Understanding Operations Analytics for Business Success
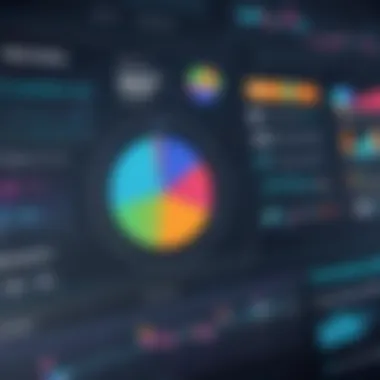
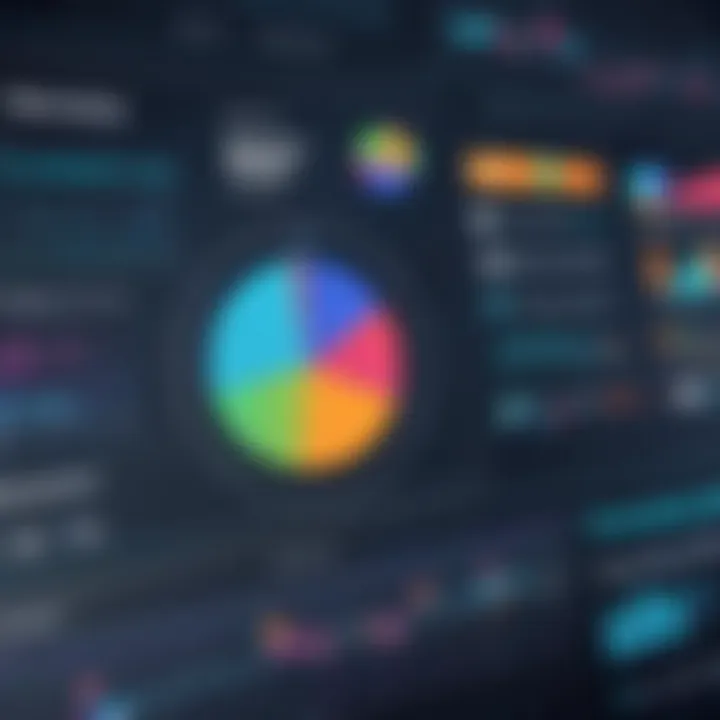
Intro
In todayās rapidly changing business landscape, the ability to glean actionable insights from data is crucial. This is where operations analytics comes into play. By harnessing data analytics, businesses can not only enhance operational efficiency but also make informed decisions that drive growth and innovation. Through the integration of various methodologies and tools, operations analytics provides a framework for organizations to refine their processes and optimize performance.
As organizations increasingly turn to data for their strategic initiatives, the need for a thorough understanding of operations analytics becomes increasingly important. Let's dive deeper into the key aspects of this essential topic.
Features and Capabilities
Overview of Key Features
Operations analytics is defined by various features that set it apart from traditional analytical approaches. Some of these key elements include:
- Real-time data processing: The ability to analyze data as itās generated facilitates timely decision-making.
- Predictive analytics: Forecasting future trends based on historical data helps businesses prepare strategically.
- Visualization tools: Graphical representations of data make it easier to identify patterns and insights.
- Integration capabilities: Seamless integration with existing tools and platforms enhances workflow efficiency.
These features enable companies to leverage vast amounts of data to drive organizational success.
User Interface and Experience
The user interface and experience (UI/UX) of operations analytics tools play a pivotal role in user adoption. A well-designed interface allows users to engage with complex data intuitively. Important aspects to consider include:
- Ease of navigation: Users should find it simple to access features without extensive training.
- Customization options: Tailoring dashboards and reports to meet specific needs is essential for user satisfaction.
- Mobile access: As businesses operate in a more flexible environment, access from various devices increases operational efficiency.
A focus on user experience ensures that insights can be quickly communicated, helping teams make informed decisions promptly.
Performance and Reliability
Speed and Efficiency
In the realm of operations analytics, performance metrics are a significant focus. Businesses require tools that perform efficiently under pressure. The ideal analytics solution must be:
- Fast in processing data: Minimizing lag time allows for real-time insights narrow.
- Scalable: As organizations grow, their analytics tools should accommodate increasing data volumes without degradation.
Ensuring speed and efficiency not only improves decision-making but also enhances productivity across the board.
Downtime and Support
Reliable support and minimal downtime are critical factors in maintaining operational continuity. When selecting an operations analytics tool, consider:
- Service level agreements (SLAs): Understand how support is structured, and what guarantees are provided.
- Community support: Platforms with strong user communities can offer additional resources and problem-solving avenues.
A robust support system and minimal service disruptions lead to greater confidence among users and stakeholders.
"Data is the new oil. Itās valuable, but if unrefined, it cannot really be used."
Understanding that operations analytics is not just about using tools, but about adopting a mindset of continuous improvement, is crucial. Only then can organizations truly leverage the power of their data for competitive advantage.
Defining Operations Analytics
Operational analytics stands as a vital aspect within the sphere of modern business practices. In todayās fast-paced world, the ability to make informed decisions based on thorough analysis of operational data can set companies ahead of their competition. Understanding what operations analytics entails lays the groundwork for grasping how it can be harnessed effectively across various industries.
The Concept and Scope
Operations analytics can be broadly defined as the practice of collecting, processing, and analyzing data related to various operational processes to optimize efficiency and effectiveness. It involves not just mere calculations but also interpreting data to uncover valuable insights that drive informed decisions.
At its core, this field encompasses several dimensions:
- Data-Driven Approach: Operations analytics emphasizes employing real-time data to improve functioning. Companies become more agile and capable of adapting to changes when they utilize facts over intuition.
- Cross-Functional Insight: By examining operational data across multiple departmentsālike supply chain, production, and customer serviceābusinesses can identify areas that benefit from improvement. Itās like taking a holistic view of an organizationās heartbeat to find anomalies that might affect overall health.
- Iterative Learning: This process doesnāt end after data is gathered and analyzed. The learnings feed back into operations, leading to continuous improvement, much like tuning a musical instrument where slight adjustments yield a harmonious outcome.
The scope of operations analytics is vast, stretching from industries such as manufacturing to services, healthcare to technology. Businesses are leveraging it to manage performance, streamline processes, and ultimately enhance customer satisfaction.
Distinction from Other Analytical Methods
While various analytical methods exist, operations analytics possesses a unique focus that sets it apart. It's essential to understand this distinction to appreciate its true value.
- Focus on Operational Data: Unlike other analytics forms, which may dwell on financial or market data primarily, operations analytics zooms in on the intricate details of operational workflows. This method looks at daily processes, staff productivity, and resource allocation, ensuring organizations work efficiently.
- Predictive and Prescriptive Nature: A hallmark of operations analytics lies in its predictive capabilities, allowing businesses not only to analyze what has happened but also to give insights into what is likely to occur. It can suggest actions based on data trends, marking a step beyond merely descriptive analytics.
- Integration with Operational Systems: Operations analytics integrates seamlessly into existing systems, providing insights in real-time. It differs from standalone analytical techniques that require data extraction and separate analysis.
"The modern age of operations analytics constitutes a bridge between raw data and actionable insight, providing the roadmap for enhanced operational strategies."
Ultimately, distinguishing operations analytics from other methodologies iterates its specialized role in enhancing internal processes, which is critical for long-term business sustainability and competitiveness. As organizations navigate the ebbs and flows of their environments, a solid grasp on operations analytics grants them not just a compass but a detailed map.
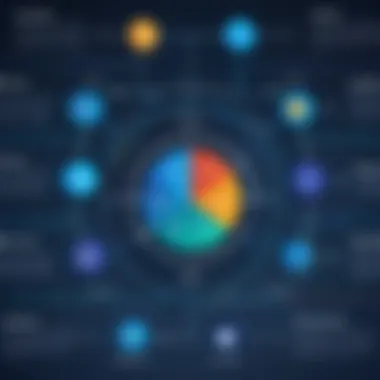
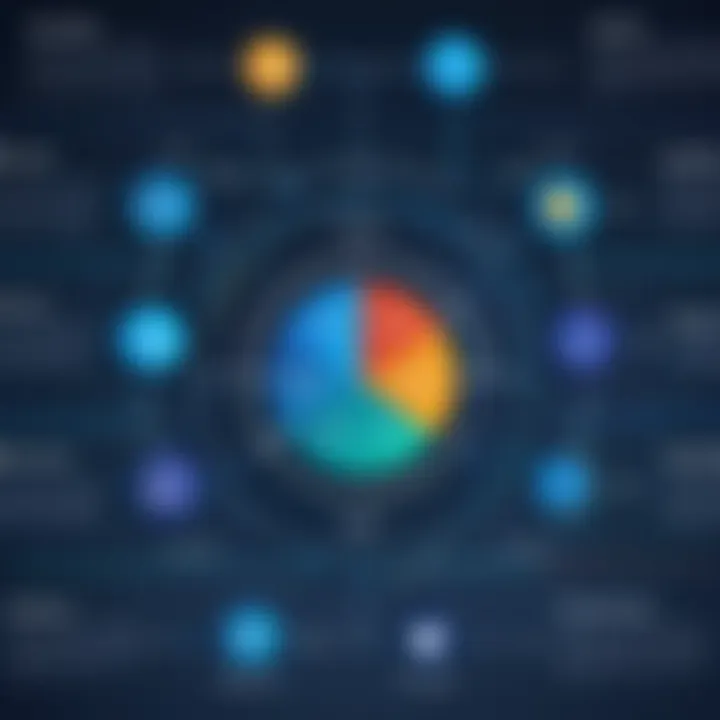
Historical Context
Understanding the historical context of operations analytics is akin to looking through a rearview mirror; it reflects the evolution and significant shifts that have shaped the practices we see today. Historical analysis provides key insights into how various methods and technologies developed, offering a lens for us to better appreciate current innovations and challenges.
Evolution of Analytical Practices
Operations analytics has not always held the prominent place it does today. In the early days, businesses relied largely on intuition and experience-driven decision-making. It wasn't until the late twentieth century that organizations began incorporating systematic data analysis into their operations. Initially, this involved basic statistical methods and spreadsheets to process data.
As industries faced increased competition and complexity, the demand for more sophisticated analysis techniques grew.
- Shift from Qualitative to Quantitative: The transition from qualitative insightsāthink gut feelingsāto quantitative data marked a pivotal change. Businesses began to realize that numbers could tell powerful stories.
- Advent of Advanced Statistical Models: As statistical methods evolved, so too did the tools. Techniques such as regression analysis and optimization algorithms became commonplace, allowing for more accurate forecasting and modeling of operations.
- Integration of Internet Technologies: The rise of the internet introduced new possibilities for data collection and analysis. Organizations could tap into a vast pool of information, opening doors to enhanced decision-making capabilities.
This evolution was not simply about adopting new tools; it represented a cultural shift in how companies approached business challenges. Many began creating dedicated analytics teams, which ultimately laid the groundwork for more complex operations analytics we see now.
The Role of Technology in Advancements
It's no surprise that technology serves as the backbone of operations analytics. The historical advancements in computational technologies have played a vital role in transforming data into actionable insights.
- From Mainframe to Cloud: Initially, businesses relied on robust mainframe computers, limited by the hardwareās capabilities. Fast-forward to the present, where cloud computing allows for scalability and access to powerful computing resources without significant upfront investment.
- Big Data and Storage Solutions: The introduction of Big Data technologies allows organizations to manage and analyze vast datasets efficiently. Companies now harness the power of data lakes and warehouses, enabling them to store various types of data, generating insightful analytics.
- Tools and Software Evolution: Tools like Tableau, Power BI, and various specialized analytics software have emerged, reflecting a trend towards user-friendly interfaces that democratize data access. This democratization allows not just data scientists but also operational managers to derive insights effectively.
- Machine Learning and AI: In the past decade, artificial intelligence and machine learning have added another layer of sophistication to operations analytics. Algorithms can now sift through data, learn patterns, and predict future trends with bewildering accuracy. The ability to process vast amounts of information quickly has led to efficiencies that were once thought impossible.
"Technology has redefined how we perceive analytics. From simple statistical models to AI-driven solutions, the journey has created a diverse landscape of opportunities."
To sum up, the evolution of analytical practices and the integral role of technology in advancements not only underscore the rich history of operations analytics but also paint a vivid picture of its potential future. Understanding where it all started is crucial for appreciating the complex landscape we navigate today.
Key Components of Operations Analytics
In the realm of operations analytics, certain pillars serve as the foundation upon which effective strategies can be built. Understanding these key components is essential for businesses aiming to harness the power of data to improve efficiency and decision-making. These components include data collection methods, analytical techniques, and the tools used for reporting and visualization. Each aspect plays a vital role in ensuring that organizations can derive actionable insights from raw data.
Data Collection Methods
Collecting data is akin to gathering raw materials before crafting a masterpiece. Different methodologies exist to capture the right data, and the choice depends on the goals of the organization. Organizations often employ various sources for data collection:
- Surveys and Questionnaires: These are traditional yet effective methods for obtaining qualitative data directly from stakeholders. Understanding customer satisfaction or employee engagement can greatly influence strategies.
- Enterprise Resource Planning (ERP) Systems: Modern businesses often utilize ERPs to integrate various business processes. These systems automatically capture operational data across departments, offering a comprehensive view.
- Internet of Things (IoT) Devices: With technology evolving at breakneck speed, IoT devices have become ubiquitous. They collect real-time data from devicesāthink smart sensors in production lines that track performance and efficiency metrics.
- APIs (Application Programming Interfaces): APIs enable seamless data flow between different applications. This is crucial for aggregating data from multiple software solutions, thereby enriching the analysis.
Utilizing a mix of these methods allows organizations to build a robust dataset that accurately reflects their operational landscape, enabling them to respond tactically and strategically.
Analytical Techniques Used
Once the data is in hand, a business must sift through it to strike gold. The analytical techniques employed can significantly dictate the quality of insights derived. Here are some common practices:
- Descriptive Analytics: This technique explains what has happened in the past. By identifying trends and patterns in historical data, businesses can make educated guesses about future events.
- Predictive Analytics: Leveraging statistical algorithms and machine learning techniques, predictive analytics helps in forecasting future outcomes based on historical data. This technique can be particularly invaluable in sectors such as retail where demand forecasting is crucial.
- Prescriptive Analytics: This goes a step further, suggesting courses of action based on the outcome of predictive analytics. By using optimization algorithms, businesses can evaluate scenarios to find the most effective solutions.
Incorporating these techniques allows businesses not just to react to situations but to proactively shape their strategies. Analytical techniques turn mere numbers into actionable intelligence.
Reporting and Visualization Tools
Finally, presenting analytical findings in an understandable and visually appealing manner cannot be overstated. Reporting and visualization tools transform complex data sets into digestible insights. Common tools include:
- Tableau: This powerful visualization tool helps organizations create interactive, shareable dashboards that depict trends and insights.
- Power BI: Developed by Microsoft, this tool provides an interface for transforming raw data into informative visuals. Like a painter with a canvas, businesses utilize it to depict their stories.
- Google Data Studio: For those looking for a more straightforward approach, Googleās free offering allows users to create reports pulling information from different sources, making it an accessible option for many.
Through effective visualization, businesses not only make sense of their data but also communicate their findings to stakeholders effectively.
"In the world of business, data is not just king; itās the entire estate. To leverage that estate, one must know how to collect, analyze, and represent data efficiently."
All in all, the key components of operations analytics serve as critical building blocks in navigating the complexities of modern business landscapes. Each element plays its role, and their interaction enhances a businessās capacity to devise informed strategies that lead to sustainable growth.
Applications in Various Industries
Operations analytics has become an indispensable part of numerous industries, driving efficiency, enhancing productivity, and boosting competitive advantage. The application of this analytical discipline varies by industry, but the core benefits often alignāimproving operational workflows, making informed decisions, and deriving actionable insights from data. Understanding how operations analytics can specifically benefit diverse sectors helps to contextualize its importance and showcases its transformative potential across the board.
Manufacturing Sector
Manufacturing is a prime example where operations analytics shines. In this sector, companies often contend with complex supply chains, fluctuating demand, and the need to maintain quality while optimizing production costs. By leveraging operations analytics, manufacturers can gain insights into production processes and understand resource allocation better.
For instance, real-time data can identify bottlenecks in production lines, allowing for immediate adjustments to improve throughput. Additionally, predictive analytics can forecast equipment failures, enabling maintenance to be performed proactively rather than reactively, thus minimizing downtime. In this case, the integration of Internet of Things (IoT) devices allows for better monitoring and feeding of valuable data into analytical models.
"In manufacturing, success isnāt just about producing more; itās about producing smarter."
Healthcare Optimization
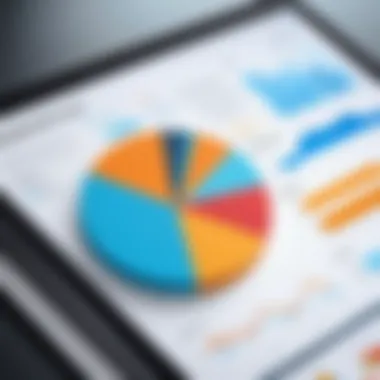
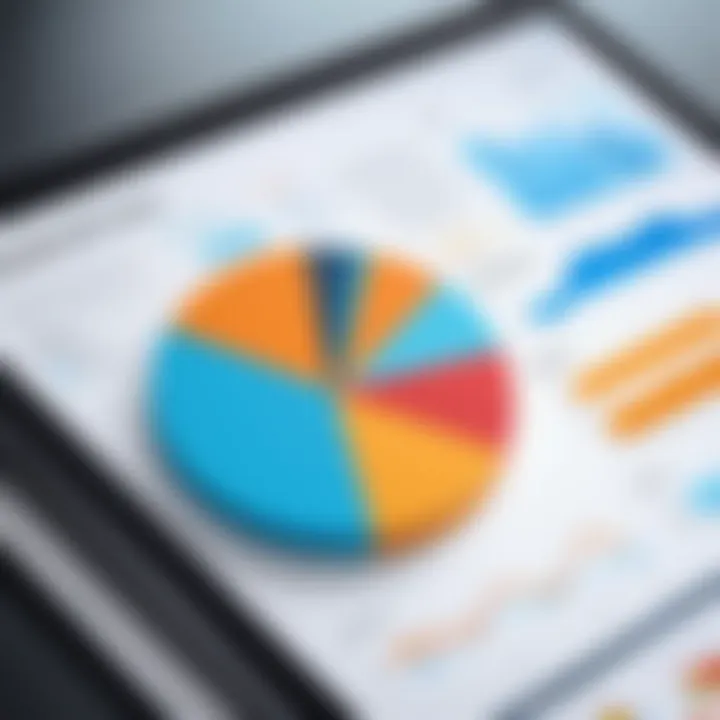
The healthcare industry stands to benefit immensely from operations analytics, offering opportunities to enhance patient care while controlling costs. Hospitals and healthcare providers can use analytics to manage patient flow in emergency rooms, optimize staffing levels, and improve patient outcomes by identifying trends in treatment efficacy.
For example, by analyzing patient records and crowdsourced data, hospitals can predict surges in patient visits, allowing for resource allocation to meet demand more effectively. Furthermore, analytics can help with better inventory management, ensuring that critical supplies are available when needed without overstocking. Such insights pave the way for a more organized and responsive healthcare environment.
Supply Chain and Logistics
In supply chain and logistics, operations analytics significantly impacts efficiency and cost management. Companies engaged in logistics can analyze transportation routes, reducing delays and fuel costs through smarter routing strategies. By harnessing data from every aspect of the supply chain, organizations can identify inefficiencies, streamline processes, and respond quicker to changes in demand.
For instance, using analytics can help companies optimize warehouse space by analyzing product turnover rates, thereby improving storage solutions and reducing costs. Moreover, predictive analytics aids firms in anticipating disruptions, whether due to market changes or weather conditions, allowing for timely adjustments to maintain service levels.
Benefits of Implementing Operations Analytics
Implementing operations analytics is not just another trend that companies decide to follow; it's a crucial aspect of modern business strategy. The benefits are manifold, making it well worth the time to understand what they encompass and how they can be maximized for value. Whether one is involved in manufacturing, logistics, or customer-oriented sectors, the push for integrating analytics into daily operations proves rewarding. Diving into specific benefits can help illustrate this point strait into professionalsā minds.
Enhanced Decision Making
Enhanced decision making is quite possibly the cornerstone of all the benefits drawn from operations analytics. When data enters the scene, decisions are no longer based merely on hunches or outdated information.
By utilizing analytics, a clearer picture emerges from the raw numbers. For instance, a large retailer can examine purchase trends, seasonal spikes in sales, and inventory turnover rates, leading to more informed decisions regarding stock levels. A manager who can anticipate which products will fly off the shelves during the holiday season is using data to steer the ship, rather than hoping for smooth waters.
"Data is like the air we breathe; it surrounds us and we cannot see it, but it is crucial for any organization."
The methodology of predictive analytics enables leaders to not only look at what's happened but also forecast what might happen next. Integrating machine learning tools lets businesses mine historical data and identify patterns, which are vital for proactive decision-making. Immediate concerns can be addressed before they become full-blown crises.
Increased Operational Efficiency
When we discuss increased operational efficiency, we tap into the heart of what analytics can achieve across various industries. This isn't just about speeding up processesāit's about refining them.
Let's consider a logistics company that tracks delivery times and routes in real time. By analyzing data on traffic patterns, weather conditions, and order volumes, the company can optimize routes and reduce delivery times significantly. Think about it; less time on the road means lower fuel costs, happier customers, and a better bottom line.
- Enhanced scheduling
- Reduced waste
- Streamlined workflows
These are just a few areas where operational efficiency blossoms as a direct result of analytics. In the world of manufacturing, utilizing data for machine utilization and maintenance predictions can keep equipment running longer and reduce costly downtimes. All these efficiencies can lead to a significant competitive advantage.
Improved Customer Insights
Improving customer insights leads to a deeper understanding of consumer behavior. We live in times where personalization attracts customers more than generic marketing ever could. Operations analytics plays a major role in achieving this deeper understanding.
By analyzing data from various touchpointsāsuch as online purchases, customer feedback, and social media interactionsācompanies can develop better products and tailor marketing campaigns in a way that resonates with their audience. For example, an online clothing retailer can assess which items are most frequently returned or which colors are flying off the shelves.
- Understanding preferences: Know what customers like and don't like.
- Anticipating needs: Predict what consumers will want before they do.
- Optimizing communications: Fine-tune messaging based on distinct customer segments.
This level of insight paves the way for not only retaining existing customers but also brings new ones on board, leading to increased revenue and brand loyalty.
In summary, the benefits of implementing operations analytics extend from boosting decision-making capabilities to achieving operational efficiency and enhancing customer insights. By tapping into the flow of real-time data, businesses position themselves ahead of competitors, ensuring they remain responsive and relevant in an ever-evolving market.
Challenges in Operations Analytics
Navigating the world of operations analytics is no walk in the park. The benefits it offers are impressive, but potential pitfalls exist, making the understanding of challenges crucial in any analysis. By exploring these hurdles, organizations can position themselves to capitalize on the advantages while minimizing risks. Letās break this down into three core challenges that affect the implementation and effectiveness of operations analytics in various industries.
Data Quality and Integrity Issues
Data is the lifeblood of operations analytics, but without quality data, the insights drawn can lead to poor decisions. Imagine a scenario where a retail company bases its inventory decisions on outdated sales figures. The result? Overstock of products that aren't selling, leading to unnecessary costs.
To improve data quality, businesses need to establish strict protocols for data entry, undergo regular audits, and employ cleansing techniques. This includes validating your sources, making sure the data is current, and ensuring it's relevant. Data governance should be at the forefront of operations analytics strategies. In practicing sound data management, organizations can mitigate risks and harness powerful insights.
A well-executed data integrity strategy can turn potential pitfalls into stepping stones for success.
Integration with Existing Systems
Another sticky wicket is the integration of operations analytics with existing systems. This can be compared to trying to fit a square peg into a round hole. Many companies may operate with legacy systems that just donāt gel with modern analytics tools.
To avoid the chaos of incompatible systems, it's vital to conduct a thorough assessment of current infrastructure before rolling out new tools. Investments in middleware or other integration solutions can pay dividends in the long run. Seamless integration ensures a smooth flow of information, enabling real-time data analysis. In this way, organizations can fast-track their decision-making processes, gaining an edge in a highly competitive market.
Skilled Workforce Requirements
Finally, the need for a skilled workforce cannot be overlooked. The tools might be advanced, but they require a human touch. A mere algorithm can't replace the intuition and creativity that comes from seasoned professionals.
Organizations face the challenge of finding talent who can not only understand the intricacies of data analysis but also possess domain expertise in their respective industries. As such, investing in training and development becomes essential. Programs that upskill current employees or attract talent can bolster an organization's analytical capabilities. Additionally, fostering a culture of continuous learning and knowledge-sharing can reinforce the skills needed for successful operations analytics.
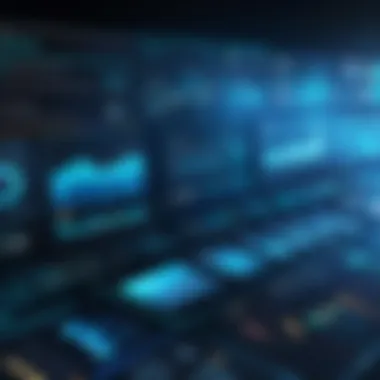
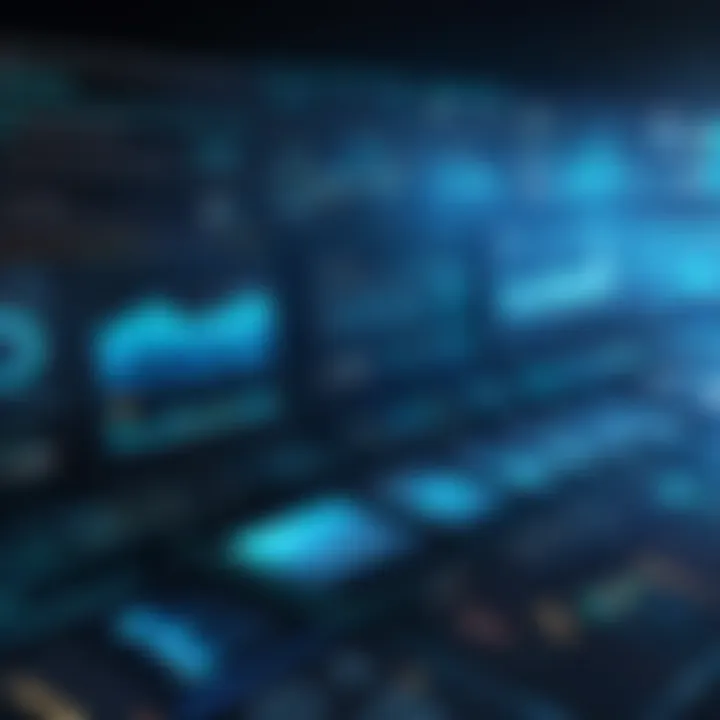
By tackling these challenges head-on, organizations can turn obstacles into opportunities, setting a solid foundation for leveraging operations analytics effectively.
Future Trends in Operations Analytics
As we look ahead, the landscape of operations analytics is continuously shaping itself with emerging technologies and methodologies. Grasping these upcoming trends isnāt just prudent; it's essential for any professional to keep their edge in a competitive environment. Operations analytics is poised to enhance decision-making processes and optimize operational efficiencies at an unprecedented pace, making it imperative to understand some key developments that are likely to take center stage in the near future.
Artificial Intelligence and Machine Learning
Artificial Intelligence (AI) and Machine Learning (ML) are not just buzzwords anymore; they are rapidly transforming operations analytics into something more dynamic and predictive. The integration of AI can automate routine tasks, freeing professionals to focus on strategic decision-making. Moreover, ML algorithms are capable of analyzing vast data sets at speeds impossible for humans, identifying patterns and trends that can forecast outcomes.
For instance, consider a manufacturing firm that implements predictive maintenance. Using AI, the system can analyze machinery data in real-time and predict failures before they happen. This not only saves costs on repairs but also minimizes downtime. The benefits of these technologies also ripple out to other sectors. In healthcare, ML can analyze patient data to optimize resource allocation, while retail can leverage it for personalized customer experiences.
"With AI and ML, the future of operations analytics is not only about data but about understanding it in meaningful ways that directly impact business outcomes."
Real-Time Data Analytics
The ability to analyze data in real-time is transforming how organizations operate. In the past, decisions were often based on historical data, which could result in missed opportunities. Now, with the advent of real-time data analytics, businesses can monitor operations as they happen, adjusting strategies swiftly based on current trends and variables.
This immediate responsiveness is particularly crucial in sectors like logistics, where delays can be costly. For instance, a shipping company using real-time analytics can reroute shipments on-the-fly based on traffic conditions or weather alerts, optimizing delivery times. In ecommerce, real-time inventory analytics can signal stock shortages, allowing companies to replenish stock before running out. This adaptability not only enhances customer satisfaction but also cultivates a proactive business culture.
Cloud-Based Solutions
Cloud computing has revolutionized how organizations handle data and analytics. It opens up opportunities for scalable solutions that can adapt as business needs evolve. Utilizing cloud-based platforms, companies can store and process vast amounts of data without the hefty investment of on-premises infrastructure.
Cloud-based solutions offer flexibility, enabling collaboration across departments and geographical locations. Teams can access real-time data from anywhere, fostering a culture of data-driven decision-making. Moreover, many cloud services come equipped with advanced analytics and AI capabilities, allowing organizations to implement sophisticated analytics without a steep learning curve. This becomes a game-changer for smaller organizations that may not have the resources to invest in extensive IT systems.
Operationalizing data used to mean significant capital layoffs; now, companies can start small and scale up based on their growing needs and insights. In this new world, organizations that harness the power of cloud solutions will be primed to leap ahead of their competitors.
Case Studies of Successful Implementation
Exploring case studies of successful implementation in operations analytics is vital as it provides practical insights, showing how theoretical concepts translate into real-world applications. These examples shed light on the specific elements that fuel success, highlighting the techniques, tools, and methodologies that lead to tangible results. Within these narratives, practitioners and scholars can glean valuable lessons on best practices, common pitfalls to avoid, and the transformative potential of operations analytics. Ultimately, these case studies offer a richer understanding of the benefits derived from strategic data utilization in diverse industries.
Case Study in Retail
In the retail sector, a prominent company utilized operations analytics to revamp its supply chain processes. The company, let's say itās a popular clothing retailer, faced challenges related to inventory management. Their traditional methods often left them with either surplus stock or severe shortages, both of which hurt profitability and customer satisfaction.
To address this, they implemented an analytics platform that integrated data from various sources, including sales records, customer feedback, and even social media trends. They began by examining historical sales patterns and correlating them with external factors like seasonal changes and promotional campaigns. Using predictive analytics, they could project future demand with greater accuracy.
As a result, the clothing retailer not only reduced excess inventory costs by 20% but also improved stock availability, enhancing customer experience. This case illustrates how data-driven insights can lead to smarter decision-making and operational efficiencies, confirming that operations analytics can be a game-changer in the competitive retail landscape.
Case Study in Manufacturing
Consider a well-known automotive manufacturer that faced production delays due to equipment malfunctions and inefficient scheduling. By leveraging operations analytics, they conducted a deep dive into their manufacturing process. They implemented a real-time data analytics system that monitored equipment performance and maintenance needs.
By gathering and analyzing data on machinery usage and failure rates, the company identified bottlenecks in production and specific machines that required preventive maintenance. The outcomes were significant. They managed to reduce downtime by 30%, increase productivity by 25%, and ultimately boost their overall output without a corresponding increase in labor or operational costs.
This case underlines how integrating analytics into manufacturing processes can lead to optimized operations, reaffirming the critical role of data in maintaining competitive advantage and operational effectiveness in todayās market.
Case Study in Logistics
In the logistics arena, a major global shipping company embarked on an analytics initiative to improve their route planning. This involved analyzing historical shipping data alongside real-time traffic and weather conditions. The company used advanced algorithms to model various shipping routes and their associated costs, factoring in fuel efficiency, travel time, and other logistical considerations such as customer deliverability requirements.
The outcome was impressive: the shipping firm reduced fuel consumption by 15%, minimized delivery times, and enhanced overall customer satisfaction. By optimizing routes and schedules through predictive analytics, the company proved how operations analytics could transform logistical operations and increase service efficiency.
Successful implementation of operations analytics not only provides cost savings but also strengthens customer relationships and fosters brand loyalty.
In summary, these case studies in retail, manufacturing, and logistics all demonstrate the potential of operations analytics to drive efficiency and innovation. Each example reflects distinct challenges and solutions yet points to a common truth: harnessing data strategically can lead to significant advancements in operational performance.
Culmination
In wrapping up our exploration of operations analytics, it is crucial to understand not just what weāve covered, but also why it matters. The insights offered throughout this article signify the larger role that data plays in driving and shaping modern business strategies. As weāve discussed, the effective use of operations analytics leads to more informed decision-making, resulting in tangible benefits such as enhanced efficiency and customer satisfaction.
Summary of Key Insights
There are a few takeaways from our discussion that stand out:
- Data-Driven Culture: Emphasizing a culture rooted in data collection and analysis empowers organizations, enabling them to adapt and respond more quickly to changing market conditions.
- Interdisciplinary Nature: Operations analytics intersects with various fields, from artificial intelligence to supply chain management, highlighting its versatility and relevance across different sectors.
- Real-World Application: Through case studies in retail, manufacturing, and logistics, we see direct examples of how analytics can lead to operational improvements, providing a roadmap for other sectors to follow.
In essence, organizations that invest time and resources into understanding and implementing operations analytics position themselves at a competitive advantage in todayās data-centric landscape.
Final Thoughts on Future Directions
Looking ahead, itās evident that operations analytics isnāt just a passing trend. The integration of technologies such as artificial intelligence and real-time data processing is set to revolutionize how businesses operate. Here's what to keep an eye on:
- Advancements in AI: Continued improvements in AI will enable deeper insights from data, allowing organizations to forecast trends and anticipate customer needs more accurately.
- Cloud Computing Growth: With the increased reliance on cloud solutions, businesses can expect more flexible and scalable analytics tools that allow for collaboration and integration across teams.
- Emphasis on Real-Time Analytics: As data generation accelerates, the ability to analyze data in real-time will become even more critical for operational responsiveness and strategic adjustments.
In closing, the journey of operations analytics is just beginning. For businesses aiming to thrive, understanding and harnessing this powerful tool is no longer optional, but a requisite for ongoing success.