SQL's Critical Role in Managing Big Data Challenges
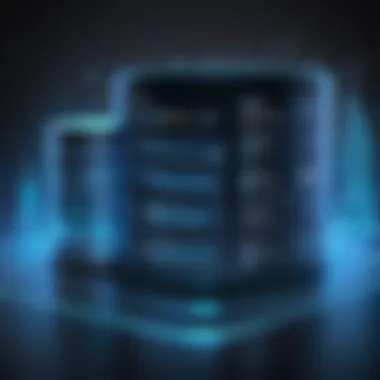
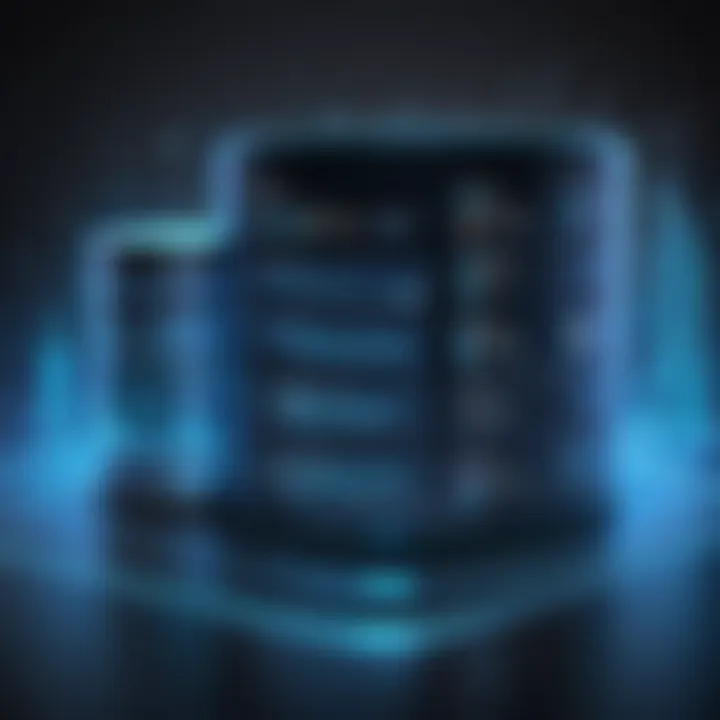
Intro
As organizations dive headfirst into the world of big data, the significance of SQL cannot be overstressed. SQL, short for Structured Query Language, has long stood as a stalwart in the realm of data management. With its rich set of capabilities, it serves as both a bridge and a catalyst, enabling data professionals to extract insights from massive datasets with relative ease.
In our discussion, we'll unravel the multifaceted role SQL plays in the intricate landscape of big data. From its core features that provide structure to data manipulation, to performance considerations that directly impact the efficiency of data processes, SQL remains a critical enabler. We will also explore the integration of modern technologies, such as cloud computing, that reshape how SQL is applied in today’s data-rich environments.
Moreover, understanding the limitations of traditional SQL in big data scenarios is essential. Despite its robust functionality, SQL faces challenges in handling the sheer volume, velocity, and variety typical in big data contexts. Yet, ongoing innovations are breathing new life into SQL's potential, paving the way for its evolution.
In this article, you will find insights valuable to both the beginner and the seasoned developer. Whether you are a software expert looking to refine your skills or a student aiming to comprehend the dynamics of SQL in big data, our exploration will provide you with a wealth of information. We will guide you through key features, performance metrics, and much more, equipping you with the knowledge to navigate the complex waters of big data using SQL.
Understanding Big Data
In this age of information, big data stands as a cornerstone in numerous sectors. It allows organizations to glean insights from vast volumes of information that were previously inconceivable to analyze. Understanding big data involves recognizing both its complexities and its potential to transform decision-making processes. The ability to harness big data provides businesses with the power to anticipate trends, personalize services, and drive efficiency.
Big data is not merely about size; it encompasses various factors such as velocity, variety, and volume. Each of these elements plays a crucial role in how data is gathered, processed, and utilized. Taking a closer look at these characteristics can elucidate the transformative effects big data could have on operations and strategy within an organization.
Definition and Characteristics
Big data generally refers to datasets that are so large or complex that traditional data processing applications are inadequate to handle them. The prominent characteristics of big data can be encapsulated in the three Vs: Volume, Velocity, and Variety.
- Volume indicates the vast amounts of data generated every second; it can come from various sources like social media, transaction records, and sensors.
- Velocity represents the speed at which this data flows in and out, necessitating real-time processing to derive actionable insights promptly.
- Variety captures the different formats of data – structured and unstructured – that require diverse tools and strategies for analysis.
These elements intertwine, creating a need for advanced methodologies and technologies to manage and analyze data effectively.
Sources of Big Data
Understanding the origin of big data can significantly enhance clarity about its potential value and applications. The following sources contribute to the growing reservoir of data in today’s world:
Social Media
Social media forms a crucial element of big data that reflects user behavior, preferences, and interactions. Platforms like Facebook and Twitter generate immense data streams through posts, comments, and likes, making them rich sources for analysis. The real-time interactions provide insights into public sentiment and trends, making social media a powerful resource for businesses looking to enhance customer engagement and brand loyalty.
However, while the volume of data is vast, its unstructured nature can pose challenges in terms of deriving clear, usable insights.
IoT Devices
The rise of the Internet of Things (IoT) has opened another floodgate of data collection. Devices from smart home appliances to wearable technology contribute to continuous data streams. Each of these IoT devices collects and transmits data about usage patterns and performance. This constant influx enables businesses to identify patterns and optimize services and products.
Yet, with multiple devices collecting data, issues related to data privacy and interoperability may arise, creating roadblocks in effectively leveraging this data.
Enterprise Systems
Enterprise systems, like Customer Relationship Management (CRM) and Enterprise Resource Planning (ERP), aggregate business data across various functions. They play an essential role in managing organizational processes, allowing companies to make informed strategic decisions based on comprehensive insights. The structured data from these systems can yield high-quality, reliable insights that are key to operational improvements and strategic growth.
However, integrating data from multiple enterprise systems may be complex, requiring substantial resources and strategic alignment.
Importance of Big Data
The importance of big data lies in its ability to drive data-driven decision-making and offer profound insights into market trends. In today’s competitive environment, leveraging big data has evolved from a luxury to a necessity for businesses aiming to thrive.
Data-Driven Decision Making
Data-driven decision-making is pivotal in contemporary businesses. By utilizing data analytics, organizations can refine strategies and reduce risks associated with guesswork. This approach fosters a culture of evidence-based decision-making that amplifies effectiveness and efficiency across all levels. Organizations that adopt this practice tend to enjoy a competitive edge, as decisions grounded in real data are less prone to bias.
Although this strategy has numerous advantages, one must be cautious about the potential for analysis paralysis, where the abundance of data becomes overwhelming, causing slow decisions.
Market Trends Analysis
Analyzing market trends is another critical outcome of big data utilization. Companies can track fluctuations, consumer behavior, and competitive activities through integrated data sets. By recognizing patterns, organizations can adjust their offerings in real-time, staying ahead of the curve.
However, interpreting these trends correctly is an art and science combined; misinterpretation can lead to misguided strategies, making proper analysis imperative.
"Big data is not just about the data; it’s about what you do with that data that counts."
Through extensive examination of big data’s characteristics, sources, and significance, one can appreciate the complex yet rewarding journey that lies ahead. SQL, as explored in this article, serves as a linchpin in navigating this landscape, enabling effective data manipulation and analysis in an era dominated by big data.
SQL Fundamentals
When diving into the realm of big data, understanding the fundamentals of SQL is not merely an option; it’s a necessity. SQL, standing for Structured Query Language, serves as a bridge between the user and the massive datasets that define the big data landscape. It equips analysts, developers, and data scientists with the tools needed to manipulate, retrieve, and analyze data effectively. This section lays the groundwork for grasping how SQL operates within big data environments, ensuring readers grasp its significance in effective data management.
Overview of SQL
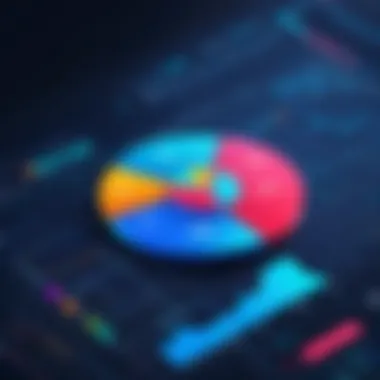
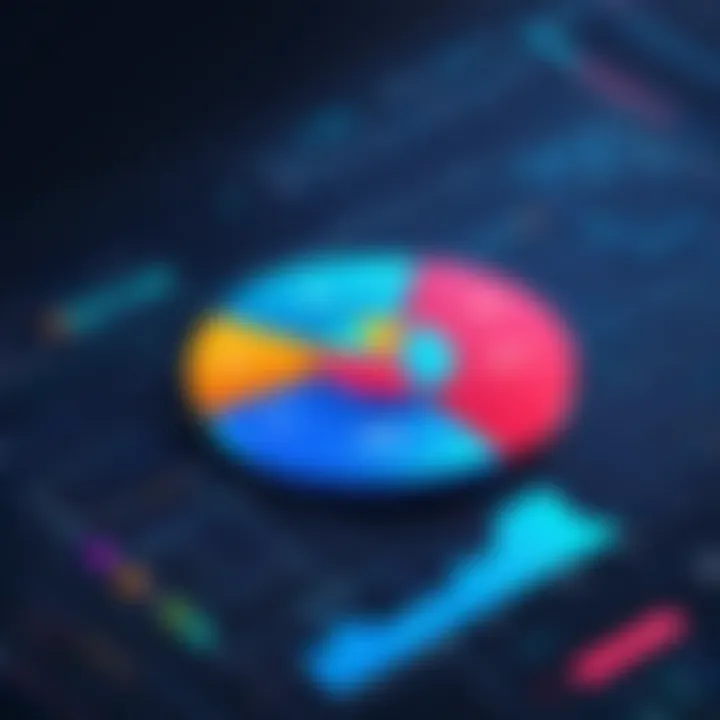
SQL is the backbone of data operations in relational database management. Rooted in the principles of set theory, SQL provides commands that enable users to perform various manipulations on data stored in structured formats. It allows for querying information, updating content, and managing database schemas, ultimately providing a systematic way to interact with large volumes of data reliably. Moreover, SQL has evolved, gaining compatibility with big data frameworks, enabling users to tap into the potential of technologies like Hadoop and Apache Spark.
SQL Data Types
Numeric Types
Numeric types in SQL play a fundamental role in storing any data that is quantitative in nature. This includes integers, decimals, and floats. The choice to use numeric types is often driven by the need for accuracy and performance in calculations. Notably, these types allow for precise arithmetic without compromising on storage efficiency, making them a preferred choice when dealing with monetary values or scientific calculations. A unique characteristic of numeric types is their ability to define precision and scale, crucial for applications in financial databases. However, improper management of these types may lead to issues such as overflow errors in constraints of data size.
Character Types
Character types encompass data that represent strings of text within a database. These can range from single characters to large blocks of text. The flexibility provided by character types makes them essential for applications involving text data, be it user names, addresses, or any other textual information. A key characteristic of character types is their capacity to store varied lengths of data (like VARCHAR). This adaptability can save storage space where fixed-length types would be wasteful. On the flip side, performance can suffer if overly large character types are misused, leading to slower processing times during searches or filters.
Date and Time Types
Date and time types in SQL are vital for applications requiring time-based analysis, such as logging events or tracking transactions. These types allow for storing information about when specific actions occurred, which, in turn, supports trend analysis and reporting. A distinctive feature is their ability to support a wide range of operations, from simple fetches to complex querying involving time zones and intervals. Their utility is evident in business intelligence scenarios where understanding the timing of events can provide competitive insights. However, improper handling, such as ignoring time zones, may result in mismatched data, leading to erroneous interpretations.
Basic SQL Commands
Each SQL command serves a unique purpose and functionalities that facilitate data handling.
SELECT
The SELECT command is perhaps the most crucial piece in SQL's puzzle. It allows users to retrieve data from a database effectively. What makes SELECT particularly powerful is the ability to filter, sort, and group results, making it a highly flexible option for extracting meaningful insights from large datasets. Its adaptability in specifying distinct records versus duplicates can streamline analysis processes. However, users must be careful about performance impacts when selecting from enormous tables without filtering criteria, leading to unnecessary delays.
INSERT
The INSERT command is foundational for populating databases with new data. This command enables users to add single or multiple records in one go, which is especially efficient in bulk operations. A notable aspect of INSERT is the flexibility it offers in specifying values for individual fields, or using a SELECT statement to fill the new records, enhancing flexibility. However, users might encounter scenario where adding too many records at once could lead to performance bottlenecks, causing delays in database interactions.
UPDATE
UPDATES are essential for modifying existing records in a database. The strength of the UPDATE command lies in its ability to change values based on specific conditions, ensuring only targeted records are altered. This precision is key in scenarios like correcting data or adjusting values based on new information. However, the potential danger lies in running an UPDATE without proper WHERE clauses, leading to unintended mass updates.
DELETE
Lastly, the DELETE command is central to maintaining data integrity by removing records no longer needed. Its primary feature is the conditional removal of data, which can help in data management and decluttering databases. A powerful aspect of DELETE is the ability to target specific records using WHERE clauses, but one has to tread carefully; accidental truncation can lead to significant data loss.
"In the ever-evolving world of big data, understanding SQL is akin to knowing the ropes in a vast sea—essential to navigate successfully."
Throughout this section on SQL fundamentals, we've scratched the surface of how SQL’s features empower users in big data environments. Each element, from data types to essential commands, weaves together to create a compelling narrative around effective data management and analysis.
SQL’s Evolution in Big Data
The landscape of data management has shifted remarkably with the exponential growth of data volume, variety, and velocity. SQL’s role has evolved from traditional relational databases to accommodate the new reality of big data. This evolution is crucial because it highlights how SQL adapts to emerging technologies and the need for swift data processing in vast and diverse datasets. Without this evolution, many organizations would struggle to harness the wealth of information that big data presents.
Traditional SQL vs. Big Data SQL
Traditional SQL was designed with structured data in mind, focusing primarily on tables and relationships. It boasts a powerful syntax for querying relational databases, making it a solid choice for many applications. However, the emergence of big data revealed some limitations. The inability of traditional SQL to handle unstructured data or perform real-time analytics effectively was apparent.
Big Data SQL solutions have started to address these shortcomings by introducing functionalities that allow processing of diverse data types. Real-time data queries, support for semi-structured data, and enhanced scalability are just a few examples. Ultimately, understanding the differences between traditional and big data SQL is essential. This allows us to appreciate how SQL has flexed its muscles to remain relevant in today's data-centric environment.
Integration with Big Data Technologies
Integrating SQL with big data technologies has allowed for a more holistic approach to data management and analysis. Two key players in this field are Hadoop and Apache Spark, both of which have their distinct roles and advantages.
Hadoop
Hadoop has revolutionized data storage and processing by enabling distributed computing. Its architecture allows for massive amounts of data to be stored across multiple machines, making it an indispensable tool for big data management. A standout characteristic of Hadoop is its scalability. Businesses can grow their storage capacity with ease, adding more nodes without undergoing significant changes to the underlying architecture.
One unique feature of Hadoop is the Hadoop Distributed File System (HDFS), which splits large files into blocks stored across a cluster. This not only improves access speeds but also ensures data redundancy. However, processing with Hadoop can be slower compared to other technologies, especially for complex queries, due to its batch processing nature.
Apache Spark
Apache Spark is gaining ground as a preferred choice for real-time data processing in big data environments. It surpasses Hadoop in speed, making it a more suitable option for complex queries. Spark operates in-memory, which significantly speeds up analytics, especially when dealing with large datasets. Its ability to run SQL queries (via Spark SQL) serves as a bridge for SQL users to transition into the big data realm.
One of the distinct benefits of Spark is its versatility. It can handle various data formats and supports both batch and stream processing, providing a comprehensive solution for analytics tasks. However, the complexity of setting up and managing a Spark cluster can be intimidating for newcomers.
SQL Engines for Big Data
The growth of SQL engines designed specifically for big data has been a game-changer. These engines provide the SQL familiarity to users while leveraging the power of big data architectures. Three notable SQL engines that deserve attention are Apache Drill, Presto, and Hive SQL.
Apache Drill
Apache Drill stands out due to its schema-free JSON data model, allowing users to query different types of data without first defining a schema. This flexibility makes it particularly useful for organizations dealing with diverse data formats. Drill's ability to perform ad-hoc queries across multiple sources helps streamline data discovery.
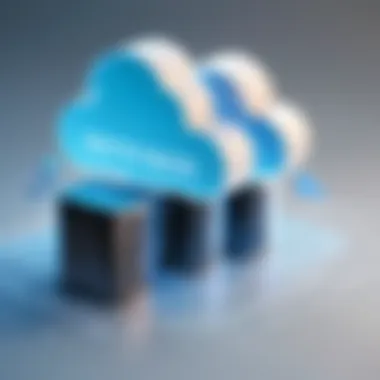
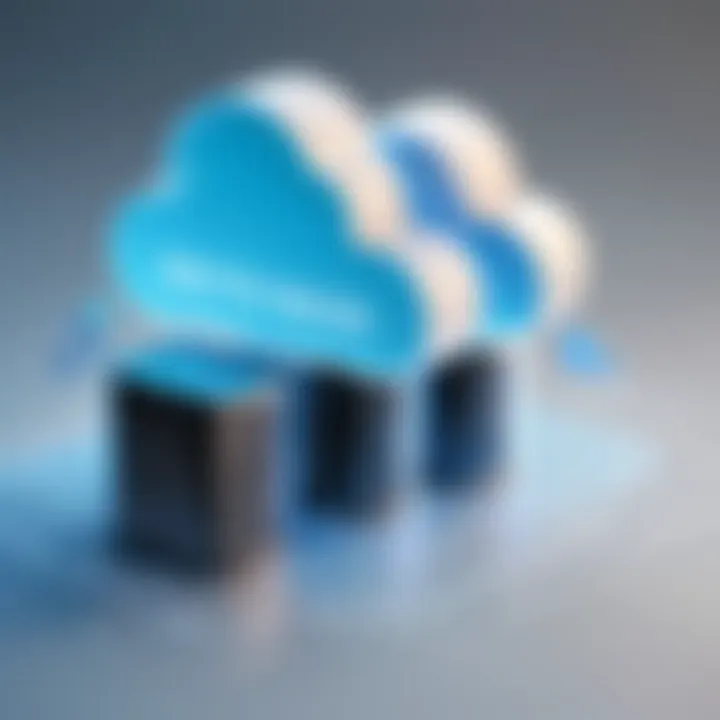
However, one downside of Drill is the potential for performance issues with very large datasets. Optimizing queries can become challenging, requiring careful planning and expertise.
Presto
Presto is tailored for high-performance queries across large datasets. Suitable for organizations needing quick access to Big Data resources, it enables users to perform queries on data residing in various sources, including HDFS and cloud storage. A key feature of Presto is its interactive query performance, which allows for real-time insights.
The downside? Presto can require substantial resources to maximize its performance. Organizations must ensure adequate support for optimal efficiency.
Hive SQL
Hive SQL was designed for big data by bringing SQL-like access to data stored in Hadoop. Its strength lies in handling large-scale data with a familiar syntax for SQL users. Hive translates its SQL queries into MapReduce jobs, making it scalable and suitable for batch processes.
One of the challenges with Hive is its slower performance compared to in-memory databases like Spark. Queries can take time since they rely on MapReduce, making it less ideal for operations needing rapid response times.
In summary, understanding the evolution of SQL in the big data ecosystem is key to navigating the complexities of today’s data environments. The interplay between SQL and big data technologies like Hadoop and Apache Spark, as well as the rise of dedicated SQL engines, shapes how data professionals approach analysis. As big data continues to flourish, SQL’s adaptability ensures it remains a vital tool for unlocking valuable insights.
Performance Optimization in SQL for Big Data
In the realm of big data, the sheer size and complexity of datasets can challenge traditional SQL approaches. Therefore, performance optimization emerges as a crucial facet that helps organizations sift through the vast oceans of data efficiently. By leveraging optimization strategies, SQL can manage larger datasets in a more effective manner, enabling users to derive valuable insights promptly and accurately.
The significance of optimizing SQL for big data environments cannot be overstated. As data volumes grow exponentially, organizations must ensure that their database queries execute swiftly. Slow responses can lead to missed opportunities and inefficient decision-making. Furthermore, performance enhancments can help in minimizing the resources consumed by these operations, thus saving costs without compromising on the data quality.
Indexing in Large Datasets
Indexing serves as a fundamental mechanism for improving query performance in large datasets. By creating an index, SQL databases can quickly locate specific rows without scanning the entire table, a process that can be extremely time-consuming. Think of indexing like the index of a book; instead of flipping through every page, you refer to the index to find the exact information you need.
However, indexing is a double-edged sword. While it accelerates read operations significantly, over-indexing can lead to slowdown in write operations. This is because every time a row is inserted or updated, the database needs to update the index as well. Therefore, striking a balance is essential when implementing indexing strategies in big data scenarios.
Query Optimization Techniques
Query optimization is the process of improving the performance of SQL queries to make them run as efficiently as possible. It plays an indispensable role in managing large datasets, given that poorly constructed queries can bog down even the most robust systems. Here are some techniques that come in handy:
Using Joins Efficiently
Using joins efficiently lies at the core of optimizing SQL queries. Joins combine rows from two or more tables based on a related column, making it a popular choice for retrieving related data across tables. The key characteristic of efficient joins is their ability to reduce the amount of data scanned. When used properly, joins can deliver powerful results without demanding excessive resources.
For instance, consider two tables: one for customers and another for orders. If you need to find all orders made by a specific customer, a well-structured join can yield the results by only accessing the rows from both tables that are necessary for your query, thus optimizing performance.
However, over-joining or using suboptimal join techniques may lead to "cartesian product" scenarios, where every row matches with every other row, leading to bloated datasets. Efficiency is about knowing how to apply these joins wisely, which is paramount in the context of SQL and big data.
Minimizing Data Scans
Minimizing data scans is another vital technique for optimizing performance. This entails tailoring queries to access only the data required, thereby reducing the computational load significantly. A common approach is using specific filtering conditions in the WHERE clause of a SQL statement. For example, pulling data from a sales table might only require records from the last quarter rather than retrieving the whole dataset.
The key characteristic of this approach is its focus on specificity. By refining queries to be as specific as possible, users reduce the number of unnecessary data scans which can slow performance. This also prevents unnecessary strain on database resources, maintaining optimal performance standards.
Moreover, fewer data scans mean quicker results, leading to much more effective analysis of data. However, care must be observed to ensure that filtering conditions don’t overlook potential essential data.
Partitioning Strategies
Partitioning strategies involve dividing large tables into smaller, more manageable segments. This can lead to significant performance gains, especially when working with vast datasets. Each partition can be queried independently, thus reducing the amount of data processed during a query execution.
For example, take a table that logs transactions over several years. By partitioning it based on the transaction date, the database can quickly access only the relevant year's data when querying, rather than sifting through records spanning multiple years.
However, designing appropriate partitioning strategies requires careful planning. Factors such as how the data is queried, the frequency of updates, and the overall structure of the datasets must be considered to avoid complications down the road.
SQL in Cloud-Based Big Data Solutions
In this digital age, the ability to manage large datasets efficiently is paramount for any business. SQL plays a critical role in cloud-based solutions when it comes to big data. It allows organizations to harness the power of vast data streams without being bogged down by traditional database limitations. Cloud-based SQL services provide an adaptable platform for storing, retrieving, and manipulating data, which is essential for making timely decisions in a fast-paced environment.
Benefits of Cloud SQL Services
- Scalability
Scalability is a standout feature of cloud SQL services. It refers to the system’s capability to handle a growing amount of work by adding resources smoothly. This allows organizations to increase their computing power and storage without needing hefty investments in physical infrastructure.
One major selling point of scalability in cloud systems is elastic provisioning. This means that as data loads fluctuate, resources can be adjusted automatically. Imagine trying to make the best of fleeting market opportunities; with SQL cloud solutions, you don’t have to lag behind because resources can be ramped up to meet demand when necessary.
However, don’t let the ease of scalability fool you into thinking there are no risks. Dependency on service providers for resources can leave businesses vulnerable to pricing fluctuations or downtimes, if not managed well.
- Cost-Effectiveness
The cost-effectiveness of cloud SQL services often catches the eye of decision-makers. Unlike traditional databases that require significant capital for setup and maintenance, cloud-based services operate on a pay-as-you-go model. This means that organizations only pay for what they use, allowing smaller players to compete without breaking the bank.
Additionally, cloud SQL providers usually take care of routine maintenance, security updates, and performance tuning, which reduces the strain on in-house IT resources. This could potentially lead to greater focus on core business operations instead of tinkering with hardware issues.
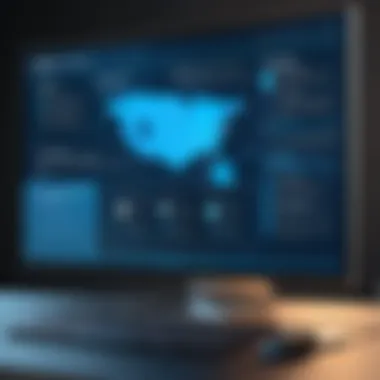
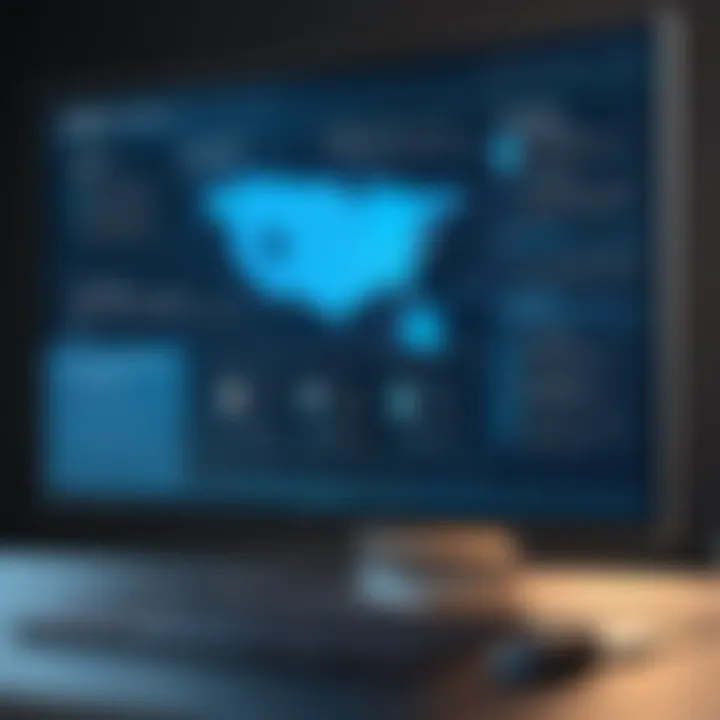
That said, budget-conscious companies ought to keep an eye on accumulating costs due to usage spikes. It’s a balancing act between leveraging the cloud’s potential and ensuring that the budget doesn’t spiral out of control.
Leading Cloud SQL Providers
- Amazon RDS
Amazon RDS stands out amongst cloud SQL services. Known for its user-friendly interface, it allows developers to set up, operate, and scale relational databases in the cloud seamlessly. One of its biggest advantages is multi-availability zones, which enhances data durability and availability.
The unique feature of Amazon RDS lies within its automated backup system and scaling capabilities. Imagine a system where performance bottlenecks disappear as the application grows. The downside? It may not be the cheapest option for businesses with tight budgets; variations in pricing can catch users off guard, primarily based on usage.
- Google BigQuery
Google BigQuery is another key player in the cloud SQL arena, optimized for analyzing large datasets quickly. Thanks to its serverless architecture, users can run queries without worrying about infrastructure. This saves time, allowing analysts to focus on insights rather than setup.
A unique aspect of Google BigQuery is its ability to execute super-fast SQL queries on vast amounts of data due to its columnar storage and distributed architecture. While this is fantastic for large-scale analytics, it may prove confusing for new users who are not accustomed to its interface or capabilities. Expect a learning curve if you’re shifting from traditional SQL environments.
In summary: Cloud SQL solutions are integral in managing big data effectively. The scalability and cost-effectiveness of these services allow businesses to adapt, while top providers like Amazon RDS and Google BigQuery offer distinctive features tailored to specific needs.**
The exploration of SQL in cloud-based solutions not only enhances understanding but also empowers organizations to make data-driven decisions efficiently.
Challenges of Using SQL in Big Data
Navigating the vast landscape of big data offers remarkable opportunities, yet it is not without its hurdles, especially when employing SQL as the primary tool for data manipulation and retrieval. Understanding the challenges present is crucial for developers, IT professionals, and students looking to leverage SQL effectively. This section outlines several prominent difficulties, providing insights into how these can impact the use of SQL in big data environments.
Data Variety and Structure
Big data is characterized by its myriad sources and forms. From social media posts to sensor data from IoT devices, the information can come in structured, semi-structured, or unstructured formats. SQL traditionally excels with structured data, meaning it follows a predefined schema. However, as organizations increasingly rely on diverse data types, SQL faces significant challenges. For instance, datasets might include free-text documents, JSON files, or even multimedia elements, all of which can complicate queries and analysis.
"In today's data-driven world, the variety of data types can present a double-edged sword, enhancing insights while complicating retrieval processes."
Considerations include:
- Schema Management: The ability to adapt to evolving schemas is essential for maintaining data integrity.
- ETL Complexity: Extract, Transform, Load processes can become intricate as data sources diversify, requiring additional computational resources.
- Performance Bottlenecks: Queries must be optimized extensively to handle large volumes of varied data without significant delays.
Latency Issues
Speed is the name of the game in big data analytics. SQL's synchronous nature can introduce latency in processing large datasets, particularly when dealing with real-time data. In scenarios where data must be ingested and acted upon immediately, like fraud detection systems or real-time analytics for stock trading, any delay can negate the effectiveness of the insights drawn.
One often hears that "time is money," and in big data contexts, that could not be more accurate. Critical decisions relying on delayed insights can lead to lost opportunities or diminished competitiveness.
Key factors affecting latency include:
- Query Execution Time: Complex queries can lead to longer execution times, especially as datasets scale.
- Network Delays: In cloud-based environments, data must travel across networks, introducing additional latency.
- Resource Contention: As multiple SQL processes run concurrently, contention for CPU, memory, and I/O can further slow down performance.
Skill Gaps
Despite its rich set of features, SQL may not be a one-size-fits-all solution, particularly in the fast-evolving realm of big data. There exists a notable skill gap in the workforce; many professionals are trained in traditional SQL environments but struggle with its applications in big data settings. The integration of modern frameworks like Hadoop or Apache Spark often requires familiarity with novel SQL engines that differ from classic SQL database management systems.
Bridging this gap is essential for a number of reasons:
- Enhanced Understanding: Professionals need to grasp how SQL interacts with big data technologies to maximize its capabilities effectively.
- Continuous Learning: The tech landscape is ever-changing, so ongoing education and training in SQL's evolution are vital.
- Collaboration with Data Scientists: Often, SQL professionals must team up with data scientists who may have diverging priorities, which necessitates a mutual understanding of each other’s toolsets.
By addressing these challenges, organizations can better utilize SQL, enhancing its effectiveness and ensuring that big data's full potential is realized.
Future Directions for SQL in Big Data
As the landscape of data management continues to evolve, understanding the future directions for SQL in big data becomes crucial. The ongoing advancements in technology are reshaping how we interact with vast datasets. This section will delve into emerging trends and the persistent relevance of SQL amidst these transformations. Recognizing these patterns enables software developers, IT professionals, and students to prepare for the challenges and opportunities that lie ahead.
Emerging Trends
AI and Machine Learning Integration
The integration of AI and machine learning into SQL systems is turning heads these days. This trend is a game-changer, as it allows databases to perform smarter data processing. With the capability to analyze patterns and make predictions, AI and machine learning enhance SQL's ability to manage and query data efficiently.
A key characteristic of this integration is the ability to facilitate automated decision-making processes. For example, algorithms can predict user behavior based on historical data, which improves resource allocation and user experience in real-time applications. This automation offers a beneficial way to tackle the ever-increasing volume of data.
One unique feature is the incorporation of natural language processing (NLP). This allows users to interact with databases using conversational queries, making data retrieval more intuitive. However, one must consider the significant resource requirements and potential bias in AI algorithms, as these can influence outcomes if not managed properly.
Real-Time Data Processing
Real-time data processing is emerging as a crucial aspect for businesses that rely on timely information. SQL systems are increasingly being developed to handle streaming datasets, enabling organizations to make instantaneous decisions based on the most current data available. This capability is invaluable for sectors such as finance, healthcare, and e-commerce, where timing is everything.
A notable characteristic of real-time processing is its ability to provide continuous updates to dashboards and analytics, which keeps stakeholders informed and agile. Companies utilizing this technology can react almost instantly to changing conditions, leading to a competitive edge.
The unique aspect here involves the rising popularity of technologies such as Apache Kafka and Apache Flink, which can be integrated with traditional SQL systems. Yet, this approach isn’t without challenges; managing consistency and latency while ensuring data integrity can be complex and demand robust infrastructure.
SQL's Continuing Relevance
Despite the changes and the introduction of novel systems, SQL remains a foundational pillar in the world of data management. This is primarily because SQL is exceptionally adept at handling structured data. Organizations may depend on various data warehousing solutions, but SQL's structured query language is still the go-to for efficient data retrieval and updates. Its reliability means many businesses aren't just throwing the baby out with the bathwater, but rather evolving their SQL capabilities to meet contemporary demands.
Furthermore, the skill set surrounding SQL is immensely valuable. Developers continue to seek SQL expertise amidst the shifting sands of big data technologies. Not only does this preserve job security for those skilled in SQL, but it also emphasizes how essential understanding traditional systems will remain, especially as the integration of new tools expands.
"SQL has stood the test of time, proving its adaptability and significance in a rapidly changing environment."