Exploring the Impact of Solver Analytics on Business
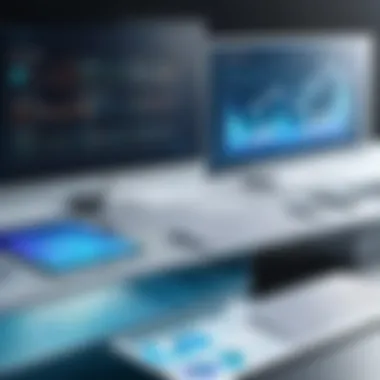
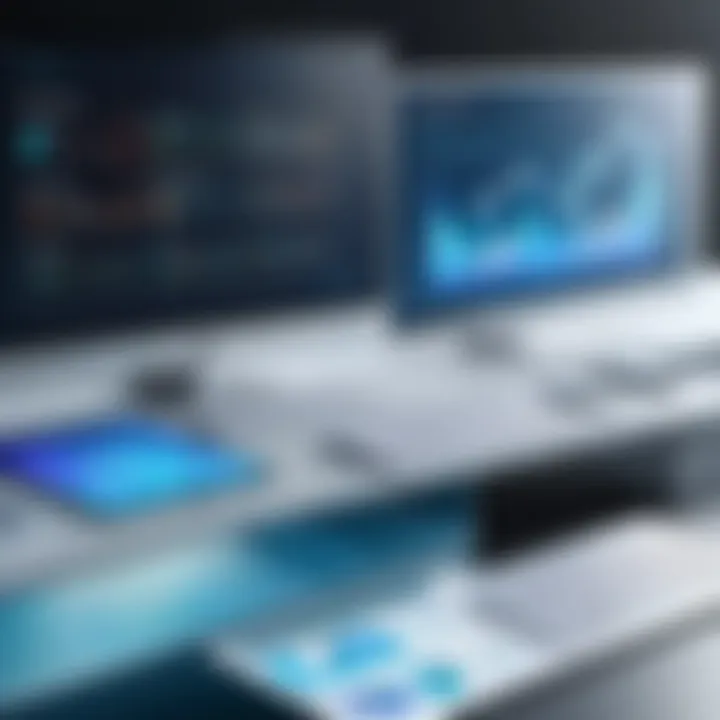
Intro
In today’s fast-paced world, decision-making is more complex than ever. Companies face a myriad of challenges, often hindered by vast amounts of data. Solver analytics emerge as a critical tool for organizations striving to optimize their operations in this intricate landscape. It’s not just about crunching numbers; it’s about unlocking the potential within data to make informed decisions.
But what exactly does solver analytics entail?
It encompasses various methods and technologies that empower data-driven decision-making, enabling businesses to navigate uncertainties. This exploration will equip readers with a deep understanding of the features, capabilities, and emerging trends in this pivotal area.
As we dive further, we’ll discuss key methodologies that leverage solver analytics, showcase various applications across sectors, and uncover the transformational impact these tools can have on operational efficiency. Get ready to explore a comprehensive framework that breaks down the complexities of solver analytics thoughtfully.
Prolusion to Solver Analytics
Solver analytics has emerged as a cornerstone in today's business landscape, particularly as organizations are increasingly faced with intricate challenges and massive datasets. The importance of this topic lies in its ability to drive efficiency, improve decision-making, and enhance strategic planning across various sectors. By leveraging solver analytics, businesses can unlock insights that were previously buried under layers of data, leading to better outcomes.
The benefits of employing solver analytics in a business setting are manifold. These analytical tools provide clarity amid complexity, enabling companies to identify patterns, optimize processes, and allocate resources more effectively. They facilitate a thorough examination of problems through quantitative methods, allowing decision-makers to choose from a range of options based on data-driven evidence rather than intuition alone.
Several factors warrant careful consideration when discussing solver analytics. First, the underlying mathematical models must be robust and applicable to the specific scenarios a business faces. Without a solid foundation, the results derived from these models can lead to misguided strategies. Additionally, proper data input and processing are crucial; inaccurate or poor-quality data can throw a wrench into the analysis and skew results.
In the following sections, we will peel back the layers to better understand the definition of solver analytics, trace its historical development, and explore its core components and applications. This comprehensive exploration not only aims to enlighten software developers and IT professionals but also engages students and other interested parties who wish to deepen their understanding of a field that continues to evolve at a rapid pace.
"The power of data lies not in its abundance, but in the insights we can extract from it."
As we embark on this journey into the world of solver analytics, let's first establish a clear definition of what this term encompasses.
Core Components of Solver Analytics
The significance of core components in solver analytics cannot be understated. These elements form the backbone of how data is interpreted, processed, and utilized for decision-making. Without a nuanced understanding of each component, the entire analytic process may become muddled or lead to suboptimal outcomes. The interplay between models and data reflects an essential aspect of analytics that is consistently underscored in its practical applications across various fields.
Mathematical Models
Mathematical models serve as the foundation of any analytics endeavor. They present a simplified representation of complex real-world problems. For instance, think of a transportation company that needs to minimize costs while maximizing efficiency. A mathematical model could depict the various routes available, the costs incurred for each route, and the demand for deliveries. Using important variables along with constraints, these models help businesses look for optimal solutions in scenarios of limited resources.
Several techniques come into play here:
- Linear Programming: Often used for problems where relationships can be expressed as linear equations. It’s effective in resource allocation scenarios.
- Non-linear Models: For situations where relationships are more complex and cannot be simply expressed through straight lines.
- Dynamic Models: Useful for problems that change over time, adapting to real-time variables and updated conditions.
A well-structured mathematical model doesn’t just solve a problem; it paints a broader picture. Understanding how various elements interact allows professionals to forecast potential roadblocks. Models should be tested and validated with historical data to ensure their robustness. This iterative process promotes a more accurate representation.
Data Input and Processing
Data input and processing is intertwined with the mathematical models to form comprehensive insights. Raw data in itself is like rough ore; it needs refinement before it can yield value. The process of organizing, cleaning, and preparing the data is critical.
A myriad of challenges can arise at this stage:
- Quality: If the data is incorrect or unreliable, the output will be flawed. Ensuring that data is accurate and trustworthy is paramount, especially for analytics.
- Volume: Big data presents both opportunities and challenges. Analytical tools must have the capability to handle large datasets effectively.
- Variability: Data can come in various forms—structured, unstructured, or semi-structured. The tools employed must be able to adapt to changing types and sources.
Essentially, data can be processed through various methodologies, such as:
- ETL (Extract, Transform, Load): This process takes data from different sources, cleans it up, and loads it into a database or warehouse for analysis.
- Data Warehousing: Acquiring and storing large datasets in a comprehensive manner makes analysis seamless.
- Real-Time Data Processing: In scenarios where immediate insights are necessary, tools that can analyze data as it arrives are crucial.
The collaboration between mathematical models and processed data is what enables solver analytics to provide actionable insights. It’s like a finely tuned machine, where each component works in harmony to drive results. The implications of optimizing these core components are substantial, equipping organizations to move forward with confidence in their strategic initiatives.
Types of Solver Analytics
In the realm of solver analytics, understanding its different types is crucial for effectively addressing complex business challenges. These types serve as the backbone for deriving insights from data, enabling organizations to make informed decisions and optimize performance. The following sections will explore three major categories of solver analytics: descriptive, predictive, and prescriptive analytics. Each type comes with distinct advantages and applications, thus elevating the decision-making process.
Descriptive Analytics
Descriptive analytics is often considered the foundation of data analysis. It involves the interpretation of historical data to understand what has happened in a business context. At its core, descriptive analytics answers questions like "What occurred?" and "What were the metrics around these events?" This type of analytics is beneficial in various ways:
- Data Aggregation: It consolidates vast amounts of data into a comprehensible format.
- Trend Identification: Companies can recognize patterns over time, paving the way for strategic planning.
- Performance Measurement: Useful for establishing benchmarks that help assess business performance.
For example, a retail company may utilize descriptive analytics to evaluate sales performance over specific time frames. Imagine analyzing sales data across different regions to uncover trends. This insight enables firms to identify high-performing sectors while flagging areas needing improvement. Moreover, visualization tools play a significant role here, transforming raw numbers into intuitive dashboards and charts that speak volumes at a glance.
Predictive Analytics
Predictive analytics takes things a step further by utilizing historical data to forecast future outcomes. As businesses gather more information, predictive analytics can help illuminate trends that may not just be evident in past metrics. It primarily addresses questions such as "What is likely to happen next?" This type of analytics boasts several key benefits:
- Informed Decision-Making: By predicting potential outcomes, businesses can prepare accordingly.
- Risk Management: Organizations can calculate the likelihood of adverse events, helping them create contingency plans.
- Resource Allocation: It enhances the ability to distribute resources efficiently based on anticipated needs.
In practice, imagine a financial institution employing predictive analytics to assess loan applications. By analyzing previous loan performance data, the institution can predict which applications are most likely to default. This opens up strategic advantages, as they can adjust lending criteria proactively to mitigate risk. Tools such as machine learning and statistical models underpin this analysis, providing robust frameworks for crafting predictions.
Prescriptive Analytics
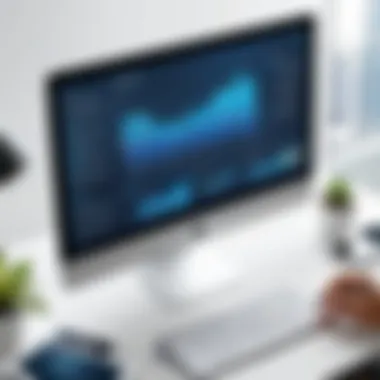
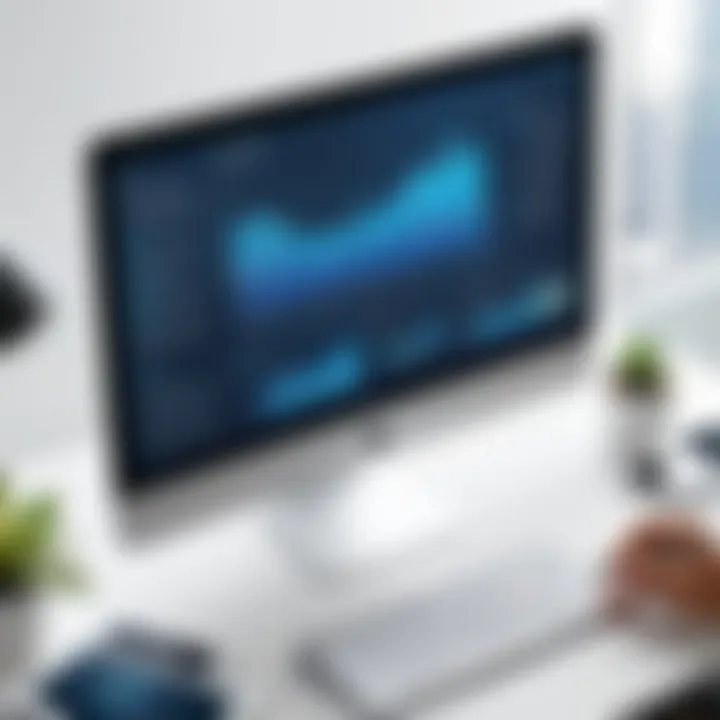
When it comes to optimization, prescriptive analytics takes the crown. Unlike its predecessors, this type doesn't merely tell what happened or what might happen; it suggests actionable steps to achieve desired outcomes. Questions like "What should we do?" are central to prescriptive analytics. Key aspects include:
- Action Recommendations: It not only identifies potential actions but also evaluates the outcomes associated with each.
- Optimization Models: Businesses can simulate various scenarios to determine the most effective paths forward.
- Dynamic Decision Making: It adapts to changing circumstances, leading to real-time responsiveness in business operations.
For instance, consider a logistics company seeking to optimize delivery routes. By employing prescriptive analytics, they can simulate multiple routing scenarios considering factors like traffic, weather conditions, and demand patterns. The algorithms will analyze these variables and recommend the most efficient routes while minimizing costs and maximizing customer satisfaction. Through this lens, prescriptive analytics becomes a game-changer for businesses operating in fast-paced environments.
"Analytics are the eyes that let you see how your business is doing and where it can go."
The types of solver analytics offer a well-rounded toolkit for organizations looking to navigate the complexities of data in decision-making. From understanding the past and predicting the future to crafting actionable strategies, these analytical forms illustrate the multifaceted opportunities available for businesses today.
Applications of Solver Analytics in Business
In the intricate dance of modern business, the prospect of harnessing solver analytics emerges as both vital and transformative. The ability of businesses to leverage analytical tools to improve decision-making processes across various domains is crucial for maintaining a competitive edge. From fine-tuning operations to making informed strategic decisions, the applications of solver analytics pave the way for clearer insights and better outcomes. With a keen focus on specific use cases, this section delves into how solver analytics can be effectively utilized in three pivotal areas: supply chain optimization, financial modeling, and marketing and sales forecasting.
Supply Chain Optimization
The logistics game is no stroll in the park. Companies are often caught in a maze of dependencies and variables that influence product flow. Solver analytics comes into play here by providing a structured approach to unraveling complexities. Companies like Amazon have demonstrated how efficient supply chain management significantly impacts overall performance and customer satisfaction.
One example might involve using linear programming to minimize transportation costs while considering constraints such as delivery windows and vehicle capacities. This method not only reduces expenses but also ensures timely deliveries, bolstering customer trust.
- Key Benefits:
- Cost Reduction: By optimizing routes and inventory levels, businesses can cut down on operational expenditures.
- Enhanced Efficiency: Streamlined supply operations improve response times and contribute to an agile business model.
- Increased Transparency: Analytics tools provide real-time insights into supply chain performance, aiding quick decision-making.
In short, employing solver analytics in supply chain management leads to a more resilient and efficient operation.
Financial Modeling
When it comes to predicting a company’s financial future, relying on gut feelings usually falls flat. Solver analytics equips finance professionals with the expertise needed to develop robust financial models. Consider the use of Monte Carlo simulations, where different scenarios can be assessed to understand potential risks and returns. This critical analysis can guide investment strategies and budget allocation.
For instance, a business can leverage data on historical revenue and expenses to craft more accurate future projections. By incorporating various financial metrics and risk factors, organizations can assess potential outcomes and make more informed decisions.
- Key Advantages:
- Risk Mitigation: With a clearer view of potential financial scenarios, businesses can prepare better for uncertainty.
- Strategic Planning: Insights from thorough financial models inform long-term strategies and resource allocation.
- Performance Tracking: Ongoing analysis allows businesses to measure actual performance against forecasts, enabling timely adjustments.
Ultimately, the integration of solver analytics into financial modeling serves as a guiding compass amidst the stormy seas of financial operations.
Marketing and Sales Forecasting
In the highly competitive market landscape, understanding consumer behavior can feel like trying to catch smoke with your bare hands. However, solver analytics dismantles this confusion by providing a data-driven approach to marketing and sales forecasting. Brands like Netflix and Spotify thrive on analytics to predict user preferences and enhance engagement.
For example, predictive analytics can identify trends based on customer data, enabling tailored marketing campaigns that resonate with targeted demographics. By applying historical sales data and market research, a company can forecast future sales with impressive accuracy.
- Crucial Outcomes:
- Targeted Campaigns: By understanding customer preferences, marketing efforts can be streamlined for better conversion rates.
- Inventory Management: Accurate sales forecasts ensure optimal stock levels, preventing overstocking or stockouts.
- Budget Optimization: Understanding peaks and valleys in sales patterns allows for more effective resource allocation.
In sum, incorporating solver analytics into marketing strategies not only amplifies efficiency but also enhances customer satisfaction and loyalty.
“The real key to success in analytics lies not just in having data, but in understanding how to use it creatively to solve problems.”
Through these applications, it becomes evident that solver analytics signifies more than just a technological advantage; it represents a fundamental shift towards data-centric decision-making that can enlighten every facet of business operations.
Key Methodologies in Solver Analytics
Understanding the foundational methodologies in solver analytics is crucial for anyone looking to harness the power of data-driven decision-making. These methodologies offer structured approaches to problem-solving, enabling organizations to derive actionable insights from complex datasets. Each technique serves a unique purpose and brings different advantages to the table, shaping how businesses tackle challenges and optimize their operations.
Linear Programming
Linear programming is a powerful mathematical method used to find the best possible outcome in a given mathematical model, subject to constraints. This technique thrives in situations where a linear relationship dominates the variables involved. For instance, a company might want to maximize profits while minimizing costs, taking into consideration resource limitations like budget or time.
A classic application of linear programming can be seen in resource allocation. Consider a factory producing two products; the aim is to determine how many of each product to manufacture to achieve the highest profit while being constrained by machine hours, labor hours, or material supplies.
Some key points about linear programming include:
- Clarity in Modeling: It helps clarify the problem by translating real-world situations into mathematical equations, making it easier to visualize potential outcomes.
- Wide Applicability: Industries like finance, logistics, and manufacturing frequently utilize linear programming to optimize operations.
- Sensitivity Analysis: This is a remarkable feature that assesses how different values of an independent variable affect a particular dependent variable under a given set of assumptions.
> "Linear programming bridges the gap between complex real-world decisions and simplified mathematical models."
Integer Programming
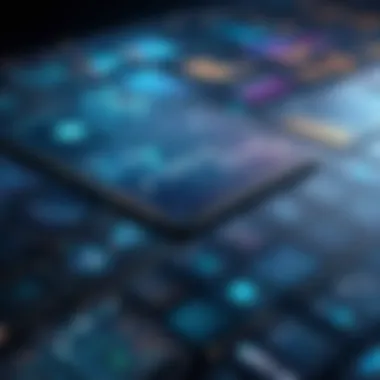
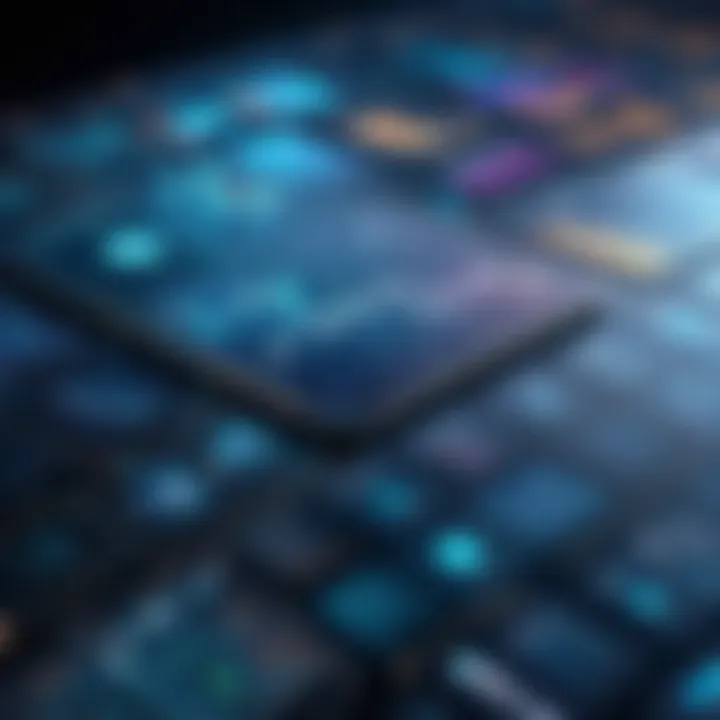
Integer programming is a special case of linear programming. It focuses on optimization problems where some or all of the variables are constrained to be integers. This approach is particularly useful in situations where you cannot break down items; for example, you cannot produce half a truck if the variable represents the number of trucks.
Take a logistics company, tasked with deciding how many delivery trucks to operate within city limits. Each truck can carry a specific amount of goods, and the decision hinges on factors like route efficiency, customer demand, and operational costs.
Key features of integer programming involve:
- Discreteness of Variables: Since integer programming deals with whole numbers, it fits naturally with issues such as scheduling, assigning resources, or any scenario requiring all-or-nothing decisions.
- Complexity: While this technique is useful, its computational intensity can be a hurdle. Finding optimal solutions in integer programming often requires more computational resources than linear programming solutions.
In practice, integer programming can include:
- 0-1 Integer Programming: This form uses binary variables and is useful in yes/no decisions, like determining whether to open or close a facility.
- Mixed Integer Programming: This incorporates both integer and non-integer variables, offering flexibility in modeling.
Simulation-Based Techniques
Simulation-based techniques employ models to replicate complex systems and processes in a controlled virtual environment. These methods provide insight into how a system behaves under various scenarios, helping decision-makers evaluate risks and potential outcomes.
An excellent example of this is in the financial sector, where companies use simulations to predict future market movements based on historical data. By testing various market conditions, firms can build a portfolio strategy that maximizes returns while minimizing risks.
Important aspects of simulation techniques include:
- Uncertainty Management: Simulation allows businesses to assess the impact of uncertainty in their models. This aspect is particularly valuable in risk analysis or resource planning, where the future is unpredictable.
- Flexibility: These methods can be tailored to suit a wide range of industries—from healthcare to manufacturing—ensuring broad applicability.
- Scenarios Testing: It enables users to run multiple 'what-if' scenarios, making it easier to visualize the effects of different decisions before implementing them in the real world.
The essence of utilizing simulation techniques lies in their ability to inform decision-making processes with a significant level of granularity, helping organizations navigate the murky waters of uncertainty effectively.
Tools and Software for Solver Analytics
In the realm of solver analytics, selecting the right tools and software can make or break an organization's analytical capabilities. With a myriad of options available, understanding the landscape of these tools is crucial. Not only do the right tools streamline processes, they can also enhance the quality of insights derived from data. Businesses today operate in increasingly complex environments, and the ability to analyze data effectively is paramount for making informed decisions. This section will delve into popular tools used in the field and discuss the benefits and considerations between cloud-based solutions and on-premises software.
Overview of Popular Tools
When it comes to solver analytics, there’s no shortage of software solutions designed to meet various analytical needs. Some notable tools include:
- Microsoft Excel: A classic yet powerful tool. Excel's Solver feature is widely used for optimization problems, allowing users to build models and solve linear programming issues relatively easily.
- IBM ILOG CPLEX: Known for its robustness and optimization capabilities, CPLEX handles complex problems efficiently, making it a go-to solution for large-scale operations.
- Apache Spark: Especially valuable in big data analytics, Spark provides a framework for data processing that can be integrated with machine learning algorithms for predictive analytics.
- MATLAB: Offers strong mathematical modeling capabilities. Users can create solver routines tailored to specific needs, making it versatile for various industries.
- Gurobi: This optimization solver excels in handling large-scale mathematical programs and offers advanced algorithms for both linear and integer programming.
These tools vary in their complexity, cost, and the specific needs they address. The right choice will ultimately depend on the organization's goals, size, and resource availability.
Cloud-Based Solutions vs. On-Premises Software
With the shift toward digitization, businesses are often faced with the choice between cloud-based solutions and on-premises software. Understanding the pros and cons of each can assist in making an informed decision.
Cloud-Based Solutions
- Flexibility and Accessibility: Cloud solutions like Google Cloud and Microsoft Azure allow users to access tools from anywhere. This flexibility is beneficial for teams that are remote or distributed.
- Scalability: As companies grow, cloud solutions generally offer easier scalability, enabling organizations to add or remove resources as needed without significant infrastructure changes.
- Cost-Effectiveness: These solutions often employ a subscription model, reducing upfront costs, although ongoing fees can add up over time.
- Automatic Updates: Users benefit from the latest features and security without additional effort.
On-Premises Software
- Customization: On-premises software may allow deeper customization for specific operational needs. Businesses can tailor installations to fit their unique requirements.
- Data Control: Organizations that handle sensitive data often prefer on-premises solutions for full control over their data security and compliance.
- Long-Term Cost: While the initial investment can be higher, long-term costs may be lower since many don’t involve subscriptions.
- Stability: Businesses may find on-premises solutions more stable, given they don’t rely on internet connectivity and external servers.
Ultimately, the choice between cloud-based and on-premises software involves balancing flexibility, security, and cost considerations.
"Selecting the right analytics tools is not just about what features they offer; it’s about understanding how they align with business strategies and operational needs."
Challenges in Implementing Solver Analytics
Unlike a smoothly running engine, implementing solver analytics is often more akin to tuning an old car; it requires effort, precision, and the right components. The deployment of these analytical tools presents various hurdles, notably because the underlying success of solver analytics hinges not just on capability, but on the quality and approach taken by the people and systems involved. Thus, understanding these challenges is essential for any organization looking to leverage solver analytics for strategic advantage.
Data Quality Concerns
One of the primary impediments in the realm of solver analytics is data quality. When one considers that data serves as the backbone of any analytical endeavor, it becomes evident that poor quality can lead to misguided decisions and misinterpretations. An example of this can be found in healthcare analytics, where flawed data can compromise patient treatment plans and operational efficiencies. Lackluster data can stem from numerous sources:
- Human Error: Entering wrong values or overlooking entries can skew results.
- Inconsistent Data Formats: Variability in data collection methods leads to inconsistencies, making it difficult to draw reliable conclusions.
- Outdated Information: Using obsolete data fails to capture current conditions, leading decision-makers to a game of catch-up.
To effectively address these concerns, organizations need robust data governance policies. Regular audits of data quality, employing data cleaning methods, and establishing standardized formats across departments can significantly enhance the overall analytics output.
"Quality over quantity" rings particularly true in the context of solver analytics, where a handful of accurate, well-maintained data points can be more valuable than vast numbers of poor-quality entries.
Complexity of Models
Another significant hurdle arises from the complexity of the models used in solver analytics. While sophistication often brings about enhanced precision, it can also lead to a web of confusion if not managed properly. The mathematical models utilized—be they linear programming, simulation techniques, or others—can become intricate, causing difficulties in understanding and application.
Consider a multi-faceted supply chain optimization model that accounts for production rates, shipping times, and storage costs. Such a model may yield fantastic outputs, but only if the operator has the proficiency to decode these outputs into actionable strategies. This intricacy requires a skill set not only in mathematics but in domain-specific knowledge as well. Miscalculations stemming from misunderstood models can result in greater losses than they resolve.
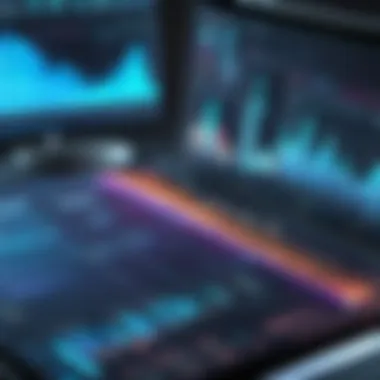
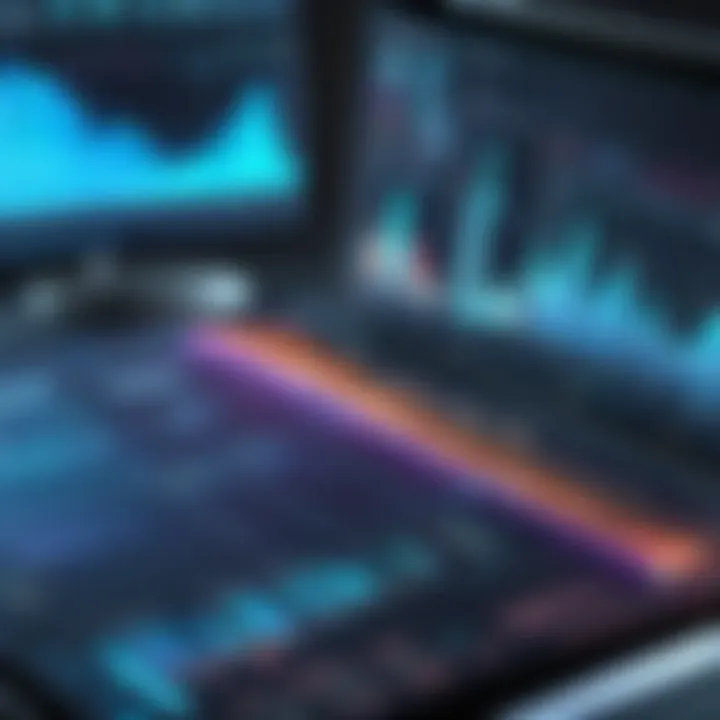
To navigate through this complexity, it’s vital that businesses invest in continuous education and training for their teams. Workshops, online courses, and mentoring programs can equip professionals and students alike with the necessary tools to handle advanced analytical models. Emphasizing a culture of learning for growth is not merely beneficial; it’s a necessity in the fast-evolving domain of solver analytics.
Future Trends in Solver Analytics
As businesses increasingly rely on data to inform their decision-making processes, the field of solver analytics is poised for significant transformation. This section delves into emerging trends that underscore the evolution of solver analytics, emphasizing the value this evolution brings to enterprises looking to stay ahead in competitive markets. The integration of advanced technologies, particularly artificial intelligence and machine learning, paves the way for new methodologies that enhance the functionality and application of solver analytics. Understanding these trends is crucial for professionals aiming to harness their potential effectively.
Integration with AI and Machine Learning
In the rapidly changing landscape of data analytics, the infusion of AI and machine learning technologies is at the forefront of future trends within solver analytics. These advanced technologies enable more robust data analysis, transforming how problems are modeled and solutions are derived. By leveraging large datasets and complex algorithms, AI can help refine decision-making processes far beyond traditional programming methods.
Consider a retail company managing its inventory through solver analytics. Integrating machine learning allows the development of predictive models that analyze past sales data, customer preferences, and seasonal trends. It goes beyond merely offering optimal stock levels and suggest tailored inventory strategies that adjust dynamically based on real-time data. This not only enhances operational efficiency but also significantly boosts customer satisfaction by ensuring products are available when needed.
A few key points regarding this integration are:
- Real-Time Data Processing: AI empowers solver analytics to make on-the-fly decisions by processing incoming data continuously.
- Pattern Recognition: Advanced algorithms recognize patterns in vast data that human analysts might overlook, leading to deeper insights.
- Automation of Routine Tasks: By automating repetitive processes, human resources can be allocated to more strategic planning tasks.
As analytics technologies continue to evolve, the groundwork laid today sets the stage for unimaginable advancements in decision-making effectiveness.
Adaptive Algorithms
Another notable trend shaping the future of solver analytics is the emergence of adaptive algorithms. These algorithms move away from a one-size-fits-all approach, adapting their parameters based on the specific context and nuances of the datasets they analyze. This adaptability is paramount for businesses facing fluctuating conditions, as it allows for more responsive and tailored solutions.
For instance, in finance, a financial institution could implement adaptive algorithms to manage its portfolio. As market conditions change, the algorithms can adjust risk assessments and investment strategies accordingly. This means that rather than relying on outdated models, the institutions utilize current data and trends, leading to better-informed investment decisions.
The impact of adaptive algorithms can be seen in several areas:
- Enhanced Flexibility: Capable of adapting to new information without needing complete model overhauls.
- Increased Accuracy: Continuous learning improves models’ predictive accuracy, allowing businesses to navigate uncertainties more effectively.
- Better Resource Allocation: With a precise understanding of changing dynamics, companies can allocate resources more effectively to meet operational needs.
In summary, the future of solver analytics is rich with potential, particularly through the incorporation of AI, machine learning, and adaptive algorithms. These trends not only promise to streamline processes and drive efficiencies but also assure robust strategic decision-making capabilities. It's essential for professionals in IT and related fields to stay informed about these emerging trends, as they represent critical tools in maintaining relevance and competitiveness in an ever-evolving landscape.
Case Studies of Successful Solver Analytics Implementation
Case studies offer a unique window into the real-world applications of solver analytics, illuminating how various organizations leverage these techniques to solve complex problems and enhance decision-making processes. This section emphasizes the significance of understanding these implementations by showcasing the tangible benefits and lessons learned. Analyzing successful cases not only provides insights into effective strategies but also serves as a litmus test for the adaptability of solver analytics across sectors.
Industry Leaders and Innovators
In today’s fast-paced business environment, staying ahead of the curve often hinges on the implementation of cutting-edge technologies like solver analytics. Let's take a look at a few industry leaders who have mastered these tools:
- Amazon: The e-commerce giant utilizes sophisticated solver models for optimizing warehouse logistics. By analyzing sales forecasts and inventory data, they improve delivery times and reduce operational costs. For example, during peak seasons, Amazon applies predictive analytics to anticipate demand, ensuring that they have the right products at the right time in their vast network of warehouses.
- Tesla: They apply solver analytics in manufacturing processes to streamline production schedules and minimize waste. By implementing simulation-based techniques, Tesla can test various production scenarios virtually before using resources in real-world settings. This not only enhances efficiency but also allows the company to swiftly adapt to market changes.
- Procter & Gamble: This consumer goods company employs solver analytics for product development and marketing strategies. By utilizing prescriptive analytics, they can model potential outcomes of various marketing strategies and product launches, helping them identify the most effective approaches before full-scale implementation.
These examples underline that the effective use of solver analytics can lead to significant competitive advantages, whether through enhanced operational efficiency or improved marketing strategies.
Lessons Learned from Failures
While the stories of triumph are compelling, it’s crucial to dissect the lessons from failures in solver analytics to fully understand its pitfalls and limitations. Here are some important insights:
- Overreliance on Data: Some organizations mistakenly treat data as infallible. A case in point is a retail company that relied heavily on historical sales data without accounting for changing consumer trends, leading to inventory mismanagement. Understanding that data should be complemented with market insights is vital.
- Lack of Stakeholder Involvement: Another common mistake is the exclusion of key stakeholders in the implementation process. A financial institution implemented a solver model that did not align with actual operational practices, resulting in confusion and pushback from employees. Including staff feedback in the development phase can enhance model usability and acceptance.
"Failure doesn't mean you are a failure; it just means you haven't succeeded yet."
- Inadequate Training: When a manufacturing firm rolled out a complex solver tool without proper training, workers struggled to utilize the software efficiently, resulting in decreased productivity. Investing in training ensures that team members are confident in leveraging solver analytics tools effectively.
These lessons learned reinforce that even leaders can stumble, highlighting the importance of combining analytical tools with thoughtful implementation strategies. Understanding these dynamics can pave the way for more informed and prudent decisions in future projects.
The End
In wrapping up our exploration into solver analytics, it's pertinent to recognize not just how vital these tools are, but also their broad impact on strategic decision-making in diverse sectors. This article has laid out the foundational elements, methodologies, and future directions of solver analytics, offering crucial insights for all stakeholders involved.
Summary of Insights
Through this journey, we have seen that solver analytics serve as a robust framework for enhancing business efficiency. They enable organizations to transform chaotic data into actionable insights, allowing for better forecasting, resource allocation, and risk management.
Key Takeaways:
- Integration of Data and Decision Making: Solver analytics harmonize vast datasets with analytical models, creating tools that provide clarity where uncertainty exists.
- Applications Across Verticals: From supply chain optimizations to financial modeling, the applications of solver analytics demonstrate versatility in addressing sector-specific challenges.
- Future Relevance: The merging of AI with traditional analytics paves the way for more adaptive algorithms, suggesting a dynamic evolution in how businesses might approach problem-solving.
"Data is the new oil, but without a good solver analytics tool, it remains untapped potential."
The Path Forward
As we peer into the horizon of solver analytics, the opportunities only multiply. The integration of machine learning and artificial intelligence with solver tools will enable businesses to navigate uncharted waters with greater agility.
Future Considerations:
- Emphasis on Education and Skills Development: As technology advances, ensuring stakeholders are equipped with the necessary knowledge is paramount. Training programs that enhance skills in using these analytics tools will be crucial for organizations aiming for competitive advantages.
- Continuous Improvement of Data Quality: Businesses must prioritize data integrity, as clean data will lead to more reliable outcomes from solver models. This is not a one-time effort, but an ongoing commitment.
- Exploration of Emerging Technologies: Keeping an eye on advancements in related fields will help organizations remain ahead of the curve. Technologies like quantum computing, for instance, may radically change the capabilities of solver analytics in the near future.
In essence, the journey of adopting and refining solver analytics is ongoing and vital for sustained success. As organizations continue to embrace this analytical revolution, the potential for enhanced operational excellence and strategic insight is limitless.