Exploring Various Types of Master Data Management Strategies
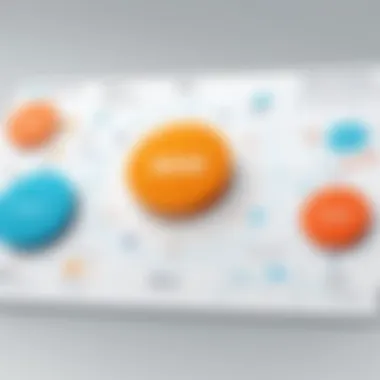
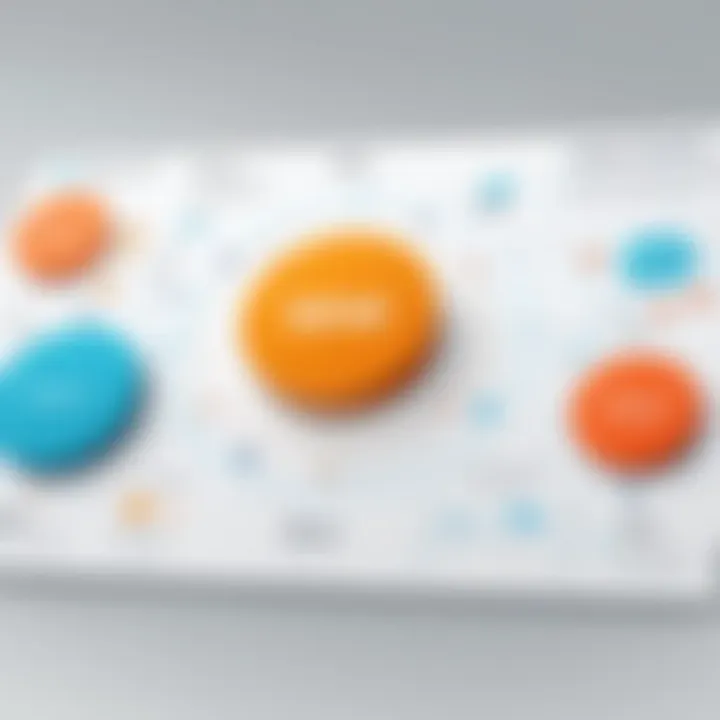
Intro
Master Data Management (MDM) is a critical aspect of data governance in modern organizations. It focuses on the creation and maintaining of consistent, accurate business data across an organization. This article explores different types of MDM, revealing their specific features and practical implementations. By understanding these strategies, professionals can align their MDM choice with the organization’s data needs, enhancing effectiveness and decision-making.
Features and Capabilities
Overview of Key Features
MDM strategies come with a variety of features designed to support data integrity and usability. Key features include:
- Data Integration: MDM systems consolidate data from various sources to create a single, authoritative source. This integration reduces data duplication and conflicts.
- Data Quality Control: MDM emphasizes data cleansing and validation to ensure data accuracy. This includes error detection and correction mechanisms.
- Workflow Management: MDM systems enable businesses to define and automate workflows for data stewardship and governance. This capability increases accountability and efficiency.
- Hierarchy Management: Organizations often need to manage relationships between data entities. MDM strategies provide tools for visualization and management of these hierarchies, improving data contextualization.
User Interface and Experience
The user interface of MDM systems greatly impacts user adoption and efficiency. Effective MDM solutions often feature:
- Intuitive Dashboards: Clear and concise dashboards allow users to view key metrics at a glance.
- Search Functionality: Robust search capabilities aid users in quickly finding specific data entities without extensive filtering.
- Customization Options: Users appreciate systems that can be tailored to their operational needs, facilitating ease of use and better alignment with workflows.
Performance and Reliability
Speed and Efficiency
Performance is vital in MDM systems, especially in data-intensive environments. Key aspects include:
- Data Processing Speed: Fast data ingestion and processing capabilities enable organizations to handle large volumes of data efficiently.
- Scalability: MDM solutions must scale with business growth, accommodating increasing amounts of data without performance degradation.
Downtime and Support
Reliability is just as important as performance. Effective MDM systems provide:
- Minimal Downtime: Continuous operation is essential for business functionality. High availability setups ensure systems remain operational even during maintenance.
- Technical Support: Strong support services and resources, such as documentation and community forums, are crucial for users to resolve issues quickly.
"Effective Master Data Management can transform how organizations interact with data, leading to enhanced decision-making and operational success."
Understanding these features and performance aspects is necessary for making informed decisions on MDM strategies. The right choice can substantially impact organizational data integrity and efficiency.
Intro to Master Data Management
Master Data Management (MDM) is a crucial discipline for businesses that rely on data to drive decisions and operational processes. The importance of MDM lies in its ability to create a single, accurate view of key business entities, such as customers, products, and suppliers. This ensures that all departments within an organization are working with the same foundational data, which fosters consistency and eliminates discrepancies.
In this article, we will explore the various types of MDM and their specific characteristics. Understanding these differences is key to implementing the most suitable MDM strategy for any organization. When organizations adopt the appropriate MDM approach, they stand to gain enhanced data quality, improved operational efficiency, and better decision-making.
Understanding Master Data
Master data refers to the critical data that is essential for operations within a business. This can include information about customers, products, suppliers, and more. Unlike transactional data which is constantly changing, master data tends to remain relatively stable over time. Managing this data effectively is vital for maintaining business operations and ensuring accurate reporting and analytics.
There are several components of master data:
- Customer Data: Information that identifies customers and their interactions with the business.
- Product Data: Descriptions and specifications of products or services offered.
- Supplier Data: Detailed records of suppliers and their arrangement with the organization.
- Location Data: Information that relates to the geographical aspects of the business.
Implementing a robust MDM strategy helps ensure that master data is accurate and consistent across all systems and platforms.
Why is Important
MDM plays a pivotal role in today's data-driven landscape. Ensuring the accuracy of master data significantly impacts various aspects of the organization.
- Enhanced Data Quality: By employing an effective MDM strategy, organizations can significantly improve the reliability of their data. High-quality data leads to more precise analyses and insights.
- Operational Efficiency: When different departments access consistent master data, it reduces the time spent reconciling discrepancies and streamlines workflows.
- Improved Decision-Making: Accurate master data allows businesses to make informed decisions based on solid data analytics. This can lead to tactical advantages in the market.
- Regulatory Compliance: A well-structured MDM system can help organizations adhere to industry regulations by ensuring that data management practices are compliant.
- Customer Satisfaction: Having a clear and accurate view of customer data enhances customer relationship management and ultimately improves customer satisfaction.
"An organization that leverages MDM effectively is better positioned to respond to changing market demands and capitalize on new opportunities."
By understanding and implementing Master Data Management, businesses can establish a foundation of credibility and trust in their data, essential for long-term success.
Types of
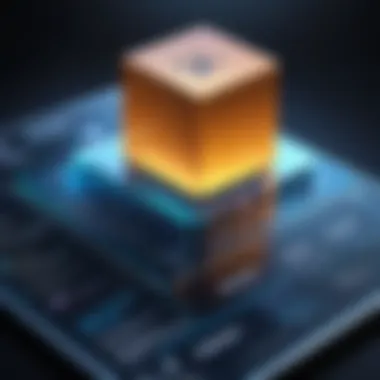
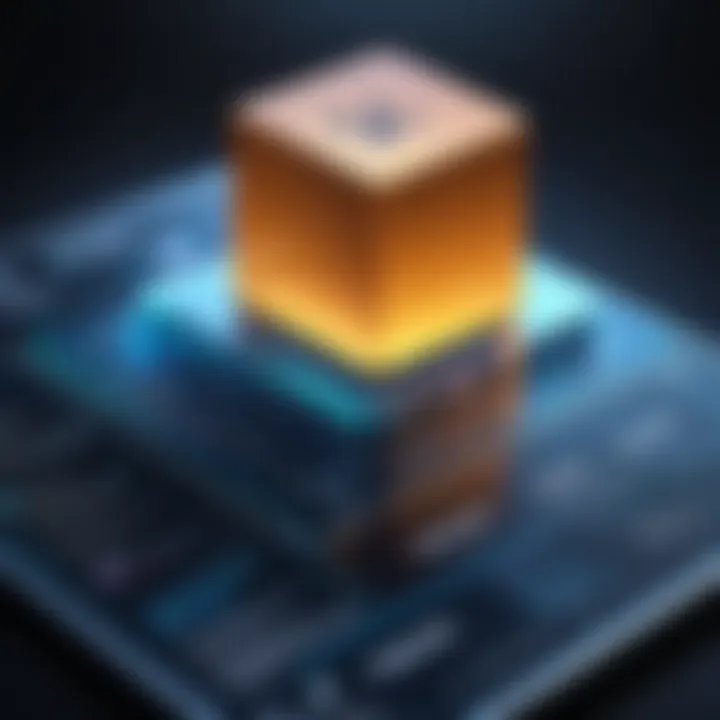
The concept of Master Data Management (MDM) is complex. Different types of MDM cater to varied business needs and scenarios. Understanding these types is crucial for organizations aiming to enhance their data management strategies. Each type of MDM has specific elements that provide unique benefits. By exploring these differences, organizations can align their MDM approach with their operational requirements and data integrity goals.
Operational
Operational MDM focuses on managing data used in day-to-day business operations. It ensures that critical data across various systems remains consistent and accurate. This type of MDM supports transactional processes and real-time applications.
One of the primary benefits includes improved efficiency. When data is reliable, it simplifies operations. Additionally, operational MDM helps in reducing data redundancy and potential conflicts that can arise in business processes.
Analytical
Analytical MDM pertains to managing data for reporting and analysis purposes. It is designed to integrate data from different sources for analytics, thus enabling informed decision-making. This type is critical for organizations that rely on insights drawn from data.
The main advantage of analytical MDM is its ability to provide a unified view of business data. It assists analysts in understanding trends and performance metrics. Furthermore, accurate analytical data can enhance strategic planning and forecasting.
Collaborative
Collaborative MDM emphasizes shared data management across various teams in an organization. This approach is essential in situations where multiple departments need access to the same data sets. By fostering collaboration, organizations can ensure that everyone operates with the same information.
Key benefits include increased data accuracy and a reduction in redundant data entries. Collaboration leads to a better understanding of data needs and improves overall data quality across the board.
Consolidation
Consolidation MDM involves merging data from different sources into a single, authoritative source. This type is favored when organizations have multiple systems that hold the same data but with variations.
The significance of this approach lies in its ability to streamline data processes. By ensuring that all data is consolidated, organizations can avoid the confusion that arises from having several versions of the same data. It enhances data governance and accuracy.
Registry
Registry MDM functions as a reference point for master data across various systems. This type does not store all data but provides a framework for accessing it from different repositories. It contains metadata to describe the data instead.
Registry MDM is advantageous because it aids in maintaining consistency without duplicating data. This method also provides flexibility in accessing and managing master data from various sources without the need for full data relocation.
Coexistence
Coexistence MDM allows organizations to maintain multiple data sources while establishing a unified view of critical data. It enables compatibility between diverse systems while supporting various business processes.
The primary benefit here is adaptability. Organizations can evolve their data management methods as needed without a complete overhaul of existing systems. This approach also facilitates a gradual transition towards more streamlined data management solutions.
Characteristics of Different Types
Understanding the characteristics of different types of Master Data Management (MDM) is crucial for organizations aiming to optimize their data handling processes. Each type serves unique purposes that align with various business strategies. Comprehending these characteristics allows organizations to choose an MDM approach that fits their specific needs. Key elements include data governance, quality management, integration strategies, and change management. These factors collectively determine how efficiently an organization can manage its essential data while adhering to regulatory compliances.
Data Governance and Compliance
The foundation of Master Data Management lies in data governance. This practice ensures that data is accurate, consistent, and trustworthy. Proper data governance structures help maintain compliance with regulations like GDPR or HIPAA. Compliance is not only about adhering to laws, but also fostering trust among stakeholders.
Important aspects include:
- Policies and Procedures: Creating guidelines for data usage and management.
- Roles and Responsibilities: Clearly assigning ownership and accountability for data integrity.
- Monitoring and Auditing: Continually assessing compliance and data quality standards.
By implementing strong governance, organizations can significantly reduce risks associated with data breaches or misutilization.
Data Quality Management
Data quality is a critical component in MDM. It directly influences business decisions and operational efficiency. High-quality data enhances analytical capabilities and leads to better forecasting and strategic planning. Key elements to consider include:
- Accuracy: Ensuring data reflects the real-world situation it represents.
- Completeness: Having all necessary data elements to support business needs.
- Consistency: Maintaining uniformity of data across various systems.
Inconsistent or poor-quality data can lead to faulty conclusions, affecting the entire organization. Therefore, a robust data quality management strategy is necessary.
Data Integration Strategies
Integrating data from diverse sources is vital for effective MDM. Well-defined integration strategies streamline data flow and enhance accessibility. Effective strategies often involve:
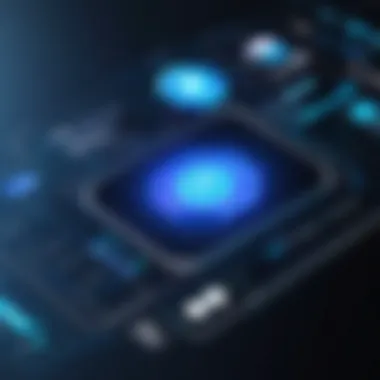
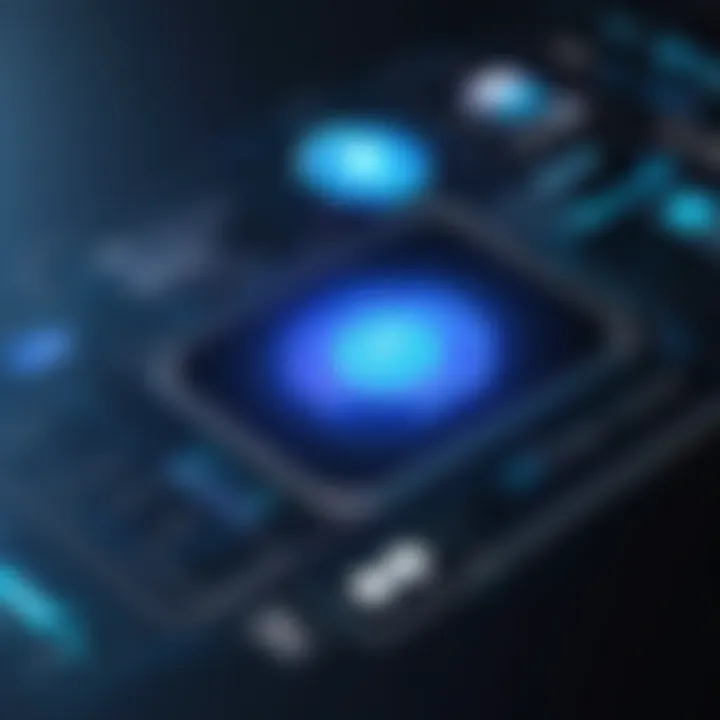
- ETL (Extract, Transform, Load) processes for consolidating data from multiple systems.
- API Utilization to enable real-time data exchange.
- Master Data Consolidation to create a single source of truth.
By investing in integration, companies can enhance efficiency and maintain consistency across different departments.
Change Management in
Change management is an essential aspect of implementing MDM solutions. Introducing new processes often meets with resistance from employees. Successful change involves:
- Communication: Keeping all stakeholders informed about new strategies.
- Training Programs: Ensuring team members are equipped to handle new systems and processes.
- Feedback Mechanism: Creating avenues for user feedback can help in adjusting processes that are not working well.
Without effective change management, MDM initiatives are likely to fail, as user acceptance is critical for data management success.
"Ultimately, the effectiveness of MDM depends not only on technology but also on the human factors that interact with it."
These characteristics illustrate the diverse elements at play in Master Data Management. Recognizing these can enhance organizational readiness to adapt to changing data landscapes.
Implementation Challenges in
Master Data Management (MDM) is a critical aspect of data governance and integrity, but its implementation is often fraught with challenges. Understanding these challenges is essential for organizations looking to effectively manage their master data. Identifying and addressing these issues can lead to improved data quality, seamless integration, and ultimately enhanced operational performance.
Data Silos
Data silos represent a fundamental barrier in the implementation of MDM. They occur when different departments or systems within an organization do not share information, thus leading to inconsistencies in data. For example, if the sales team uses a separate customer database from the marketing team, discrepancies can arise regarding customer information. This fragmentation can hinder accurate reporting, complicate analytical efforts, and, ultimately, impair decision-making.
To overcome the challenge of data silos, organizations must prioritize data integration. Effective integration strategies can help unify disparate data sources. Companies may choose solutions like data warehouses or data lakes to consolidate information. The importance of breaking down these silos cannot be underestimated; continuity in data ensures that every department operates with the same accurate dataset.
Cultural Resistance
Cultural resistance is another significant barrier to MDM implementation. Changing how a workforce approaches data can meet significant pushback from employees. Individuals often prefer their established routines and may distrust new systems or processes. This resistance can stem from a lack of understanding of the benefits that effective MDM brings to their roles or the organization as a whole.
Addressing cultural resistance requires effective change management strategies, including clear communication and education. Training sessions can be utilized to demonstrate the advantages of MDM and its relevance in daily tasks. Additionally, involving team members in the MDM development process can foster a sense of ownership and cooperation, easing transition and increasing the odds of successful adoption.
Technology Integration Issues
Technology integration issues can impede MDM efforts considerably. Many organizations utilize a variety of software solutions, databases, and tools. Ensuring these systems work together cohesively can be quite complex. For instance, challenges can arise when attempting to align cloud-based systems with on-premise infrastructure.
To handle these integration challenges, organizations may need to invest in middleware solutions or APIs that facilitate communication between systems. Additionally, thorough testing of all integrated systems is crucial. This can help catch issues early and rectify them before full implementation. Adopting comprehensive integration strategies ensures that the various technology components align with the overarching goals of MDM.
Success in MDM execution hinges on recognizing these challenges early and developing strategic plans to address them effectively.
By proactively tackling data silos, cultural resistance, and technology integration issues, organizations can enhance their chances of successful MDM implementation. Ultimately, these efforts contribute to better data quality and operational efficiency.
Selecting the Right Approach
Selecting the right Master Data Management (MDM) approach is crucial for organizations aiming to maintain data integrity and improve operational efficiency. This process involves not only understanding the organization's specific needs but also considering various factors such as cost, scalability, and flexibility. Each organization is unique and requires a tailored solution that aligns with its comprehensive data strategy. A well-chosen MDM approach can lead to significant enhancements in data usability and management.
Assessing Organizational Needs
When assessing organizational needs, it is essential to evaluate the current state of data across departments. Understanding what data is critical for various business processes helps identify specific requirements for an MDM solution. It is common for organizations to face challenges like inconsistent data, lack of visibility, and data silos. Conducting a thorough assessment allows decision-makers to pinpoint which type of MDM—operational, analytical, or collaborative—can best address these issues.
Some key questions include:
- What types of data are most important to your business?
- Who are the primary stakeholders impacted by this data?
- What are the existing pain points related to data management in your organization?
Cost Considerations
Cost is a significant factor when selecting an MDM solution. Organizations must weigh the initial investment against the long-term benefits of improved data management. Budget allocations might vary based on the company's size, industry, and specific objectives. It's critical to consider not just software expenses but also costs related to implementation, maintenance, and potential training sessions for staff.
Evaluating the total cost of ownership (TCO) can provide a clearer picture. Some essential aspects to consider include:
- Licensing fees for MDM software.
- Costs associated with data migration and integration.
- Ongoing support and maintenance costs.
- Potential increases in operational efficiency that can offset these costs.
Scalability and Flexibility
Lastly, the scalability and flexibility of the MDM solution are vital for accommodating future growth and changing data management needs. As organizations evolve, their data strategies will also need to change to match increased data volume and complexity. A suitable MDM approach should allow for adjustments without requiring a complete overhaul of the existing system.
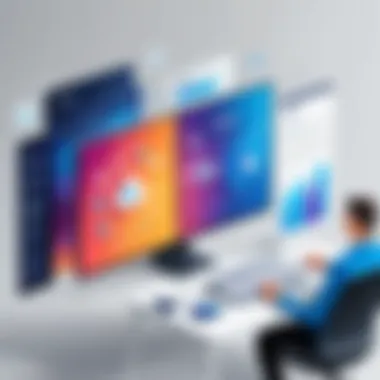
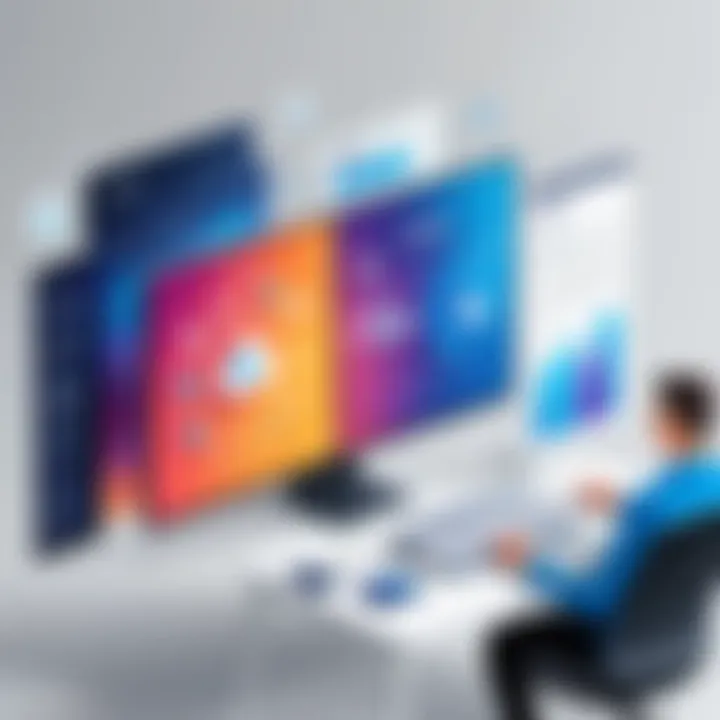
Factors to examine include:
- How easily can the MDM solution scale to handle additional data sources or increased data volumes?
- Does the solution allow for customizability to fit different departmental needs?
- Is there support for various data types and sources to ensure compatibility?
Choosing the right MDM approach isn't just a technical decision; it reflects the strategic direction of the organization and its commitment to effective data governance.
Best Practices for Implementation
Master Data Management (MDM) is integral to an organization's data strategy. Adhering to best practices ensures the effective implementation of MDM. This section outlines essential elements that contribute to a successful MDM framework. The focus is on strategies that promote accuracy, governance, and collaboration in managing data.
Establishing Strong Governance Frameworks
Strong governance is the cornerstone of effective MDM. It ensures accountability and maintains the integrity of data. Within a governance framework, roles and responsibilities must be clearly defined. This includes data stewards who oversee the quality and consistency of master data. Having a consistent process for managing data lifecycle is also crucial. This may involve procedures for data creation, updates, and archiving. Moreover, establishing policies that dictate data usage helps to ensure compliance with regulatory requirements. Considerations around data ownership and stewardship play a pivotal role in governance.
Encouraging Cross-Departmental Collaboration
Collaboration across departments enhances the effectiveness of MDM. Different teams hold varied insights into data requirements and business processes. When teams work together, the potential for misalignment decreases. Regular meetings and workshops facilitate open communication. This collaboration can also lead to a shared understanding of data policies and procedures. Encouraging input from various departments fosters a culture of data accountability. Utilizing collaboration tools can streamline this process, making it easier for teams to share insights and updates.
Investing in Technology Solutions
Technology plays a vital role in supporting MDM initiatives. Investing in the right tools can streamline data management processes and enhance data quality. Solutions may include dedicated MDM platforms that offer functionalities like data integration, quality management, and reporting. Organizations should evaluate various options in the market. This evaluation should focus on scalability, flexibility, and ease of use. Implementing automation in data workflows reduces manual errors and saves time. Furthermore, leveraging cloud-based solutions offers additional benefits, such as improved accessibility and cost-effectiveness.
The right technology paired with solid governance and collaboration can transform an organization’s approach to data management.
Successful MDM implementation hinges on these best practices. They not only aid in achieving organizational objectives but also foster a more data-driven culture.
Future Trends in
Master Data Management (MDM) is not a static field. It continually evolves alongside technological advancements and market demands. Understanding future trends in MDM is key for organizations looking to stay competitive. These trends reveal how businesses can leverage emerging technologies to enhance data accuracy, accessibility, and usability, ultimately improving overall operational efficiency.
AI and Machine Learning in
Artificial Intelligence and Machine Learning are rapidly changing how Master Data Management operates. One major benefit is the ability to automate data quality processes. AI algorithms can detect anomalies, ensuring that master data remains clean and accurate. They can also predict potential data issues before they affect decision-making. This proactive approach minimizes risks associated with poor data management.
Machine Learning can significantly enhance data integration efforts. By analyzing historical data patterns, algorithms can suggest optimal ways to connect different data sources. This results in faster and more efficient integrations, allowing organizations to respond more adeptly to changing market conditions.
Cloud-Based Solutions
The adoption of cloud-based MDM solutions is rising among organizations. These platforms offer flexibility and scalability that on-premises systems often lack. Companies can store large amounts of master data in a centralized repository, accessible from anywhere with internet connectivity.
Cloud solutions also facilitate easier implementation and updates, as vendors typically handle system maintenance. This means that businesses can focus on maximizing data utility instead of worrying about infrastructure. Furthermore, the pay-as-you-go model often used with cloud services can result in cost savings, as organizations only pay for what they use.
Integration with Data Analytics
As the importance of data analytics continues to grow, the integration of MDM with analytics platforms becomes crucial. Organizations can gain a comprehensive view of their data landscape by linking MDM systems with data analytics tools. This ensures that decisions are based on reliable, high-quality data.
The synergy between MDM and analytics fosters improved reporting capabilities. Companies can generate insights that inform strategic initiatives and operational efficiencies. Additionally, integrating MDM with analytics helps organizations comply with data governance policies. They can track data lineage and validate that the data used in analytical processes meets quality standards.
Organizations must remain vigilant of these trends, ensuring they not only follow them but also leverage their potential to drive significant improvements in Master Data Management.
Epilogue
Concluding this exploration of Master Data Management (MDM) is vital. The discussion reveals how MDM processes and technologies play a critical role in managing key business data effectively. Each MDM type has its own unique characteristics, strengths, and applications that can be leveraged based on organizational needs. By recognizing these aspects, businesses can implement more structured data governance frameworks.
Summary of Key Considerations
When considering MDM, several key points should be taken into account:
- Data Quality: High-quality data is essential for effective decision-making. Inaccurate or inconsistent data can lead to misguided strategies and operational failures.
- Integration: MDM is not just about data storage but also about how data integrates across platforms. To be successful, integration strategies must be in place.
- Governance: A strong governance policy ensures that data management processes align with overall business objectives. This creates a culture of accountability.
- Scalability: As organizations grow, their data management needs will evolve. The chosen MDM approach must be flexible enough to adapt to these changes.
Communicating these considerations effectively helps organizations evaluate how MDM fit into their specific context.
Final Recommendations
To solidify the comprehension of MDM, here are some final suggestions:
- Evaluate Organizational Needs: Each company's data landscape is unique. An initial assessment will help determine the most appropriate MDM strategy for implementation.
- Invest in Technology Solutions: Leveraging tools like Informatica and Talend can aid in streamlining MDM processes and integrating technologies effectively.
- Engage Stakeholders: Involving all relevant teams nurtures a collaborative atmosphere, vital for a successful MDM initiative. Collaboration fosters buy-in from all levels of the organization.
- Monitor and Adjust: Implementing MDM is not a one-time activity. Ongoing monitoring and evaluation are crucial to adapting strategies as organizational requirements change.
In summary, understanding the types of MDM available and their implications is key to unlocking better data management practices. Aligning MDM approaches with business goals leads to improved operation efficiency and strategic advantage.