Sift Fraud Detection: Insights and Implications
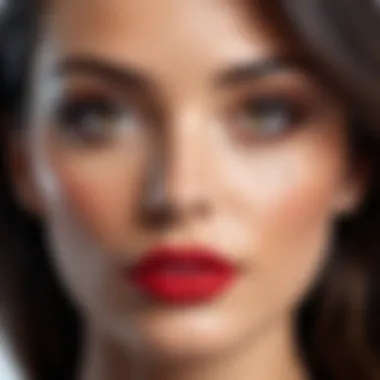
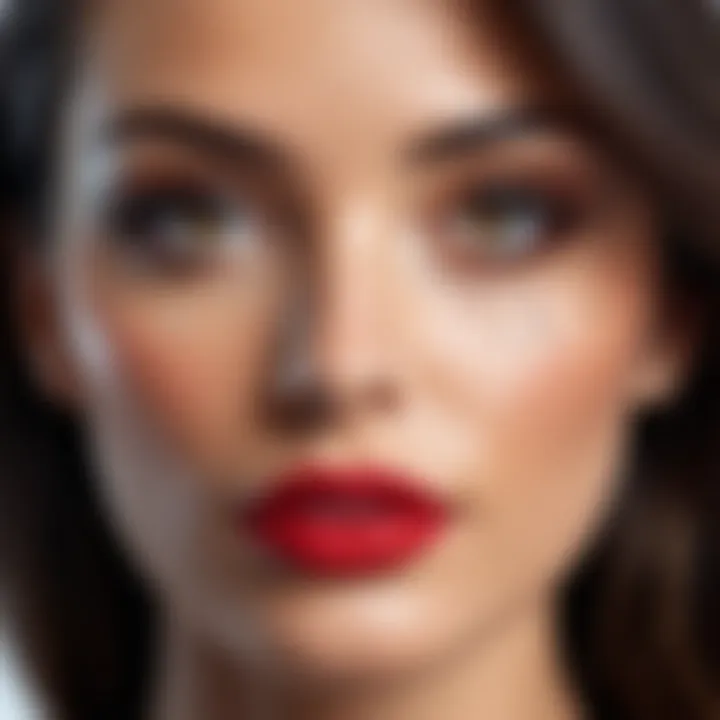
Intro
In todayâs digital era, where transactions happen at the speed of light, the stakes in fraud detection have never been higher. With a growing dependence on online services, the escalating rate of cyber threats is a concern that can't be overlooked. Fraud detection plays a pivotal role in protecting sensitive information, preventing financial loss, and ensuring overall trust within the landscape. In this context, Sift has emerged as a beacon, illuminating the path to better fraud prevention methods.
At its core, Sift combines the prowess of advanced technology with the analytical skills of machine learning to offer solutions that stand out in an increasingly crowded market. Itâs crucial to understand what makes Sift's approach distinct and relevant to businesses today. With a focus on nuances and underlying mechanisms, this article endeavors to shed light on Siftâs effectiveness in thwarting fraud while fostering a secure environment for consumers and businesses alike. Let's jump into the features and capabilities of this innovative tool.
Intro to Fraud Detection
Fraud detection is more than just a necessary component of business; itâs a vital pillar that upholds the integrity of any organization. With the rise of digital transactions and online interactions, the stakes have never been higher. Businesses face threats from different corners, making it clear that they cannot afford to ignore fraud detection. This article provides a deep dive into how Sift addresses these pressing challenges, ensuring organizations can protect their interests effectively.
Definition of Fraud Detection
Fraud detection can be understood as a systematic approach to identify instances of deceitful practices that can harm an organization's financial integrity. It involves techniques that monitor transactions, track user behavior, and analyze data patterns to spot anomalies that signify potential fraud. This process encompasses a range of activitiesâfrom automated systems that flag suspicious activities to intricate investigations by fraud specialists. In today's increasingly complex ecosystem, having robust fraud detection methods is crucial for safeguarding assets and preserving customer trust.
Historical Context of Fraud Techniques
The world of fraud techniques has a rich history that stretches far back into ancient times, where deception was often executed through simple tricks and scams. As commerce evolved, so did the art of fraud. In the past century, with the expansion of financial markets and the emergence of new technologies, con artists have continually found innovative ways to exploit weaknesses.
- Early Techniques: In the 1990s, something as straightforward as check fraud began to grow and gave rise to response mechanisms from banks.
- The Internet Era: With the dawn of the internet, opportunities for fraud expanded. Online scams like phishing attacks emerged, taking advantage of unsuspecting users' trust.
- Todayâs Landscape: The landscape has transformed with algorithms and machine learning models harnessed for fraud detection. Still, fraudsters are becoming adept at evading detection systems, making it a game of cat and mouse.
Understanding these historical contexts is vital. The adaptive nature of fraud requires an equally evolving response; hence, innovations in fraud detection are imperative to remain one step ahead. Ultimately, this section highlights how far we have come and sets the stage for the introduction of Sift as a modern solution for detecting and preventing fraud in today's digital age.
Understanding Sift as a Solution
As businesses increasingly navigate a digitalized environment, the stakes of security and trust grow higher. Understanding Sift as a Solution is pivotal in this landscape, where traditional methods of fraud detection often fall flat under the weight of sophisticated cyber threats. By diving into Siftâs unique approach, we unearth not just how it functions but also why it stands out in a saturated market. The intricacies behind its technology, combined with the specific features it offers, provide businesses with the tools necessary to maintain the delicate balance between mitigating risk and fostering customer trust.
Overview of Sift's Technology
Siftâs technology harnesses a blend of advanced algorithms and data analytics, fusing them into a cohesive system tailored for real-time fraud detection. One of the cornerstones of this technology is its use of machine learning, enabling it to adapt and evolve based on patterns of fraudulent behavior. This adaptability is essential, as fraud tactics continuously shift and diversify. For instance, Sift analyzes user behaviors, aggregating data points from various sources. This might include device type, geographic location, and previous transactions, to establish a comprehensive risk profile for each interaction.
Moreover, Sift leverages artificial intelligence to automate decision-making. When a transaction is initiated, the system evaluates the associated risk score almost instantaneously. This immediate feedback can significantly decrease the chances of friction in user experiences, while concurrently improving the accuracy of fraud prevention efforts. The technology is not just reactive but also predictive, mapping out potential fraud pathways before they become a reality.
Key Features of Sift Fraud Detection
Sift's solution encompasses several key features that not only bolster its effectiveness but also position it uniquely against competitors. These include:
- Adaptive Machine Learning: This feature learns from both legitimate and fraudulent transactions. Each new data point enhances the systemâs ability to predict future threats more accurately.
- Customizable Risk Assessment: Organizations can tailor the platform to fit their specific needs. Risk thresholds can be adjusted to accommodate the varying levels of risk associated with different transactions or user behaviors.
- Global Data Network: By tapping into a vast network of transaction data across various industries, Sift can identify anomalies that might not be visible within a singular organizational context.
- Real-Time Analytics: This functionality ensures that decisions are made at the speed of the transaction, which minimizes fraudulent activities effectively without causing undue delays for genuine customers.
"In todayâs fast-paced digital economy, the ability to scale fraud detection techniques without compromising user experience is no longer just an option but a necessity."
In summary, Siftâs technology not only embodies innovation but also integrates seamlessly into existing infrastructures, thereby enhancing security measures across diverse sectors. As we continue to explore Siftâs applications and benefits, itâs crucial to recognize that its roots lie deep in a sophisticated blend of technology designed for a world that demands immediate, reliable, and effective fraud prevention.
Mechanisms Behind Sift
Understanding the mechanisms behind Sift is crucial for grasping how this technology operates to combat fraud effectively. Siftâs approach is deeply rooted in various sophisticated methodologies including machine learning, data analysis, and real-time monitoring. These elements collectively contribute to its efficacy in identifying and mitigating fraudulent activities while maintaining operational efficiency.
Machine Learning Algorithms in Sift
Machine learning is the heart of Sift's operations. At its core, Sift employs various algorithms that constantly learn from new data to enhance accuracy in detecting fraud. This is much like teaching a child; the more experiences they encounter, the more adept they become at discerning patterns.
- Adaptive Learning: Siftâs algorithms adapt to changing fraud patterns, which is imperative in a landscape where deceit evolves continually. This allows businesses to stay one step ahead of cunning fraudsters who find new ways to exploit systems.
- Anomaly Detection: By utilizing advanced statistical techniques, Sift identifies unusual patterns within massive datasets. If a user suddenly alters their purchasing habits, for example, the system flags it for review.
- Predictive Modeling: Algorithms are trained on historical fraud data to predict the likelihood of future fraud occurrences. This proactive stance is key in resource allocation and risk management.
Implementing machine learning leads to smarter systems and helps in drastically reducing false negatives, that is, genuine transactions incorrectly flagged as fraudulent.
Data Analysis Techniques Utilized
Data analysis techniques in Sift enable deeper insights into user behavior and transaction patterns. The sophisticated methodologies employed are not only about analyzing a static set of numbers but also about interpreting the context surrounding these numbers.
- Behavioral Analysis: By monitoring user interactions, Sift builds profiles that detail normal behavior patterns. This allows for more accurate judgments about activities that might seem suspicious.
- Cluster Analysis: This technique helps in understanding groups of similar transactions. For instance, if numerous purchases from the same IP address occur in a short timeframe, cluster analysis can identify this as potentially problematic.
- Risk Scoring: Every transaction is assessed and assigned a risk score based on multiple parameters. Higher scores trigger immediate alerts for further investigation.
"What you look for, you will find. With effective data analysis, Sift ensures that they're not just looking, but searching and understanding."
Real-Time Detection and Monitoring
Sift places significant emphasis on real-time detection and monitoring, which is vital in todayâs fast-paced digital environment. In fraud detection, time is of the essence; delays can result in considerable losses. Hereâs how Sift ensures immediacy in detection:
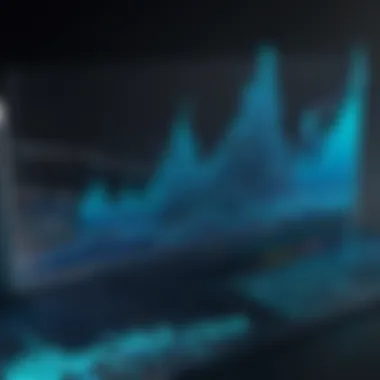
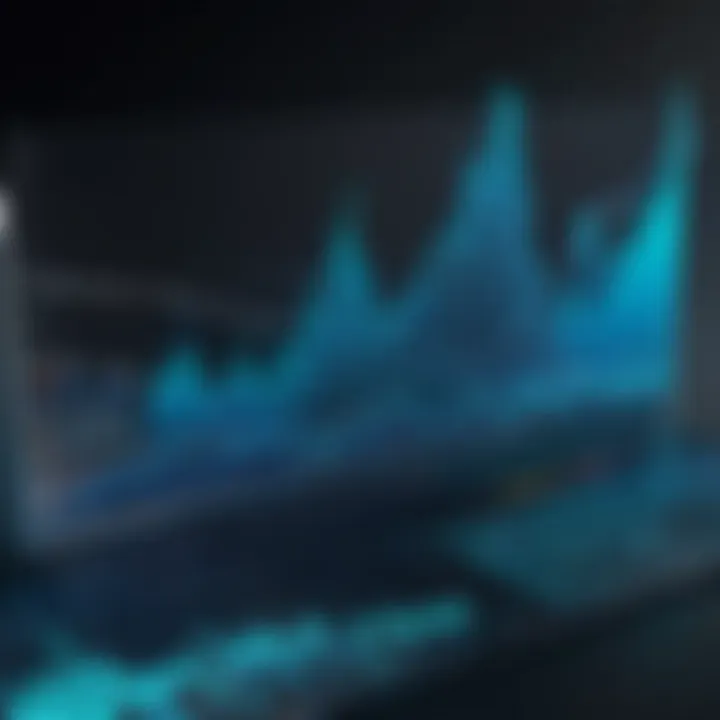
- Instant Alerts: Transactions deemed high risk are flagged instantly, allowing businesses to take immediate action. This creates a level of responsiveness that is critical in mitigating losses.
- Continuous Monitoring: The platform engages in round-the-clock surveillance of transactions. This ensures that any fraudulent activities can be caught almost at the moment they occur.
- Adaptive Real-Time Feedback: As new fraudulent patterns emerge, Siftâs monitoring system adjusts dynamically, updating itself based on the latest data inputs. This adaptability significantly enhances the systemâs effectiveness.
Through these mechanisms, Sift demonstrates a robust system that not only reacts to fraud but proactively anticipates its occurrence, creating a safer digital environment for businesses and their customers.
Applications of Sift in Various Industries
In todayâs fast-paced digital economy, industries face an escalating threat from fraud. Sift stands at the forefront of tackling this challenge, providing solutions that are not only effective but also adaptable across a range of sectors. Understanding how Sift is applied in various industries is crucial for organizations looking to enhance security measures. This section will delve into the specific areas where Sift's fraud detection capabilities create significant impacts, highlighting key benefits and considerations.
E-Commerce Sector
E-commerce has exploded in the last decade, with businesses both big and small turning to the online marketplace. But, with this rapid expansion comes the risk of fraudulent activities that can undermine trust and hurt revenues. Sift offers a robust solution tailored to the needs of the e-commerce sector. By leveraging advanced machine learning algorithms, Sift can analyze transaction data in real time, evaluating patterns that may indicate fraudulent behavior.
Key Benefits in E-Commerce:
- Real-Time Monitoring: Instant analysis helps in catching suspicious activities before they escalate.
- Customizable Rules: Businesses can configure specific criteria to automatically flag or block transactions based on their unique operating environment.
- Greater Trust from Customers: By minimizing fraud, brands foster a sense of safety, encouraging consumers to shop freely.
Utilizing Sift can be a game-changer; it transforms the user experience by creating an invisible shield that protects both customers and retailers.
Financial Services
When we think of financial services, we think of trust. Banks and financial institutions hold sensitive information and large sums of money, making them prime targets for fraudsters. Given the industry's stringent regulatory requirements, the implementation of Sift can help institutions meet compliance standards while keeping their customers safe.
Considerations for Financial Services:
- Adaptive Technology: Sift's algorithms continuously learn from new data, refining their fraud detection capabilities and keeping pace with emerging threats.
- Fraud alerts: Instant alerts notify users when suspicious transactions occur, allowing them to take immediate action.
- Reduced Fraud Losses: With a more sophisticated mechanism for detecting fraudulent transactions, banks can significantly cut down on losses from fraud while maintaining customer trust.
Adopting Sift not only enhances security but also streamlines operational processes in a sector where efficiency is paramount.
Gaming and Entertainment
The gaming and entertainment industry has seen a rapid uptick in online transactions, driven by the convenience and accessibility of platforms. However, this shift has brought along a rise in fraudulent behavior, compromising user experiences and revenue. Sift offers tailored solutions that can monitor gambling behaviors in real time, detecting anomalies that may signify fraud.
Essential Features for Gaming and Entertainment:
- Behavior Analytics: By analyzing user behavior patterns, Sift can identify deviations indicative of fraud.
- Seamless User Experience: Automated decision-making reduces friction for legitimate players while effectively filtering out potential fraudsters.
- Multi-Panel Monitoring: With users often accessing platforms from multiple devices, Sift maintains robust security across all fronts.
Implementing Sift in gaming platforms ensures an environment that prioritizes player safety while enhancing overall customer satisfaction.
"In a landscape where every click counts, safeguarding transactions is no longer optional but essential for success."
In summary, Siftâs adaptability across sectors makes it an invaluable tool in battling fraud. From e-commerce to financial services and the gaming industry, its mechanisms provide tailored solutions that not only protect businesses but also enhance customer trust. As industries continue to evolve, leveraging Siftâs strengths can become a cornerstone of a solid fraud prevention strategy.
Benefits of Implementing Sift
Implementing Sift for fraud detection brings a variety of advantages that cannot be overlooked. These benefits encompass not just improved security but also enhanced user experience and operational efficiency. In a landscape where cyber threats are becoming increasingly sophisticated, relying on Sift can mean the difference between a secure transaction and a major breach of trust. Letâs explore three core benefits in detail.
Enhanced Accuracy in Fraud Prevention
One of the standout features of Sift is its ability to enhance accuracy in identifying fraudulent activities. Traditional methods often rely on static rules or past patterns that may not accurately represent the evolving tactics of fraudsters. In contrast, Sift employs machine learning algorithms that continuously adapt as new data comes in.
For example, if a particular pattern of fraudulent activity emerges, Sift can learn from this incident and adjust its algorithms accordingly. This means that the next time a similar transaction occurs, the system can flag it with higher precision than older systems might.
"With Sift, the ability to differentiate between genuine user behavior and fraudulent interactions becomes more refined, leading to a notable decrease in both false positives and negatives."
Additionally, the data analysis techniques used by Sift are tailored to analyze vast amounts of data in real-time. This extensive scrutiny allows for a much more comprehensive view of customer behavior, thereby leading to more accurate fraud detection that goes beyond just surface-level metrics.
Improved Customer Experience
Another fundamental benefit of deploying Sift relates to the enhancement of customer experience. Trust plays a critical role in any customer interaction, particularly in e-commerce and online services. When customers know they are in a safe environment, theyâre more likely to engage, make purchases, and return for future transactions.
Sift minimizes the disruption to genuine usersâ experiences by reducing the number of false positives. False positives occur when legitimate transactions are flagged as suspicious. With Sift's smart algorithms, the likelihood of this happening is significantly curtailed. This helps maintain a smoother transaction process, where users donât have to jump through unnecessary hoops to prove their authenticity.
It also contributes to maintaining a robust customer relationship. When customers feel secure, they tend to exhibit brand loyalty. Thus, improving customer experience can lead to higher retention rates and repeat business, which are crucial for long-term success.
Streamlined Operational Efficiency
Beyond accuracy and customer satisfaction, Sift has a direct impact on operational efficiency within firms. The smart automation that Sift employs allows teams to focus their efforts on responding to genuine cases of fraud rather than sorting through a mountain of flagged transactions that may actually be safe.
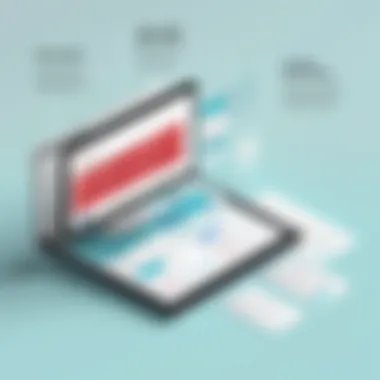
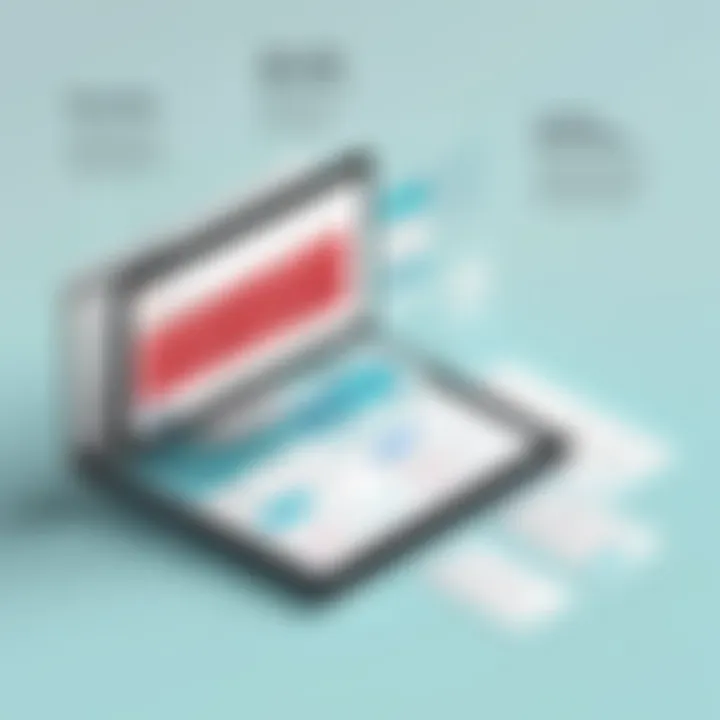
Reducing manual intervention means less time is spent on investigating alerts that are ultimately unsubstantiated. This streamlining of resources allows employees to engage in higher-value activities, such as improving product features, enhancing customer service, or developing innovative solutions to further combat fraud.
Moreover, having a highly systematized fraud detection approach often leads to cost savings. Less time and fewer resources involved in handling false alarms translate into financial benefits for organizations. This kind of operational efficiency not only saves time but can also positively impact the bottom line.
In summary, the implementation of Sift isn't just about enhancing security; it provides a multitude of intertwined benefits ranging from improved accuracy in fraud detection to a more pleasant customer experience and streamlined operations. As companies navigate through the challenges presented by digital threats, investing in robust solutions like Sift becomes essential.
Challenges and Limitations of Sift
Investigating the challenges and limitations of Sift is vital for understanding not only its effectiveness but also its boundaries when it comes to fraud detection. Every advance in technology comes with its own set of hurdles, and Sift's instance is no exception. As organizations increasingly rely on Sift to protect their assets and customer information, becoming aware of these pitfalls is essential for optimizing its usage.
False Positives and Negatives
A significant challenge faced by Sift, as with many automated systems, lies in the realm of false positives and negatives. False positives occur when the system mistakenly flags a legitimate transaction as fraudulent. This can lead to unnecessary disruptions for customers, who may find themselves having their purchases declined. In a world where customer experience is paramount, false positives can hurt a businessâs reputation. Conversely, false negatives happen when the system fails to identify an actual fraudulent transaction, allowing bad actors to slip through the cracks.
Itâs essential for businesses to strictly monitor these metrics; otherwise, the cost of lost sales or eroded trust could outweigh the benefits that Sift brings. Having a system that learns from past mistakes can be fundamental. A recent study suggested that fine-tuning machine learning algorithms could reduce false positives by as much as 30%.
Scalability Issues
As organizations grow, their requirements regarding fraud detection evolve. Siftâs performance might not always scale seamlessly. For instance, as transaction volumes spike, particularly during high seasons like holidays, businesses might find that Sift struggles to adapt. Under such pressure, the risk of both false positives and negatives may heighten, presenting a double-edged sword scenario.
When deploying Sift, itâs critical for organizations to assess their scale and growth trajectory, ensuring that the solution can accommodate increasing demands. Effective load-testing strategies can provide insights into how Sift would perform under various volumes. Moreover, businesses may need to consider cloud solutions that could enhance Siftâs scalability without compromising operational integrity.
Data Privacy Concerns
In the era of stringent data protection regulations, privacy concerns loom large, especially with data-driven services like Sift. Collecting and analyzing vast amounts of user data raises questions about consent, data storage, and potential breaches. Businesses must navigate regulatory frameworks such as the GDPR or CCPA, which create an intricate dance between ensuring security and customer privacy.
While Siftâs algorithms demand comprehensive data input to maximize accuracy, companies must strike a balance between analytical needs and regulatory compliance. Failing to do so could land organizations in legal troubles and damage their reputation. Educating the teams involved about these laws takes precedence as much as understanding the technological aspect.
"In the world of fraud detection, knowledge isnât just power; itâs a necessity to maintain trust and compliance in the digital age."
In sum, Sift presents an array of possibilities for fraud detection, yet it is not without its drawbacks. Understanding the science behind false positives and negatives, recognizing scalability limitations, and addressing data privacy concerns are substantial considerations for any organization keen on utilizing Sift. As technology continues to advance, businesses that remain informed and adaptable will likely reap the most benefit.
Case Studies of Sift in Action
When discussing fraud detection, the importance of real-world examples canât be overstated. Case studies provide context and illustrate how theoretical concepts translate into practical solutions. In the case of Sift, delving into its application through various organizations sheds light on its effectiveness and adaptability.
By examining specific instances where Sift technology has been deployed, stakeholders can better understand both its prowess and its limitations. These explorations demonstrate the technologyâs ability to mitigate risks, enhance user experiences, and promote trust among consumers. Moreover, analyzing past successes and setbacks helps in calibrating future implementations.
Success Stories
Sift has amassed quite a collection of success stories across different industries, marking its territory in fraud prevention. Take the e-commerce giant, for instance. A platform that saw an alarming rise in fraudulent activities implemented Sift and subsequently tracked a notable drop in chargebacks. This was not by mere chance; Sift's machine learning models, trained on vast amounts of transaction data, identified patterns of behavior indicative of fraudulent activities.
Another compelling example comes from the gaming industry. A popular gaming platform integrated Sift to handle a surge in account takeovers. By leveraging real-time behavior analysis, Sift efficiently discerned legitimate players from potential fraudsters. The result? A marked improvement in customer satisfaction and a decrease in operational interruptions.
Here are a few elements common in these success stories:
- Rapid Response: Clients experienced a quicker turn-around time in addressing fraudulent attempts.
- User Retention: Companies noted fewer complaints from genuine users as the false positives rate decreased.
- Financial Savings: Significant cost savings were reported, stemming from reduced chargebacks and improved transaction approval rates.
These success stories not only reflect Siftâs capabilities but also underscore its value as a go-to solution for organizations during turbulent times. In many cases, the technology is not just reactive; it's preventative, safeguarding revenue before threats materialize.
Lessons Learned from Failures
However, not all implementations have been smooth sailing. There have been instances where Sift faced challenges, leading to valuable lessons. One notable example includes a multinational retail chain that initially struggled with Sift's oversight capabilities. The integration was not seamless, resulting in a spike in false positives, which alienated loyal customers and even skewed sales data.
Key takeaways from such setbacks include:
- Importance of Calibration: Companies must invest time in tuning the algorithms to match their specific context, as what works for one might not for another. Siftâs models need to understand unique behaviors regular to a certain sector or even a particular company.
- User Education: Continuous communication with users, especially after implementation, can curb misunderstandings and build trust. When customers are informed about security protocols, they're generally more accepting of security measures.
- Real-Time Monitoring: Establishing a robust mechanism for monitoring Siftâs outputs can help in promptly identifying discrepancies, allowing companies to refine strategies before significant loss occurs.
A crucial aspect of these lessons is the reminder that technology alone won't solve every problem. The human element remains irreplaceable in navigating the complex landscape of fraud detection. While Sift provides the tools, users must synergize with the technology for optimal outcomes.
Future Directions in Fraud Detection
Understanding the future of fraud detection is crucial in maintainig the security of digital transactions and the safety of user data. As technology evolves, so do the methods used by fraudsters. Therefore, it's essential to anticipate the trends and tools that will define the landscape in the coming years. In the context of Sift, the focus on upcoming techniques provides insights not only into enhancing detection capabilities but also into improving user trust.
Emerging Technologies
New technologies are emerging faster than you can say "cybersecurity". Innovations like blockchain, which is inherently secure and decentralized, are being explored to strengthen fraud detection systems. By leveraging these technologies, organizations can create systems that ensure transactions are legitimate and enhance transparency.
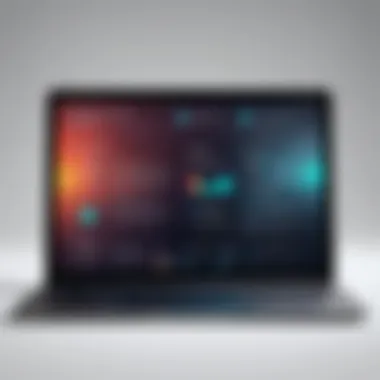
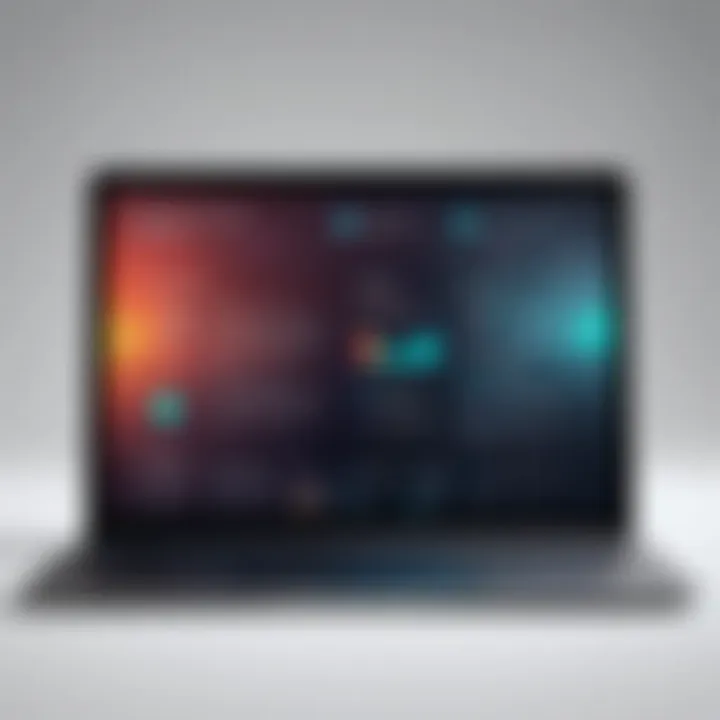
- Blockchain represents a way to track transactions that cannot be easily altered.
- IoT Devices collect vast amounts of data, which can be analyzed for fraud signals. One can monitor behaviors across different devices and establish more robust detection frameworks.
- Cloud Solutions offer scalability and flexibility. They allow businesses to process and analyze data in real-time, making it easier to spot discrepancies that signal fraudulent activity.
By integrating these technologies, organizations can stay ahead of fraudsters, who are constantly developing new tactics.
AI and Predictive Analytics
AI is not just a buzzword anymore. Itâs become a key player in fraud detection. Machine learning algorithms allow systems to learn from past data and improve their predictions over time. This adaptability is essential as fraud schemes evolve.
- Predictive Modeling enables businesses to forecast potential fraud based on historical data, which can help in allocating resources effectively.
- Anomaly Detection identifies unusual patterns that deviate from the norm, giving early warnings about potential fraud.
AI-driven tools can sift through enormous amounts of data quickly, identifying patterns that humans might miss. This capability not only ensures a faster response but also reduces the chances of false positives, which can annoy customers and waste resources.
Regulatory Changes Impacting Detection
As digital transactions grow, regulations are being updated to better protect consumers. Understanding the regulatory environment is vital since compliance can significantly impact how fraud detection systems are built and deployed.
- General Data Protection Regulation (GDPR) mandates strict data protection policies that affect how companies handle personal information. Companies must be transparent about how they use data, which can directly influence fraud detection strategies.
- Payment Services Directive (PSD2) in Europe changes the way financial institutions approach security, emphasizing stronger customer authentication.
These regulations add layers of complexity to fraud detection but also present opportunities for enhanced consumer trust. Businesses that adapt proactively will likely gain a competitive edge.
"Staying ahead of the regulations not only protects businesses but also reinforces trust among consumers."
Comparative Analysis of Fraud Detection Solutions
In the fast-evolving realm of cyber threats, understanding the various fraud detection solutions available is not only crucialâitâs a lifeline for many organizations. A comparative analysis serves as a beacon, shedding light on different methodologies employed by solutions like Sift and its competitors. It highlights their strengths, weaknesses, and overall effectiveness in combating fraudulent activities, all while keeping customer trust intact. By delving into the uniqueness of each solution, businesses can make informed decisions that suit their specific needs.
Sift vs. Competitors
When putting Sift under the microscope alongside its competitors, the differences become quite apparent. For instance, Sift employs a robust mechanism that balances machine learning with constant data analysis. In comparison, some rivals might lean heavily on rule-based approaches. While rule-based systems can catch obvious fraud patterns, they often fall short in scenarios where fraudsters adapt quickly.
Here are some key points of differentiation:
- Diversity of Data Sources: Sift thrives on utilizing a plethora of data sourcesâuser behavior, device data, and even historical transaction patterns. Others may not integrate as many variables, risking blind spots in detection.
- User Experience: One notable aspect in which Sift excels is in maintaining customer satisfaction. Complicated verification processes can turn customers away. Siftâs methods often feel seamless, reducing friction while still preserving security.
- Scalability: Many traditional systems struggle when transactions surge, especially during peak seasons. Sift is designed to handle high volumes without sacrificing accuracy.
Ultimately, the choice between Sift and its competitors hinges on the specific landscape of a business's operations. Companies need to weigh several factors, including their customer base, transaction volume, and unique vulnerabilities to fraud.
Cost-Benefit Considerations
When considering any fraud detection solution, the cost-benefit matrix becomes essential. On the surface, it might seem straightforward; however, it dives into deeper waters when you start examining concealed costs and long-term benefits.
- Initial Investment vs. Long-Term Savings: Solutions like Sift may command higher pricing initially but provide savings down the line through reduced charges from fraud losses and less need for customer service interventions.
- Integration Costs: Deploying new systems isnât as easy as pie. Organizations must consider the costs associated with integrating Sift into existing frameworks.
- Brand Reputation: A single fraud incident can tarnish a brand's reputation. A robust solution like Sift not only provides defense against losses but also helps in preserving trust with consumers. This is a benefit often overlooked when examining short-term costs.
In essence, conducting a thorough cost-benefit analysis when selecting a fraud detection solution is imperative. It ensures alignment of business goals with the chosen technology, ultimately positioning the organization to tackle fraud more effectively.
"Choosing the right fraud detection solution is akin to selecting armor for a knightâwhat works for one battle may not be suited for another. Itâs all about purpose and context."
In the journey of assessing solutions, firms can create roadmaps tailored to their objectives, ensuring that every dollar spent is an investment in their future resilience against fraud.
End and Final Thoughts
In the intricate world of fraud detection, this discussion on Sift stands as a cornerstone for understanding not just the technologies involved, but also the broader implications they carry for businesses across various sectors. Fraud detection is no longer a luxury; it is a necessity that influences the way companies protect their revenue and safeguard consumer trust. As we navigate through the realms of cyber threats and aggressive fraud tactics, Sift's methodologies offer tangible solutions that adapt and evolve in real time.
The key takeaways from this exploration revolve around several specific elements:
- Holistic Approach: Sift employs a multi-faceted strategy, integrating machine learning and data analysis to enhance detection rates. This is not just about blocking fraudulent transactions; itâs about understanding user behavior on a granular level.
- Industry Relevance: The application of Sift spans various industries, from e-commerce to financial services. This adaptability underscores its value in diverse environments where fraud can manifest in different forms.
- Continuous Feedback Loop: By learning continuously and adapting algorithms based on emerging patterns, Sift ensures a proactive rather than reactive stance on fraud.
As we evaluate the benefits Sift bringsâlike increased confidence in transactions and improved customer experiencesâit becomes clear that the tool is more than just software; it is a strategic ally in crafting a secure digital realm. Many businesses find themselves weighing the cost against potential losses from undetected fraud, which often tips the scale towards investing in robust systems like Sift.
Ultimately, businesses should not merely adopt new tools; they must embrace a mindset geared towards continuous improvement.
Summarizing Key Insights
Reflecting on the insights gathered, a few striking points stand out:
- Proactive vs. Reactive: Traditional fraud detection often relies on historical data, leading to a reactive rather than proactive methodology. In contrast, Sift emphasizes a forward-looking approach, anticipating threats.
- Data-Driven Decisions: The reliance on real-time data affords businesses the ability to make informed choices. Collaboration between datasets generates a holistic view of transactions, which is vital in understanding user intent.
- User Trust: At the end of the day, the battle against fraud is also about user trust. A smooth, secure user experience can significantly affect customer loyalty and retention.
The Role of Continuous Improvement
Continuous improvement should be a fundamental principle guiding companies that handle sensitive transactions. In the context of fraud detection, this means keeping pace with technological advancements and emerging threats. Siftâs infrastructure implies not only automated learning but a culture of agility and adaptability within organizations.
- Feedback Mechanism: Regularly analyzing outcomes and incorporating feedback is crucial. An effective fraud detection strategy involves learning from past fraud attempts and adjusting techniques accordingly.
- Innovation: The tech landscape is always shifting; what works today may not work tomorrow. Hence, staying updated on new research and enhancing existing systems keeps a company ahead of the curve.
- Training and Awareness: Continuous improvement also extends to the human aspect. Training staff to recognize heuristic signs of fraud plays a big role in creating a robust defense system.
As technology advances, the tools for equality must also evolve. Businesses leveraging Sift should continuously re-evaluate their strategies, ensuring they align not just with current trends but also with future projections in fraud detection. In this ongoing journey, the willingness to embrace change will determine long-term success in maintaining integrity and security in digital transactions.