analytics field. By assessing these organizations, professionals can make informed decisions about which services best align with their analytics goals.
Technologies Powering Data Analytics
The landscape of data analytics is significantly shaped by the technologies that drive its capabilities. Understanding these technologies is crucial for organizations aiming to leverage data for decision-making and competitive advantage. Each technology offers unique features and benefits, and together they create a robust framework for analyzing large volumes of information efficiently. This section examines the critical components, including Big Data technologies, Machine Learning and AI, and Cloud Computing, that underpin data analytics services.
Big Data Technologies
Big Data technologies are essential in managing and processing vast amounts of data generated every day. Traditional data processing tools often cannot handle such large scale data, leading to the emergence of advanced solutions like Hadoop and Apache Spark. These frameworks allow businesses to store, process, and analyze data in a decentralized manner.
Benefits of Big Data technologies include:
- Scalability: They can grow with the increasing volume of data.
- Flexibility: Diverse data types, including structured and unstructured, can be processed.
- Speed: Faster data processing helps derive insights quickly, which is vital for timely decision-making.
Companies that ignore these technologies risk falling behind competitors who utilize them to extract valuable insights from their data. As organizations strive for increased efficiency, Big Data technologies become indispensable.
Machine Learning and AI
Machine Learning and Artificial Intelligence are transforming data analytics by enabling systems to learn from data patterns and make predictions. This helps businesses adapt strategies based on insights derived from historical performances. For instance, customer behavior can be analyzed to improve user experience.
Considerations regarding Machine Learning and AI include:
- Predictive analytics: Anticipating trends and behaviors accurately.
- Automation: Reducing manual labor in data analysis tasks.
- Personalization: Tailoring services or products to individual customer preferences.
Implementing these technologies often requires expertise and a careful approach to data governance and ethics to ensure responsible use. Businesses that leverage Machine Learning and AI can achieve substantial improvements in efficiency and effectiveness.
Cloud Computing in Analytics
Cloud computing provides a flexible and cost-effective solution for data storage and processing needs. Platforms like Amazon Web Services and Microsoft Azure facilitate easy access to powerful analytics tools without the need for extensive on-premises infrastructure.
Benefits of cloud computing in data analytics encompass:
- Cost-efficiency: Lower upfront costs and pay-as-you-go models.
- Accessibility: Data can be accessed from any place, which fosters collaboration.
- Security: Enhanced security measures and consistent updates from the service providers.
As organizations increasingly adopt cloud solutions, they gain the ability to scale their analytics efforts, reducing the time taken to derive insights.
"Harnessing the right technologies not only streamlines the analytics process but also significantly enhances the value derived from data."
Challenges Faced by Data Analytics Companies
The landscape of data analytics services is vibrant yet fraught with challenges that impact both companies and their clients. Understanding these challenges is essential for both analysts and organizations that rely on data-driven insights. Two primary challenges stand out: data privacy and security issues, as well as the integration with existing systems. Addressing these obstacles is crucial for the growth and reliability of data analytics services.
Data Privacy and Security Issues
Data privacy is a major concern for organizations handling sensitive information. With the rise of data breaches and heightened regulatory scrutiny, companies must prioritize the protection of personal data. Regulations such as the General Data Protection Regulation (GDPR) impose strict requirements on how data is collected, stored, and processed. Failure to comply not only leads to hefty fines but also damages credibility.
In a data-driven ecosystem, companies collect vast amounts of information from various sources. Data analytics services must ensure confidentiality and security amidst this complexity. Employing encryption technologies is one way to protect data during transfer and storage. Moreover, organizations should conduct regular audits and vulnerability assessments to identify potential risks.
"Data protection is not just a legal obligation; it’s also a trust-building exercise between companies and customers." - Unknown
Strengthening data privacy protocols can result in increased customer loyalty. Companies that show commitment to safeguarding personal information gain a competitive edge in the market. In this era, trust is a valuable asset.
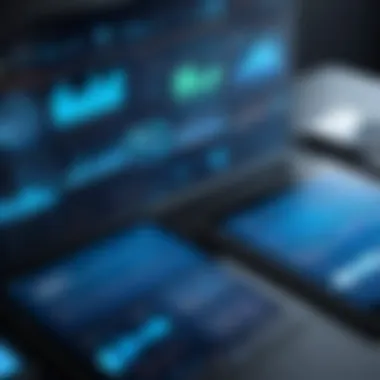
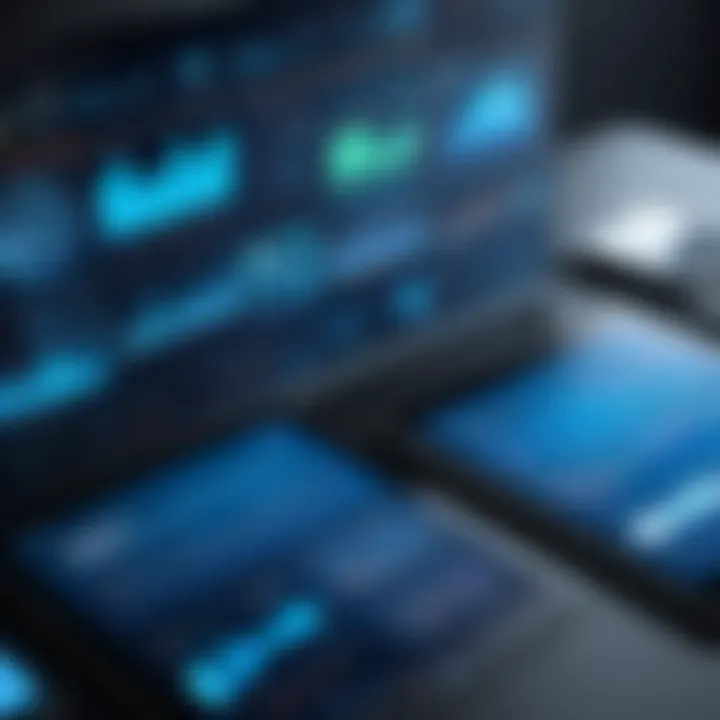
Integration with Existing Systems
Integration is another significant challenge faced by data analytics companies. Many organizations operate legacy systems that do not easily align with modern data analytics tools. This creates obstacles for data retrieval and analysis, leading to inefficient processes.
Compatibility issues hinder the effectiveness of data analytics services. A seamless integration ensures that data flows freely between systems, enabling accurate analysis and meaningful insights. Companies must invest in middleware solutions or custom APIs to bridge the gap between old and new technologies. In some cases, a complete system overhaul may be necessary to harness the full potential of data analytics tools.
Furthermore, cross-departmental collaboration is essential. Different parts of the organization may use separate data systems, leading to siloed information. Facilitating communication between teams can enhance the quality of insights generated from data analytics services.
As organizations continue to evolve, addressing these challenges is vital for the success of data analytics companies. A proactive approach to data privacy and integration can pave the way for more secure and effective analytics solutions.
Future Trends in Data Analytics Services
Understanding future trends in data analytics services is essential for organizations aiming to stay competitive in a data-driven landscape. The growth of technology is not just changing how data is analyzed but also influencing the very nature of business decisions. Companies must adapt to these trends to leverage their data effectively.
Rise of Real-Time Analytics
Real-time analytics refers to the ability to analyze data as it becomes available. This trend is crucial because it allows organizations to make decisions quickly based on the most recent data. The importance of this is evident in sectors like finance, retail, and healthcare, where timely decision-making can lead to significant advantages.
For example, in e-commerce, real-time analytics empowers companies to adjust their pricing strategy immediately based on consumer behavior.
The advantages of real-time analytics include:
- Immediate Insights: Businesses can react promptly to trends or issues, enhancing agility.
- Improved Customer Experience: Personalized experiences are possible as data about customer interactions can be analyzed instantaneously.
- Operational Efficiency: Operations can be fine-tuned in real-time, reducing waste and improving resource management.
However, implementation poses challenges such as the need for advanced technology and skilled personnel. Organizations must ensure they have the necessary infrastructure to support such analysis.
Increased Use of AI and Machine Learning
The integration of AI and machine learning into data analytics is a transformative trend. These technologies enhance the volume, variety, and velocity of data analysis. With evolving algorithms and techniques, businesses can uncover insights that are not easily recognizable through traditional methods.
The benefits of utilizing AI and machine learning in data analytics include:
- Predictive Modeling: Businesses can better forecast trends and customer behaviors, leading to more informed strategic planning.
- Automation: Routine tasks in data processing can be automated, allowing data scientists to focus on higher-level analysis.
- Enhanced Accuracy: AI algorithms can detect patterns and anomalies that human analysts might miss.
As companies look to integrate these technologies, considerations of ethical AI use and data governance become important. Striking the right balance between leveraging technology and maintaining privacy is essential for long-term success.
The End
In a world increasingly driven by data, understanding the role of data analytics services has become essential. The current business environment requires organizations to be agile and informed. This article has explored the various facets of data analytics services, including their types, benefits, challenges, and future trends. Recognizing these factors is critical for businesses aiming to harness the power of data.
Summary of Key Insights
Data analytics services offer robust solutions that can significantly enhance organizational performance. The four main types—descriptive, predictive, prescriptive, and diagnostic analytics—serve distinct purposes that help businesses make informed decisions. Key benefits include improved operational efficiency, enhanced decision-making processes, and the facilitation of data-driven strategies. The leading companies in this space, such as IBM and SAS, demonstrate the power of analytics in transforming data into actionable insights.
"Data analytics is not just about collecting data; it is about translating that data into meaningful strategies that drive success."
Furthermore, future trends indicate a shift towards real-time analytics and a growing reliance on AI and machine learning, which can refine and accelerate insights for businesses. It is evident that companies that prioritize data analytics services are better equipped to adapt and thrive in their markets.
The Path Forward for Businesses
As organizations look forward, the integration of data analytics into their operational and strategic frameworks will be paramount. Businesses should start by assessing their data needs and existing processes, setting clear goals for what they aim to achieve with analytics.
Investing in training and upskilling employees to handle analytics tools effectively is crucial. Also, collaborating with established data analytics firms can provide the necessary expertise and technology solutions. By leveraging analytics services, companies can not only navigate current challenges but also identify growth opportunities. This path requires commitment and a willingness to embrace change, as data-driven decision-making will undoubtedly play a pivotal role in the future of sustainable business practices.
Why References Matter
References serve multiple important functions in this article. They help in:
- Establishing Credibility: Readers tend to trust information that is backed by reputable sources. Citing relevant materials demonstrates thorough research.
- Providing Additional Resources: Interested readers can pursue deeper knowledge through the cited works. Links to studies or articles can offer further context or nuanced understanding on specific topics.
- Supporting Claims: In a field as dynamic as data analytics, assertions need solid backing. References justify the claims made, enabling a logical flow of ideas.
Key Elements of Effective References
- Reputable Sources: Only include references from well-known and respected publications or organizations to ensure your content is credible.
- Diverse Perspectives: Include various types of references, such as articles, white papers, webinars, and reviews. This variety creates a holistic view of the topic.
- Updated Information: Data analytics is rapidly evolving. Ensure that references reflect the most current trends and technologies in the field.
Considerations When Using References
- Citation Style: Maintain a consistent citation style throughout the article. This consistency enhances readability.
- Avoid Overloading: While references are important, too many can overwhelm the reader. Focus on quality over quantity.
- Relevance: Ensure each reference directly supports the content discussed. Irrelevant citations dilute the overall quality of the writing.
"References not only validate the information presented but also enrich the reader’s understanding of the subject matter."
By following these guidelines, references can significantly enhance the article's impact. The integration of these key points allows for a deeper engagement with the material, positioning the article as a valuable resource for professionals and students alike in the field of data analytics.