Exploring DataRobot: Features and Applications
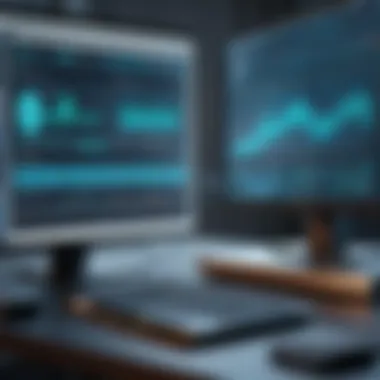
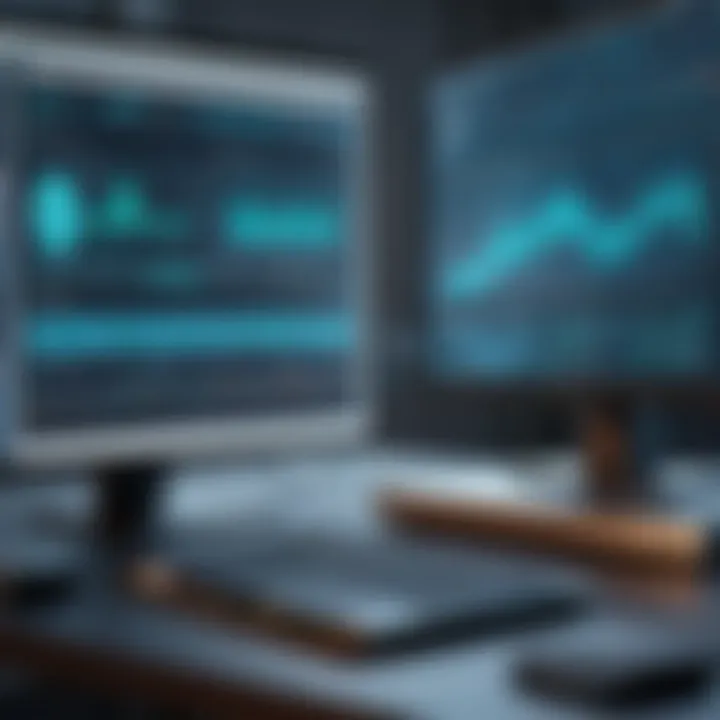
Intro
DataRobot is becoming a significant player in the field of automated machine learning. The importance of understanding its demo features cannot be overstated. This exploration serves as a guiding light, offering insights into how this platform operates and the various capabilities it presents.
The demo sessions enable users to witness firsthand how DataRobot can redefine data science workflows. These sessions showcase the simplicity and power of working with the platform. Users, whether seasoned professionals or newcomers, can benefit greatly from engaging in these demonstrations.
Next, this article will delve into the core features and overall performance, emphasizing areas that directly affect user experience.
Prolusion to DataRobot
Understanding DataRobot is crucial for anyone interested in advancements in machine learning and data analytics. This section serves as a foundation for the entire article, enabling readers to grasp the essential role that DataRobot plays within the realms of AI and data science.
DataRobot’s significance lies in its capability to automate various machine learning tasks, making it accessible for users who may not have extensive technical backgrounds. The platform simplifies complex processes, such as data preprocessing, feature selection, and model deployment, allowing it to cater to both skilled data scientists and less experienced users who wish to leverage advanced analytics without delving deep into intricate programming.
Additionally, an overview of its features can illuminate how DataRobot optimizes productivity and enhances decision-making across industries. Its benefits extend beyond traditional applications. As businesses continue to confront data challenges, understanding DataRobot’s potential can amplify their analytics strategies, thus leading to more informed conclusions and effective solutions. By exploring this section and the subsections that follow, readers will better appreciate DataRobot’s role in the modern data landscape.
Overview of DataRobot
DataRobot is an end-to-end automated machine learning platform that aims to make the power of AI accessible to all. It streamlines the process from data ingestion to model deployment, significantly reducing the time and resources traditionally required for data science projects. Users can upload data sets with ease, allowing DataRobot to perform quick analyses and develop predictive models.
The platform provides an intuitive user interface, enabling users to visualize model performance and make adjustments as necessary. Moreover, its diverse collection of pre-built algorithms ensures optimal performance, addressing a wide array of business problems. DataRobot supports various data types, and its scalability allows it to cater to both small and large data sets effectively.
The Role of AI in DataRobot
Artificial intelligence is at the heart of DataRobot’s functionality. The platform harnesses machine learning algorithms and tools to process large datasets, recognizing patterns and drawing insights that may not be apparent through manual analysis. This capability not only expedites the analysis but also enhances the accuracy of predictions.
AI within DataRobot automates critical components of the machine learning workflow. These include data preparation, feature engineering, and model evaluation, which can often be time-consuming and labor-intensive if performed manually. By integrating AI, DataRobot allows users to focus on interpreting results rather than getting bogged down in the intricacies of model building.
Furthermore, the platform’s use of AI facilitates continuous learning. As new data is introduced, DataRobot can adapt its models, thus maintaining their relevance and potency in changing environments. This aspect is particularly vital for businesses that rely on real-time data for decision-making.
"DataRobot allows both expert and novice users to harness the power of AI, making advanced analytics more accessible than ever."
In summary, DataRobot’s role in the landscape of data science is paramount. It democratizes access to machine learning, brings efficiency to data analysis, and leverages the capabilities of AI to deliver insights that can transform data into actionable strategies.
Understanding the Demo Feature
The demo feature of DataRobot serves a pivotal role in demonstrating how the platform can be utilized in various data science endeavors. Understanding this feature is crucial for users who want to ascertain how DataRobot can facilitate machine learning processes. The demo provides a hands-on experience, allowing prospective users to observe the functionalities in action. It highlights the intuitive design and capabilities of DataRobot, showcasing its user-friendly interface and robust analytics tools.
Purpose of the Demo
The primary purpose of the DataRobot demo is to equip participants with a real-time understanding of the platform. Demos illustrate how to import data, build models, and generate predictive insights seamlessly. Moreover, the demo serves as an educational tool. It allows users to grasp the complexities of machine learning without the steep learning curve typically associated with it. Through guided sessions, participants can also explore the various functionalities that DataRobot offers, including automated feature engineering and model selection. This interactive component is essential for users to build confidence in their ability to navigate and utilize the platform effectively.
Key aspects of the demo purpose include:
- Familiarization with the Interface: Users are walked through the layout, ensuring they know where to find essential tools and resources.
- Showcasing Capabilities: Important features, such as data visualization and prediction accuracy, are highlighted to demonstrate the strength of DataRobot.
- Hands-On Experience: Participants engage with the platform, allowing them to practice skills and understand the workflow.
Who Should Participate in the Demo?
The DataRobot demo is designed for a diverse audience, making it suitable for various professionals. It is particularly beneficial for data scientists, IT professionals, researchers, and students who seek to enhance their knowledge in data science and machine learning.
Specific groups that would find value in attending the demo include:
- Data Analysts: Those looking to develop predictive models and better understand data trends will benefit greatly from the insights shared during the demo.
- Business Leaders: Executives and managers exploring data-driven decision-making strategies can see firsthand how DataRobot can impact their operations.
- Students: Individuals studying data science or related fields can learn about industry-standard tools and practices, enriching their educational experience.
In summary, understanding the demo feature of DataRobot is essential for maximizing its utility. By participating in the demo, users can develop a clearer perspective on how this platform can enhance their data analytics capabilities.
Components of the DataRobot Demo
The Components of the DataRobot Demo section is crucial for understanding what the demo consists of and how these features collectively showcase the platform's capabilities. These components not only provide a user with a framework of how DataRobot functions but also highlight the advanced technologies behind it. Understanding the components helps potential users see the value and the practical applications of the tool in real-world scenarios.
User Interface Overview
The user interface (UI) of DataRobot plays a central role in how users interact with the platform. A well-designed UI enhances user experience and enables data scientists, businesses, and IT professionals to efficiently navigate the tool’s extensive functionalities.
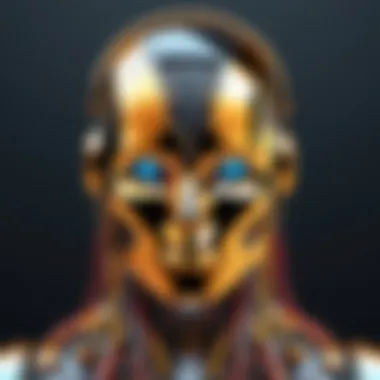
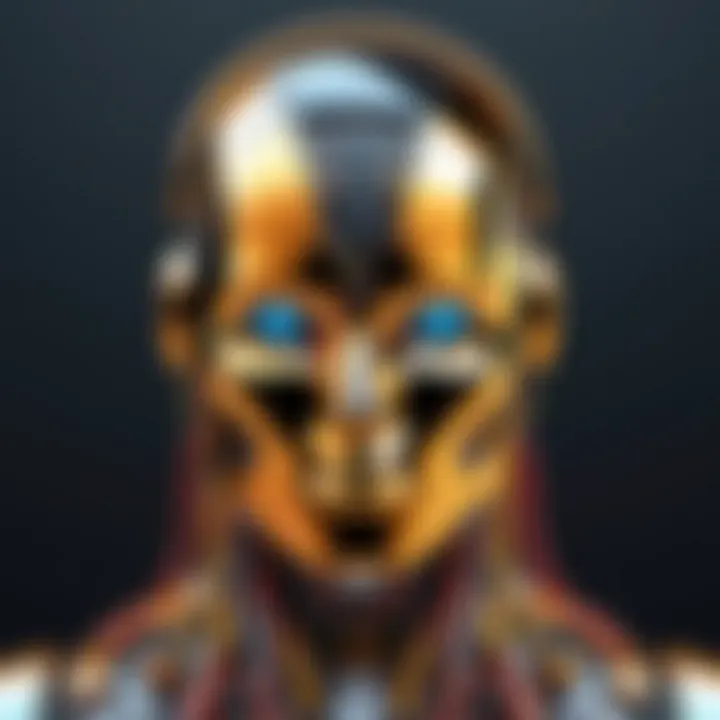
At first glance, the DataRobot interface is intuitive, making it easier for both experts and novices to use. The layout is organized into different sections like project creation, data input, model deployment, and performance metrics. Each function is accessible and designed to simplify interactions.
Key elements include:
- Dashboard: This serves as the central hub, displaying important metrics and ongoing project statuses.
- Project Workspace: This area allows users to manage projects with ease, including data uploading and model management.
- Modeling Choices: Users can select modeling options and monitor the training process visually, which aids in comprehending the machine learning lifecycle.
One significant aspect is the responsiveness of the UI. Whether on desktop or mobile, DataRobot maintains performance, allowing users to stay connected with their projects wherever they are. Overall, a well-designed user interface makes the demo more effective by allowing participants to focus on understanding the underlying functionalities rather than getting lost in navigation.
Key Functionalities Demonstrated
During a DataRobot demo, various key functionalities are presented that showcase its powerful capabilities. These functionalities are foundational to understanding how DataRobot can be applied across different industries:
- Automated Machine Learning: DataRobot automates critical processes from data preparation to model selection, reducing the time and effort needed by users. It demonstrates how users can perform predictive modeling with minimal manual intervention.
- Visualization Tools: The demo showcases advanced visualization tools that help users understand data insights, model performance, and outcomes effectively. Such tools facilitate better decision-making based on data.
- Collaboration Features: The platform emphasizes sharing and collaboration tools, making it easy for teams to work on projects together and leverage collective knowledge.
These functionalities collectively illustrate DataRobot's efficiency and speed in providing solutions to complex data problems, thus making it a vital tool for any data-driven organization. Furthermore, showing these components in a demo not only builds trust in the product but also demonstrates its practical utility in making informed business decisions.
Technical Aspects of DataRobot
The technical aspects of DataRobot are crucial for understanding how this platform operates and supports various machine learning initiatives. These elements allow professionals to leverage the full potential of data analytics and its integration with existing systems. A clear grasp of these factors can significantly impact the effectiveness of machine learning projects.
Integration with Data Sources
DataRobot supports seamless integration with multiple data sources. This is essential for any organization that aims to streamline its data analysis processes. By connecting with various databases and data storage solutions, DataRobot enables users to pull in data from sources such as MySQL, PostgreSQL, and cloud services like Amazon S3 or Google Cloud Storage.
The integration process is designed to be user-friendly, allowing even those with limited technical knowledge to set up connections quickly. The ability to unify disparate data sources in one platform enhances data accessibility. This saves time and resources, which can be allocated to more analytical tasks instead.
Furthermore, integrating various data types into one coherent dataset is vital for training machine learning models effectively. It enables the models to learn from diverse inputs, which can enhance their predictive accuracy. Organizations must ensure that they understand the data structures being integrated to maintain data integrity and quality.
Automation of Machine Learning Processes
Automation within DataRobot is a key feature that sets it apart from traditional machine learning platforms. This functionality allows users to automate repetitive tasks involved in the machine learning pipeline, such as data preparation, model selection, and hyperparameter tuning.
Automation benefits users in several ways:
- Efficiency: Users can complete complex processes more rapidly, reducing the time from data ingestion to model deployment.
- Consistency: Automated processes minimize human error, leading to more reliable results.
- Scalability: Organizations can easily scale their data projects without needing proportional increases in manpower.
DataRobot's automation features are not just limited to model training. Users can schedule batch predictions and manage model deployments through automated workflows. This translates to quicker insights, allowing businesses to respond promptly to market changes.
"Automation in machine learning is vital for advancing productivity and maintaining high-quality outcomes."
In summary, both integration with data sources and automation processes are critical technical aspects of DataRobot. These features play a significant role in maximizing the efficiency and effectiveness of machine learning initiatives, paving the way for organizations to drive impactful analytics.
Use Cases of DataRobot
Use cases of DataRobot are pivotal to understanding its practical value in various domains. This section explores how organizations harness DataRobot to derive insights and drive decision-making. By examining specific industries and practical applications, professionals can appreciate how DataRobot fits into diverse business scenarios. The relevance of these use cases extends beyond simple application; they show DataRobot's ability to enhance productivity and decision accuracy.
Industries Leveraging DataRobot
DataRobot has made significant inroads across multiple industries, showcasing its adaptability and efficiency. Here are some sectors where DataRobot is particularly effective:
- Finance: Financial institutions leverage DataRobot for risk assessment and fraud detection. Machine learning models help in analyzing patterns from historical data, enabling quicker and more accurate decision-making.
- Healthcare: Hospitals and pharmaceutical companies utilize DataRobot to predict patient outcomes and optimize treatment plans. These models help in personalizing medical care, which can lead to improved patient satisfaction and health results.
- Retail: Retailers employ DataRobot to manage inventory and enhance customer experiences through targeted marketing. Predictive analytics assist them in understanding customer preferences and inventory needs better.
- Manufacturing: DataRobot supports predictive maintenance and quality control in manufacturing. By analyzing equipment data, organizations can predict failures and reduce downtime.
The diverse applications across these industries underscore the flexibility of DataRobot. Each use case demonstrates how machine learning can create tangible benefits, reinforcing the platform's value.
Practical Applications in Business
Organizations utilize DataRobot to convert data into actionable insights in numerous practical applications. These applications often involve automating complex processes and enhancing the overall performance of teams.
- Customer Churn Prediction: Businesses use DataRobot for identifying customers likely to disengage. By understanding the factors leading to churn, companies can take preemptive action to retain valuable clientele.
- Product Demand Forecasting: Companies can predict future demand for products, allowing them to optimize production schedules and inventory. This approach minimizes waste and ensures that businesses meet customer needs efficiently.
- Credit Scoring Models: Financial institutions create advanced credit scoring models with DataRobot. These models assess the creditworthiness of applicants, improving the lending process and reducing defaults.
- Employee Attrition Analysis: HR departments analyze employee data to identify patterns influencing turnover. This application aids in developing strategies to enhance employee retention and workplace satisfaction.
The practical applications mentioned here are just a portion of how DataRobot can reshape business operations. The platform allows users to explore various scenarios, helping organizations make informed decisions.
"DataRobot empowers businesses to become data-driven by providing the tools necessary to extract actionable insights from their data."
Understanding these use cases makes clear why DataRobot is a leader in the machine learning platform space. Its capabilities are not limited to theory but translated into real-world solutions making it indispensable in modern business strategies.
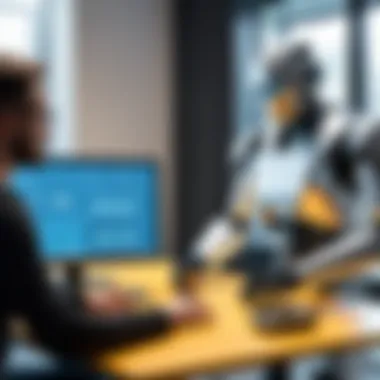
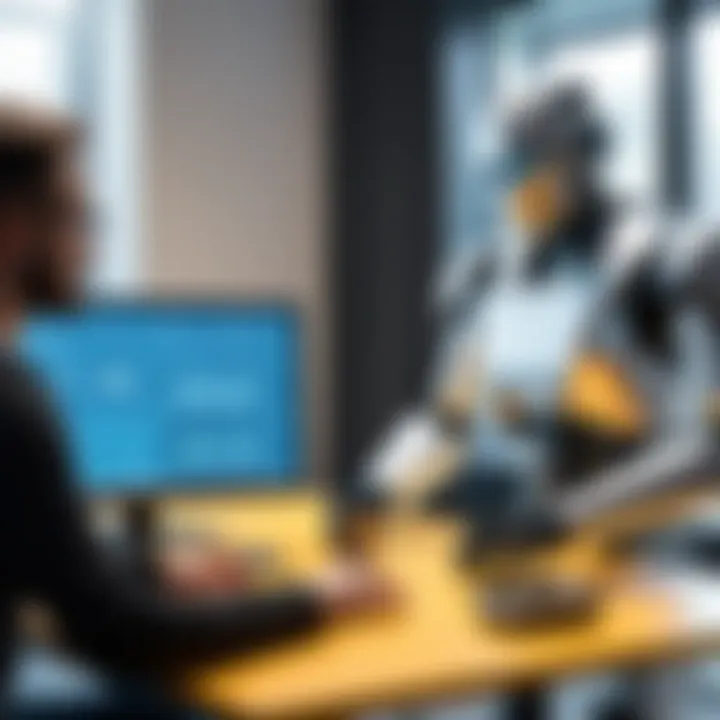
Preparing for the Demo
Preparing for the DataRobot demo is a critical phase that can significantly impact the overall effectiveness of the session. Adequate preparation not only enhances the audience's understanding of the platform but also reinforces the capabilities of DataRobot as a leading machine learning automation tool. Effective preparation sets the stage for engaging presentations that resonate with both experienced professionals and newcomers alike. Through careful planning and organization, presenters can effectively communicate the value and functionality of DataRobot while highlighting its utility in real-world applications.
Essential Pre-Demo Steps
Before the demo begins, certain essential steps must be taken to ensure a smooth flow of information. Start by defining the objectives of the demonstration clearly. This includes determining what you want the participants to learn by the end of the session. Aligning the goals with the audience’s expectations is essential.
Next, familiarizing yourself with the specific features and functionalities of DataRobot that will be showcased is paramount. This requires understanding the common user queries, as well as pain points that DataRobot addresses. Being prepared to address these will increase the credibility of the presentation.
Furthermore, it is wise to prepare an agenda or outline that covers all the key aspects to be discussed during the demo. This not only assures the audience that the session is organized but also serves as a roadmap for the presenter, keeping discussions focused and productive.
Before the demo, conduct a test run using the demo environment to identify any technical issues that may arise. This is particularly important for software demonstrations, as technical glitches can disrupt the flow and reduce audience engagement.
Gathering Data for Demonstration
Gathering relevant data is equally crucial for a successful demo. The data used should reflect realistic scenarios that potential users may encounter, thereby making the demonstration more relatable and impactful. Using synthetic datasets can also be advantageous, providing a controlled environment to showcase DataRobot’s capabilities without compromising sensitive information.
You must also ensure that the data is clean and properly formatted. Poor data quality can lead to inaccurate predictions and outcomes, undermining the credibility of the demonstration. Prepare corresponding scripts or workflows in advance to demonstrate automated processes effectively.
Additionally, organizing the data with clear labeling can help make the demonstration more informative. This organizational effort allows for quick access during the presentation, making the session more fluid and easy to follow.
"Effective preparation is a vital aspect of a successful DataRobot demo. It ensures that both the presenter and the audience are aligned, increasing the likelihood of a meaningful exchange of ideas."
By focusing on these preparatory steps, presenters will be equipped to lead a demo that not only informs but also engages the audience in exploring the multifaceted world of DataRobot.
Conducting the DataRobot Demo
Conducting a DataRobot demo is a crucial step in effectively showcasing the platform's capabilities. The way the demo is performed can significantly impact the audience’s understanding and perception of DataRobot. It is not merely a presentation of features, but a strategic opportunity to illustrate the platform's integration into real-world scenarios. Presenters should approach this task with clarity and purpose, as it can lead to insightful discussions that help potential users grasp the value of DataRobot for their specific needs.
Best Practices for Presenters
When conducting the demo, presenters should keep a few best practices in mind:
- Know Your Audience: Tailor the presentation to the audience's background and experience. Software developers may appreciate more technical details, while business professionals might focus on practical applications.
- Use Real-World Examples: Incorporating case studies or examples that align with the audience's industry will resonate better. For instance, presenting an example of how a retailer used DataRobot to optimize inventory management can create relevance.
- Stay Clear and Concise: Avoid overwhelming participants with technical jargon. Focus on clear explanations and guided navigation through the platform’s features.
- Encourage Interaction: Invite questions and feedback throughout the demo. This encourages engagement and can lead to a deeper understanding of how DataRobot applies to their organization.
- Follow a Structured Outline: A well-organized presentation will help maintain the audience's attention. Begin with an overview, outline key features, and end with practical implications.
Applying these practices can enhance the effectiveness of the demo, ultimately leading to a more engaged and informed audience.
Engaging the Audience After the Demo
Post-demo engagement is as important as the demo itself. Following up with the audience can solidify the information shared and pave the way for further discussions.
- Q&A Session: Dedicate time for questions and answers at the end of the demo. This allows attendees to clarify doubts and ensures they leave with a better understanding of the platform.
- Provide Resources: Sharing supplementary materials, like access to DataRobot’s documentation or helpful links, can aid further exploration.
- Share Contacts: Make sure attendees know how to reach you or the DataRobot team for any future inquiries. Personal connections can cultivate ongoing conversations.
- Solicit Feedback: Ask attendees for their impressions of the demo. What resonated most with them? Understanding their perspectives can help tailor future demos and improve overall effectiveness.
Engaging with your audience post-demo not only reinforces learning but also establishes a relationship conducive to future collaborations.
By following these strategies, presenters can create a meaningful experience that informs and inspires. Conducting the DataRobot demo effectively can be a game-changer for many organizations seeking advanced data analytics solutions.
Post-Demo Follow-Up
Post-demo follow-up is a crucial phase in the engagement process with potential users of DataRobot. It serves to solidify the insights gained during the demonstration and address any lingering uncertainties. A methodical approach post-demo can greatly enhance the overall perception of the platform. Most importantly, it fosters a continued relationship with participants, opening doors for deeper conversations about their needs and how DataRobot can address them.
Collecting Feedback
Feedback collection is a fundamental component of the post-demo follow-up. It not only demonstrates respect for the participants' views but also provides invaluable insights into the effectiveness of the demo presentation.
- What aspects did they find most beneficial?
- Were there any features that confused them?
- How well did the demonstration align with their expectations?
These questions can help presenters refine their future demonstrations. Employing various methods for gathering feedback is effective:
- Surveys: Using tools like Google Forms or SurveyMonkey enables easy analysis of responses.
- Direct Communication: Engaging participants in a conversation can yield more in-depth insights and foster deeper relationships.
- Follow-Up Emails: Crafting a personalized follow-up email can prompt responses that are much more detailed.
Organizing feedback effectively enhances the participants' experience and provides insights that help improve future demos. It can also confirm whether or not the demo resonated with the audience, which is crucial to converting interest into actionable leads.
Addressing Common Questions
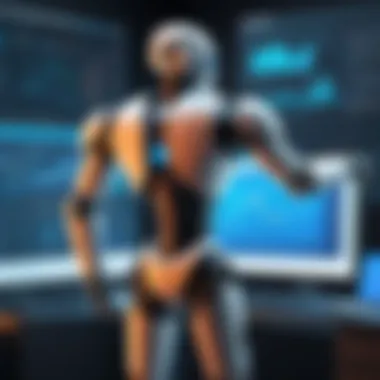
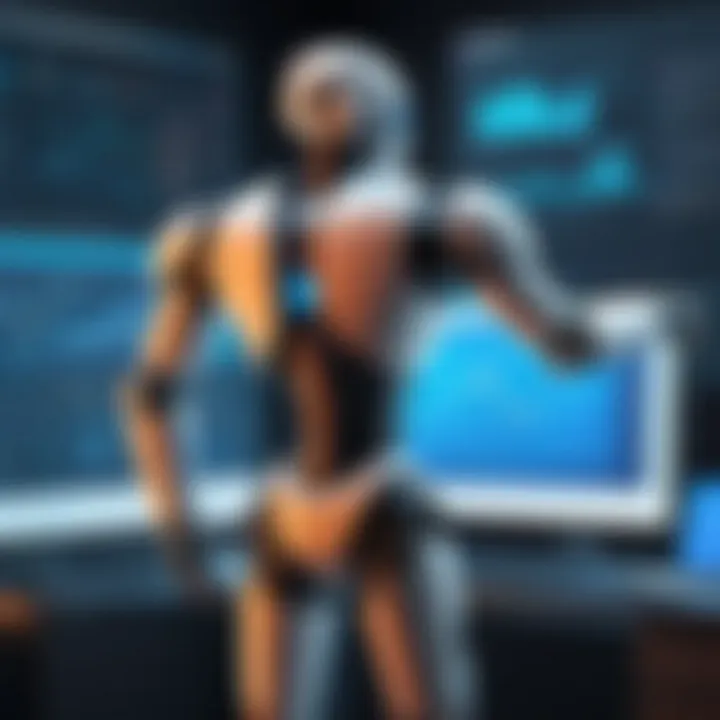
Addressing common questions that arise post-demo is essential. Often, participants may have concerns or queries about the practical use of DataRobot after the presentation.
- Clarifications on Features: Ensuring the audience understands specific functionalities can aid greatly in their assessment of the platform.
- Implementation Queries: Many participants may wonder how DataRobot can be applied within their existing workflows or systems.
- Data Security Concerns: Questions about data security and compliance are valid concerns, particularly in industries handling sensitive information.
Responding promptly and thoroughly to these inquiries can not only alleviate concerns but also demonstrate a commitment to customer support. Moreover, this additional layer of interaction can reinforce the value proposition of DataRobot, making it clear how it stands out in a competitive market.
"Engaging with participants post-demo is not just about answering questions; it’s about building a foundation for ongoing communication that can foster innovation and address needs effectively."
Evaluating DataRobot's Impact
Evaluating the impact of DataRobot is an essential step for users who want to gauge the platform's effectiveness in their data science projects. Understanding this impact involves not just assessing performance metrics but also considering how well DataRobot integrates with existing workflows. Users of DataRobot, especially those involved in software development, IT fields, or even academic research, need to perceive how this platform enhances their ability to build predictive models and derive insights.
One of the primary benefits of assessing DataRobot's impact is the clarity it provides in decision-making. Users can identify which machine learning models performed best on their specific datasets, leading to informed choices about future projects. Furthermore, this evaluation process lets organizations articulate the value added by the platform in terms of achieving business objectives. As a result, companies can derive a strong return on investment (ROI) from their use of DataRobot.
Success Metrics for DataRobot Users
Success metrics are crucial for quantifying the returns on investment when using DataRobot. These metrics typically include:
- Model Accuracy: The precision of predictions made by the developed models. High accuracy signifies effective model performance.
- Time Efficiency: Measure of hours saved in model development and deployment due to automation features within DataRobot.
- Scalability of Solutions: Ability to handle larger datasets without significant losses in performance.
- User Adoption Rate: The number of users accessing and utilizing DataRobot as part of everyday workflow. Increased adoption usually correlates with user satisfaction and the platform's overall effectiveness.
Keeping track of these metrics helps teams focus on areas for improvement. It also allows them to showcase success stories within their organizations, encouraging more robust integration of DataRobot into everyday practices.
Long-Term Benefits of Using DataRobot
Using DataRobot offers several long-term benefits that extend beyond immediate project outcomes. Key advantages include:
- Consistent Improvement in Model Performance: With a huge repository of algorithms, DataRobot continuously refines its suggestions, which improves model outcomes over time.
- Enhanced Collaboration: The platform facilitates teamwork across departments. Individuals from different backgrounds can engage in the data science process, drawing from their unique experiences.
- Future-Proofing Workflows: As machine learning continues evolving, DataRobot's adaptable architecture ensures users can easily incorporate new techniques and technologies, keeping their models at the cutting edge.
- Comprehensive Training Resources: DataRobot provides extensive learning materials, allowing team members to continually upskill.
Future Trends in Data Science
The field of data science is in constant motion, shaped by advancements in technology and evolving business needs. Understanding future trends is crucial for practitioners, as these trends can dictate the trajectory of their careers and the potential applications of the tools they utilize. This section will explore the emerging trends that promise to redefine how data science functions, particularly through advancements in platforms like DataRobot.
The Evolution of Machine Learning Platforms
Machine learning platforms have undergone significant evolution over the past years. Early systems required extensive manual coding and deep expertise in statistics and programming. Complexity was often a barrier to entry for many potential users.
Now, we see a shift towards user-friendly, automated solutions. Modern platforms offer pre-built models and automated workflows that simplify the process of data analysis. DataRobot exemplifies this shift perfectly. Its combination of user accessibility and powerful capabilities attracts a broad audience, from seasoned data scientists to less tech-savvy business professionals.
Key elements driving this evolution include:
- Accessibility: Advanced algorithms are now more approachable for users lacking formal data science training.
- Automation: Tasks such as data cleaning, feature engineering, and model selection are automated, allowing users to focus on interpreting results.
- Integration: Machine learning platforms are increasingly integrating with various data sources and third-party applications, enhancing data accessibility and usability.
This evolution signals that the future of machine learning will be more collaborative, where domain experts can contribute insights without needing extensive statistical knowledge.
DataRobot's Role in Shaping the Future
DataRobot stands at the forefront of these advancements, playing a pivotal role in the future landscape of data science. By providing an enterprise-level platform, DataRobot supports organizations in harnessing the full potential of their data. Its continuous updates ensure users benefit from the latest machine learning developments.
Several contributions highlight DataRobot's influence:
- Scalability: As organizations generate more data, DataRobot offers scalable solutions to accommodate growing datasets without losing efficiency.
- Rapid prototyping: The platform allows for quick model iteration, enabling teams to experiment with several approaches easily. This speed fosters innovation.
- Support for diverse use cases: From finance to healthcare, DataRobot's adaptability makes it suitable across sectors, thereby enhancing its relevance.
In summary, the future of data science will likely be characterized by increasing automation and accessibility, qualities embodied by platforms like DataRobot. Embracing these trends can lead to significant advantages for organizations willing to invest in expanding their data capabilities.
"Staying ahead in data science means understanding where technology is headed and aligning your skills accordingly."
As the evolution of machine learning platforms continues, professionals should remain vigilant about these emerging trends to leverage their potential for developing effective data strategies.
Ending
Summarizing Key Points
DataRobot stands out due to its user-friendly demo features and robust functionalities. Key points include:
- User Interface: The platform's design enables quick navigation, fostering an efficient user experience.
- Automation: DataRobot automates key processes in machine learning, streamlining workflows and reducing the time from data procurement to model deployment.
- Integration Capabilities: The easy integration with various data sources simplifies data management for users.
- Real-World Applications: Real-life use cases from industries such as finance and healthcare illustrate the platform’s versatility.
Final Thoughts on the DataRobot Demo
In final thoughts, the DataRobot demo serves as a vital tool for prospective users. It not only showcases the functionalities of the platform but also enhances understanding of how DataRobot can adapt to specific business needs. The demo provides a firsthand look at the efficiency of automated machine learning, fostering confidence in the product.
By engaging with the demo, users can make informed decisions regarding adoption and implementation. As machine learning continues to evolve, familiarizing oneself with platforms like DataRobot is increasingly beneficial. Therefore, attending a demo session can lead to a greater grip on harnessing data for imminent business advantages.