Harnessing DataRobot AutoML for Advanced Analytics
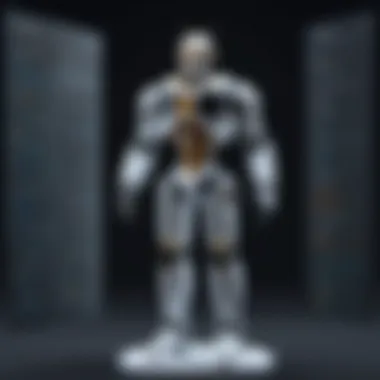
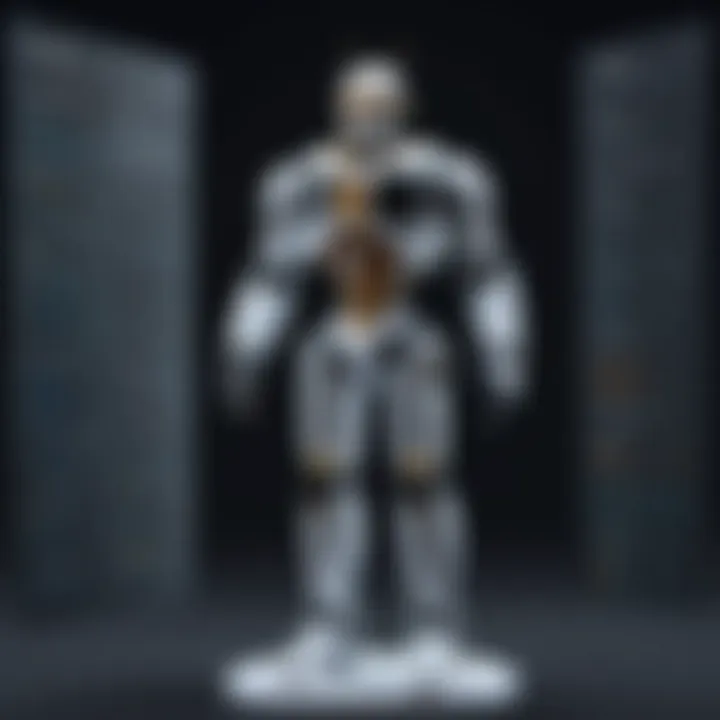
Intro
In the ever-evolving landscape of machine learning, the demand for tools that simplify complex processes is skyrocketing. DataRobot's AutoML platform emerges as a noteworthy player, designed to democratize and streamline the machine learning journey. This section sets the stage for delving into the platform's core features and capabilities, shedding light on what makes it indispensable for both seasoned professionals and budding data enthusiasts alike.
This article aims to provide you with a clear roadmap of DataRobot's functionalities, including its robust user interface and the seamless experience it offers across various applications. Whether you're a software developer looking to enhance your predictive analytics skills or a student exploring the possibilities of machine learning, understanding DataRobot's AutoML potential could be a game changer.
Let’s take a closer look at the features that make DataRobot AutoML a standout choice in a crowded marketplace.
Prolusion to DataRobot AutoML
As technology rapidly evolves, the significance of efficient and powerful tools in the realm of data science cannot be overstated. DataRobot's AutoML platform stands at the forefront of this movement, simplifying automated machine learning processes and making it accessible to a wider audience. Understanding what DataRobot offers is crucial for anyone interested in harnessing the power of machine learning, be they seasoned professionals or inquisitive students.
Understanding AutoML Concept
AutoML, or Automated Machine Learning, is not just a passing trend; it represents a paradigm shift in how machine learning is approached. Traditionally, developing a machine learning model has required considerable expertise in data science, coding, and statistical analysis. AutoML tools like DataRobot change the game by automating many of the intricate and time-consuming tasks typically required.
In essence, the concept revolves around making machine learning tangible for non-experts while enhancing the productivity of seasoned practitioners. AutoML orchestrates the processes of data cleaning, model selection, and hyperparameter tuning, thereby streamlining the modeling pipeline considerably. By enabling a more hands-off approach, users can focus on insights and applications rather than getting bogged down by the nitty-gritty details of the algorithms.
"AutoML enables users to deploy machine learning models with unprecedented ease and speed, spurring innovations across sectors."
The Evolution of Machine Learning Platforms
Looking back, the evolution of machine learning platforms has been nothing short of revolutionary. Early systems demanded domain knowledge and programming proficiency, causing barrier for entry for many potential users. As machine learning applications burgeoned in industries from finance to healthcare, the need for more accessible and user-friendly solutions became abundantly clear.
Enter DataRobot, among others, which began to reshape the landscape. By leveraging the power of cloud computing and innovative algorithms, DataRobot transformed complex machine learning processes into straightforward automated tasks.
Some key milestones in this evolution include:
- Introduction of specialized libraries: Frameworks like TensorFlow and Scikit-learn made machine learning more approachable but still required solid programming skills.
- Rise of GUI-based solutions: Tools that focus on graphical interfaces have helped decrease the learning curve, but many still didn't reach full automation.
- Integration of AutoML: With platforms like DataRobot, a completely automated approach emerged, democratizing access and application of machine learning techniques.
The culmination of these advancements has led to a scenario where machine learning capabilities are not just confined to data scientists but can be leveraged by stakeholders across various sectors, from business analysts to healthcare professionals.
In summary, understanding DataRobot AutoML and its context is vital for grasping the future of machine learning. As we move forward, efficiency, accessibility, and innovative applications will continue to redefine what is possible with data and automated systems.
Key Features of DataRobot
DataRobot is a robust platform designed to optimize the efficiencies of automated machine learning. It stands out among other tools by offering features that significantly enhance productivity and accuracy in modeling processes. Understanding these key features is paramount for developers, IT professionals, and students alike, as they highlight potential benefits, limitations, and important considerations involved in deploying DataRobot in their work.
Automated Model Building
One of the crown jewels of DataRobot is its automated model building. This sophisticated feature harnesses the power of machine learning algorithms to streamline the model creation process. Rather than slogging through manual coding and trial and error, users can quickly generate multiple models with just a few clicks. This efficiency not only saves time but also allows users to focus more on interpreting results rather than being bogged down by the mechanics of model creation.
For instance, a data scientist looking to predict customer churn can input the dataset and let DataRobot test numerous algorithms simultaneously. The platform ranks these models based on performance metrics, providing clear insights into which approach yields the best results. Users can easily deploy their preferred model into production, ensuring a seamless transition from theory to application.
Data Preparation Tools
Data often comes in a rough state needing polishing before it can be effectively analyzed. DataRobot addresses this challenge head-on with its comprehensive data preparation tools. This feature enables users to efficiently cleanse, transform, and enrich their data, ensuring high quality for their machine learning processes.
For instance, if working with customer purchase history, one might encounter missing values, outliers, or inconsistencies. DataRobot includes functions to handle these issues, automating tasks such as imputation or normalization. This means users can allocate less time on pre-processing and more on generating insights from their data.
Additionally, preparatory tools enable users to visualize data distributions, assess feature importance, and derive meaningful features that contribute to their predictive models. This holistic approach to data management not only sharpens accuracy, but also enhances the overall robustness of analysis performed.
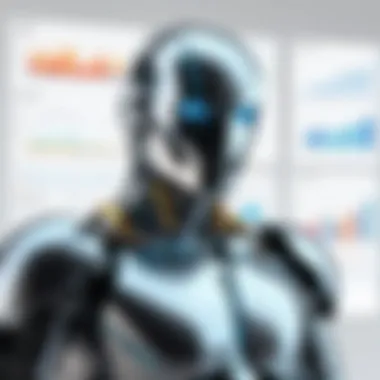
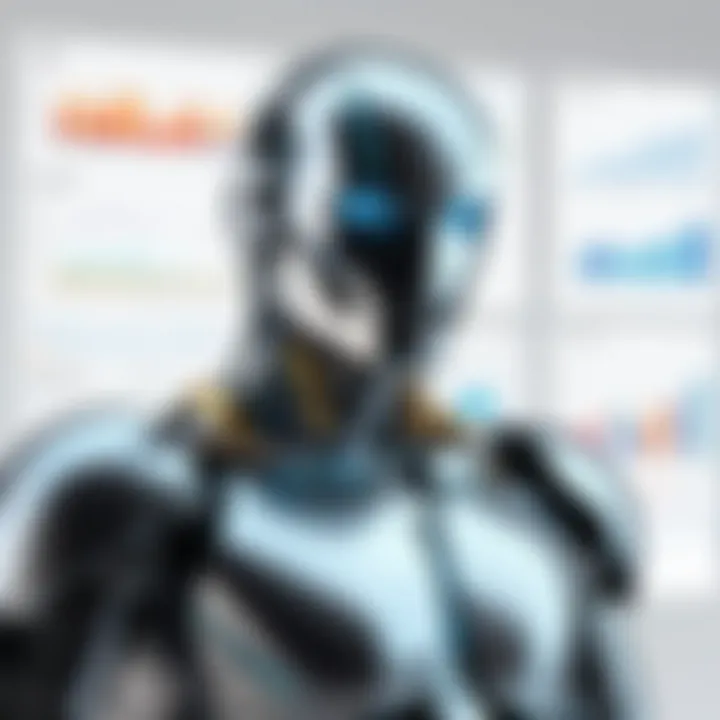
Advanced Analytics Capabilities
DataRobot's advanced analytics capabilities further distinguish it from traditional machine learning frameworks. The platform enables predictive modeling that incorporates ensemble learning techniques, boosting both accuracy and reliability.
The advent of advanced analytics means that users can forecast trends and behaviors with higher confidence. For example, in a healthcare setting, models predicting patient outcomes can incorporate a variety of datasets—ranging from genetic information to environmental factors—creating a multidimensional view of health predictions. Better models correlate with better results, ultimately saving lives.
Moreover, DataRobot offers interpretable outcomes, an often overlooked but crucial element of model development. Users can access explanations for predictions and ascertain how input features influence outputs. This interpretability is vital in industries like finance or healthcare, where understanding model decisions can directly impact regulatory compliance and ethical considerations.
In summary, the amalgamation of automated model building, effective data preparation tools, and advanced analytics capabilities in DataRobot makes it an essential platform for anyone looking to leverage machine learning in real-world applications. These features empower users to achieve results swiftly and accurately while encouraging a deeper understanding of the underlying processes.
Benefits of Using DataRobot AutoML
DataRobot AutoML is all about the perks it brings to the table. With the growing demand for machine learning solutions, understanding how this tool streamlines the process is pivotal. Businesses and individuals alike stand to gain a lot from employing this platform. The discussions here focus on three main benefits: efficiency in model training, predictive accuracy, and accessibility for those who aren't experts in data science.
Efficiency in Model Training
When it comes to model training, time is often of the essence. DataRobot excels by automating tasks that typically require a lot of manual input. This allows data scientists and machine learning engineers to focus more on strategic decisions rather than getting bogged down in repetitive processes. The platform effortlessly handles the intricacies of feature selection and hyperparameter tuning. This means quicker iterations and more opportunities for fine-tuning the models.
For instance, consider a retail company aiming to predict customer purchasing behavior. Traditionally, this would involve a lengthy process from data preparation to model deployment. With DataRobot, the company can jump straight into training various models without needing detailed knowledge of every algorithm. In turn, this drastically reduces the time from the initial data analysis to actionable insights.
In summary, efficiency in model training is one of the cornerstones of DataRobot's AutoML capabilities, making projects run smoother and faster.
Enhanced Predictive Accuracy
Let’s talk about accuracy—something every organization aims for in predictive analytics. DataRobot AutoML leverages advanced algorithms, allowing it to generate models that are often more accurate than those developed manually. The platform systematically evaluates thousands of algorithms and their configurations, which greatly enhances the odds of finding an optimal solution for any given problem.
Take the healthcare industry as an example. Employing DataRobot, a hospital can predict patient admissions more accurately. This could aid in resource allocation, staffing, and even patient care strategies. The implications here are significant, as enhanced accuracy leads to more precise decision-making and potentially better patient outcomes.
In short, the enhanced predictive accuracy offered by DataRobot is an undeniable strength, enabling organizations to make informed decisions based on data-driven projections.
Accessibility for Non-Experts
Last but certainly not least is accessibility. Many organizations possess valuable data but lack the expertise to deploy sophisticated machine learning models. DataRobot AutoML takes that barrier down. The intuitive interface is designed to guide users through the process without requiring them to be math whizzes. With clearly defined steps and smart assistance, individuals can jump into the world of machine learning without prior experience.
Imagine a small business owner wanting to optimize their marketing campaigns but unsure of where to begin. DataRobot provides them with easy-to-follow instructions to upload their data, choose a model, and interpret the results. This democratization of machine learning is vital, as it empowers anyone with interest and relevant data to harness the power of analytics.
"By making machine learning accessible to the masses, DataRobot is fundamentally changing how organizations operate and strategize."
The accessibility provided by DataRobot AutoML opens doors that had long been closed, allowing a wider audience to engage with data analytics directly.
Practical Applications of DataRobot AutoML
When we talk about the real-world implications of AutoML, DataRobot certainly takes center stage. The platform's practical applications make it quite indispensable in today's data-driven environment. Companies across various sectors are diving headfirst into automated machine learning, aiming to capitalize on efficiency, accuracy, and insights without needing an army of data scientists. Let's explore four key areas where DataRobot is making a significant impact, specifically highlighting its tailored use cases.
Use Cases in Business Intelligence
In the realm of business intelligence, DataRobot AutoML stands as a beacon of hope for organizations looking to make data-driven decisions quicker and more reliably. For instance, consider a retail chain that needs to predict sales for the upcoming quarter. By rapidly deploying DataRobot, they can sift through historical sales transactions, marketing expenditures, and seasonal trends to build predictive models. This can result in optimized stock levels, effective promotional strategies, and even personalized marketing efforts based on consumer buying patterns.
Moreover, its intuitive interface allows users to drag and drop data, simplifying what would usually be a technical task. This kind of accessibility equips business analysts without deep technical know-how to harness complex algorithms, ensuring that insights are not trapped within the confines of the IT department.
Applications in Healthcare
AutoML’s potential in the healthcare field is nothing short of revolutionary. Imagine hospitals using DataRobot to streamline their patient admission process. By analyzing past patient data, including length of stay, diagnosis, and treatment, healthcare providers can predict bottlenecks and optimize bed occupancy rates.
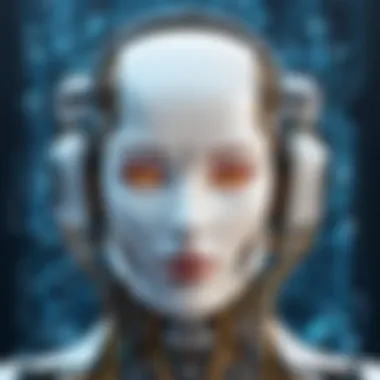
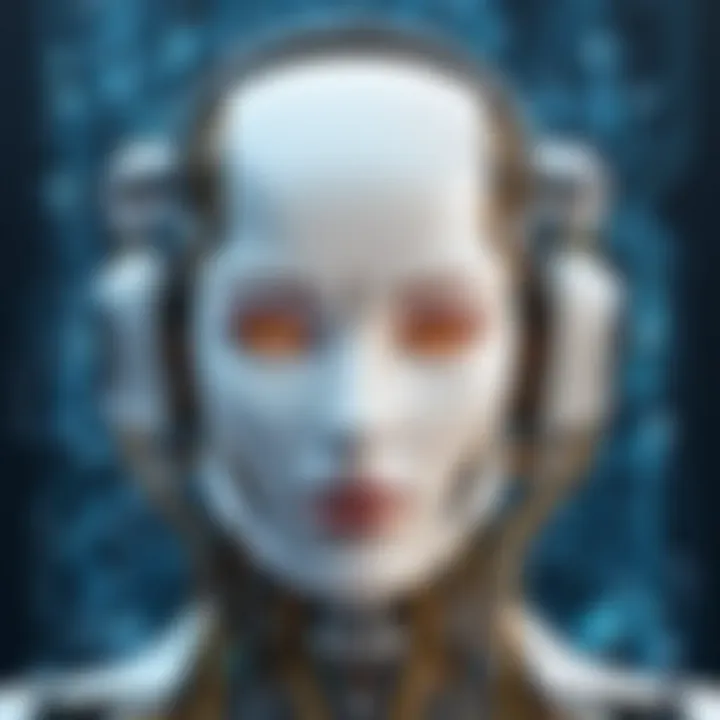
For example, a health organization could implement this technology to forecast patient inflow based on external factors like flu season. Such insights not only enhance operational efficiency but also significantly improve patient care by ensuring that resources are deployed where they’re needed most. Furthermore, DataRobot’s models can assist in identifying potential disease outbreaks, underpinning preventive measures and public health strategies.
Financial Services and Risk Assessment
In finance, the stakes are high, and the margin for error is low. DataRobot AutoML provides a solid framework for risk assessment—think about credit scoring and fraud detection. Banks can analyze vast datasets that include transaction histories, credit scores, and demographic factors to develop models that assess creditworthiness with greater precision.
For instance, a credit analyst in a financial institution might utilize DataRobot to detect irregular patterns in transaction data that could signify fraud. This not only saves time but also allows institutions to adapt swiftly to potential threats, mitigating risks that could lead to significant financial losses. The automation of such models not only shortens the decision-making time but also keeps compliance risks at bay.
Impact on Marketing Strategies
Marketers are increasingly embracing DataRobot for its ability to enhance campaign effectiveness. The platform enables companies to leverage customer data to craft more targeted marketing strategies. By predicting customer segmentation and behavior, companies can tailor their outreach efforts to resonate with individual preferences.
Consider an e-commerce store that deploys DataRobot to analyze user browsing patterns and previous purchases. By segmenting their customer base and predicting likely future purchases, they can deliver personalized recommendations, vastly improving conversion rates. This is more than just feeling out potential buyers; it’s about using data to drive meaningful interactions that lead to higher sales and customer satisfaction.
In summary, the practical applications of DataRobot AutoML transcend sectors, showcasing its versatility and practical relevance in decision-making. By delivering precision and efficiency, it enables companies from all walks of life to harness the power of data, ultimately driving innovation and growth.
Technical Architecture of DataRobot AutoML
Understanding the technical architecture of DataRobot AutoML is crucial as it unveils the underlying mechanisms that support automated machine learning functionality. This framework is what allows organizations to harness big data and derive actionable insights efficiently.
Integration with Existing Data Sources
One of the standout aspects of DataRobot is its seamless integration capabilities. Organizations often have vast volumes of data stored across various systems – databases, cloud storage, or even on-premise solutions. Recognizing this, DataRobot is equipped to connect with these diverse data sources without a hitch.
By enabling connections to major databases like MySQL, PostgreSQL, and Oracle, as well as cloud platforms such as Amazon S3 and Azure Blob Storage, it simplifies the process of data ingestion. This means users can focus on analyzing their data rather than wrestling with the logistics of bringing it into the AutoML pipeline. Here's why this integration matters:
- Flexibility: Users can work with multiple data types without any need for manual data preparation.
- Time-Saving: Automating the process of data fetching cuts down project lead times significantly.
- Consistency: It ensures that data is pulled directly from the source, reducing the risk of errors associated with manual data handling.
"The ability to integrate data effortlessly is not just an efficiency lever; it’s a major competitive advantage!"
Scalability and Cloud Deployment
In today’s fast-evolving data landscape, scalability is essential. DataRobot's architecture is thoughtfully designed to support this need. As data volumes explode and analytical demands increase, having a solution that can scale elastically is non-negotiable. DataRobot’s deployment options significantly contribute to this scalability.
Deploying via cloud infrastructure provides several benefits:
- On-Demand Resources: Users can tap into computational resources as needed, allowing them to handle large datasets without upfront investments in hardware.
- Cost Efficiency: Companies pay only for what they use, making it a prudent financial choice for many businesses.
- Global Reach: Cloud deployment enables access to resources globally, which is vital for companies with distributed teams.
In addition to cloud flexibility, DataRobot supports various deployment models such as public, private, or hybrid clouds, giving users added control over their data. This flexibility caters to different organizational needs, from those pursuing complete control over their infrastructure to those preferring a hands-off, all-inclusive approach.
When coupled with DataRobot’s robust APIs, organizations can easily incorporate machine learning capabilities into their existing applications, thus unlocking new business possibilities without heavy lifting.
With these elements of technical architecture firmly in place, users can appreciate how DataRobot AutoML effectively facilitates complex machine learning tasks, all while simplifying the user experience.
Challenges and Limitations
Understanding the challenges and limitations of DataRobot's AutoML is crucial for both seasoned practitioners and novices. As automated machine learning garners more attention for its potential to streamline processes, it also comes with its own set of hurdles that can impact effective implementation. Acknowledging these challenges not only provides a realistic perspective but also equips users with tools to navigate issues that may arise during deployment.
Data Quality Considerations
Data quality is paramount. In the realm of machine learning, if the input data isn’t up to snuff, the models will generally follow suit—garbage in, garbage out, right? DataRobot can crank out automated models at lightning speed, but the effectiveness of these models hinges on the integrity of the data fed into them. Poor data can lead to misleading results, skewed predictions, and ultimately, erroneous conclusions that can derail business strategies.
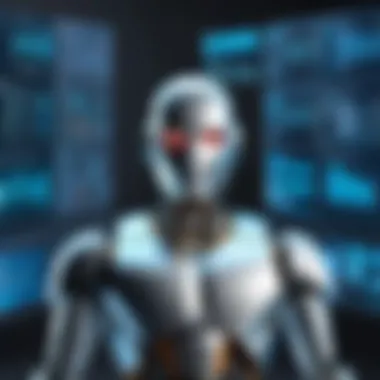
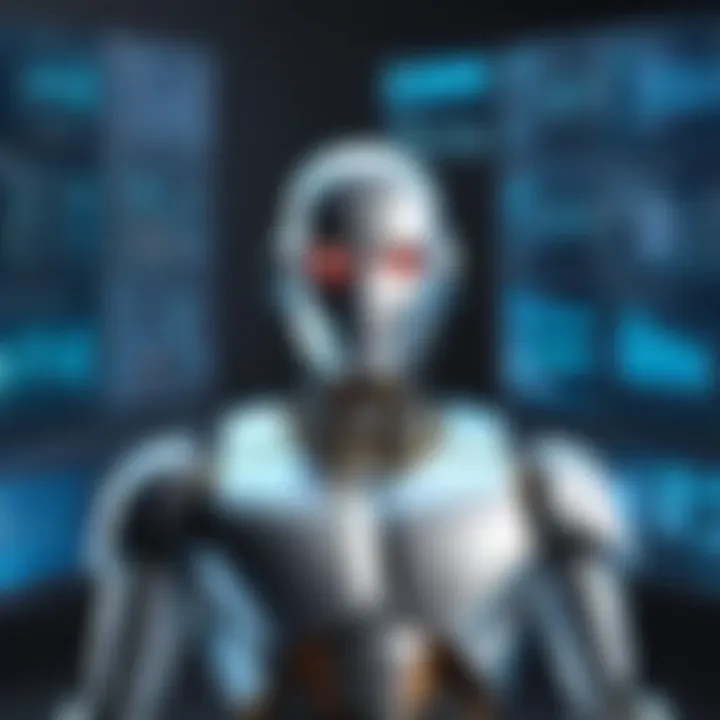
- Data Completeness: Models require comprehensive datasets. Missing values can distort training processes, causing models to perform poorly in a production environment.
- Relevance of Data: Using outdated or irrelevant data can similarly mislead the model, as it may not align with current market dynamics or organizational needs.
- Bias in Data: Bias in the dataset can lead to biased models. This is particularly concerning in sensitive areas like healthcare or finance, where biased predictions can have serious repercussions.
To mitigate these issues, users must actively engage in data validation and thorough preprocessing before utilizing DataRobot AutoML for model building. It’s wise to adopt a mindset of continual data governance—because the world is always changing, and so should your data.
Interpretability of Automated Models
A significant hurdle often faced with AutoML solutions is model interpretability. While DataRobot uses cutting-edge algorithms that can offer high levels of accuracy, understanding how these algorithms arrive at their conclusions can be elusive.
- Complex Algorithms: As machine learning models grow more sophisticated, their internal workings often transform into a ‘black box.’ This can make it difficult for users to ascertain the rationale behind predictions, potentially hindering trust in the automation process.
- Risk Management: In sectors like finance or healthcare, stakeholders need to understand decision-making processes. If a model flags a loan application as risky without a clear explanation, for instance, it may stall business decisions or result in disputes.
- Ethical Considerations: The lack of interpretability can lead to ethical challenges. Organizations must navigate the fine line between powerful predictive analytics and the accountability for outcomes influenced by these automated decisions.
To tackle these challenges, organizations using DataRobot can invest in tools and approaches that foster transparency. For instance, leveraging SHAP (SHapley Additive exPlanations) values can help elucidate how different features contribute to predictions, making strides towards more understandable, actionable insights.
Understanding and addressing these challenges empowers professionals to harness DataRobot AutoML’s capabilities responsibly and effectively.
Future Trends in AutoML
The landscape of automated machine learning, or AutoML, is evolving at a pace that can be quite astonishing to those involved in the field. As industries begin to embrace this technology, it’s important to take note of where things are headed. Understanding these future trends can greatly influence strategic decisions within organizations, pushing the boundaries of what can be accomplished through machine learning.
Advancements in AI-Driven Automation
In the realm of AutoML, one of the most significant trends is the continuous improvement of AI-driven automation techniques. This covers a broad spectrum of elements, from preprocessing data to model selection and hyperparameter tuning. With machine learning algorithms becoming smarter, they are increasingly capable of handling complicated data transformations on their own. This not only saves time but also reduces human error, leading to more reliable outcomes.
Recent advancements, such as the introduction of neural architecture search (NAS) and automated feature engineering, have placed AI automation at the forefront. For instance, NAS helps determine the best model architecture for specific datasets, leaving the intricate decision-making to the algorithms while practitioners can focus on overarching strategies. Additionally, some platforms now offer meta-learning capabilities, which guide the system to learn from past models.
The potential here is enormous. As tools become more capable of handling greater complexities without human intervention, businesses can expect to experience shorter development cycles and, ultimately, a quicker return on investment.
By embracing these advancements, organizations can utilize machine learning models that not only work faster but also yield better predictive results, propelling industries into a new era of data-driven decision-making.
Predictions for Industry Adoption
Looking forward, the adoption of AutoML technologies across various industries seems like a foregone conclusion. The need for timely insights and predictions has never been greater, leading many to consider how these tools can streamline their processes.
- Increased Accessibility for Businesses: Many companies are beginning to realize the importance of data-driven decision-making. Small and medium enterprises, which might have previously felt overwhelmed by resource constraints, can now leverage AutoML tools to implement analytics solutions that were once the domain of large corporations. This democratization of technology signifies a fundamental shift in the landscape.
- Integration with Enterprise Systems: Another likely outcome is the deeper integration of AutoML solutions within existing enterprise software ecosystems. Firms are increasingly seeking seamless workflows. Therefore, connecting AutoML capabilities with customer relationship management (CRM) systems or enterprise resource planning (ERP) software gives users a comprehensive toolkit that enhances productivity and insight.
- Focus on Specific Industry Solutions: Future trends will also likely reveal a surge in solutions tailored for specific industries. For example, sectors like healthcare and finance may see bespoke AutoML platforms that adhere strictly to regulatory standards while driving innovation. Businesses will be looking for solutions that fit their particular needs, and vendors who provide these specialized tools will gain a competitive edge.
"The future of AutoML isn’t just about making machine learning easier; it's about unlocking its full potential across various sectors."
As AutoML continues to develop, its capacity to transform industries is set to grow. These advancements could redefine how businesses approach data, making it an even more critical component of strategic initiatives.
Embracing these changes enables organizations to prepare for the shifts, ensuring that they remain competitive in an increasingly data-driven world.
End
In summarizing the explorations into DataRobot AutoML, a clearer picture emerges regarding the potential of automated machine learning. The significance of this topic extends beyond simple enhancements to predictive analytics. It importantly touches on how businesses, educational institutions, and professionals can adapt and thrive amid growing data complexities. Automated Machine Learning, with its repeated processes and minimal manual interference, addresses the growing demand for faster insights, efficiency, and scalability.
Summarizing the Potential of AutoML
The strengths of AutoML are many, including:
- Speed: DataRobot allows for the rapid intake and processing of data, dramatically reducing the time between data acquisition and actionable insight.
- Versatility: Whether in healthcare harnessing patient data or in retail analyzing purchasing patterns, AutoML adapts to various sectors, revealing opportunities that manual analysis might overlook.
- Empowerment of Non-Experts: With its user-friendly interface and guided workflows, DataRobot democratizes access to advanced analytics, allowing those without a technical background to contribute towards data-driven decisions.
Moreover, the comprehensive capabilities within DataRobot—spanning model building, data prep, and analytics—demonstrate how organizations can capitalize on machine learning without needing extensive prior knowledge. It's like getting a sports car with automatic features; you enjoy speed and performance without becoming a mechanic first.
"DataRobot AutoML transforms the complexities of machine learning into manageable tasks, enabling broad participation in data analytics initiatives."
The Path Forward for DataRobot Users
As organizations continue to adopt AutoML systems like DataRobot, several considerations lay ahead for its users:
- Continuous Learning: Staying updated with the latest developments in machine learning is pivotal. As technologies evolve, new features and methodologies can significantly alter how organizations leverage their data.
- Integration Needs: Users must ensure that AutoML tools integrate seamlessly with existing workflows and data sources. Pay attention to compatibility with databases or other analytics tools.
- Ethical Considerations: With great power comes great responsibility. Users should be cautious about biases in data and the potential consequences of their model predictions. Establishing guidelines on ethics in AI use will be crucial, especially in sensitive industries.