Methods and Applications of Social Media Data Analysis
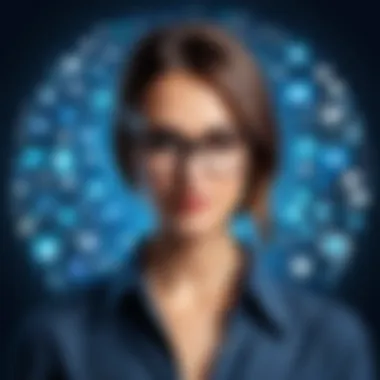
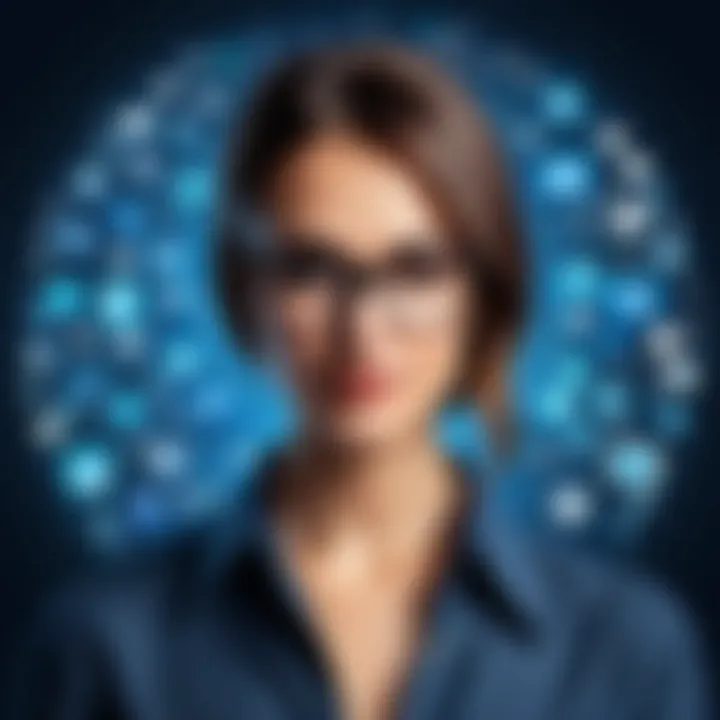
Intro
In a world where information flows faster than a speeding bullet, the ability to analyze social media data becomes paramount. Social media platforms like Facebook, Twitter, and Reddit host an ocean of opinions, trends, and interactions. Diving into this data can yield invaluable insights that inform decision-making across various sectors.
Understanding how to navigate the landscape of social media data analysis equips professionals and students alike with skills for the digital age. From corporations trying to gauge consumer sentiment to researchers exploring social dynamics, the implications of effectively analyzing this data are vast.
As we traverse through this exploration, we will unravel the methods employed in social media data analysis, examine real-world applications, and confront the ethical considerations that arise in this complex milieu. By the end of our journey, readers will gain a nuanced understanding of the dynamics at play in social media interactions.
Features and Capabilities
Overview of Key Features
Social media data analysis tools come equipped with a variety of features that optimize the way data is collected, analyzed, and visualized. Key features include:
- Data Collection: The ability to gather tweets, posts, comments, and likes from various platforms, allowing a comprehensive overview of public sentiment.
- Sentiment Analysis: Utilizing Natural Language Processing (NLP) to determine the emotional tone behind communications, whether positive, negative, or neutral.
- Trend Identification: Uncover patterns in the data that may indicate emerging trends, hot topics, or shifts in public interest.
- User Profiling: Creating profiles based on user behavior and engagement, offering insights into demographics, preferences, and behaviors.
User Interface and Experience
The design and usability of data analysis tools can make or break user engagement. An intuitive user interface that provides a seamless experience is crucial. Users expect easy navigation, drag-and-drop functionalities, and visually appealing dashboards that translate data into easily digestible formats.
Elements such as interactive graphs and customizable reports enhance the overall user experience. Moreover, offering features like real-time data feeds can make a significant difference in staying updated with the latest social media happenings.
Performance and Reliability
Speed and Efficiency
In the fast-paced realm of social media, speed is of the essence. Analysis tools that process and deliver insights quickly can place organizations a step ahead. Optimal performance means handling large data sets without significant lag or downtime.
Downtime and Support
Reliability is an indicator of a tool's quality. Users seek consistent performance, meaning that tools should have robust supportive frameworks in place in case issues arise. It’s crucial to consider providers that offer strong customer support, frequent updates, and proactive maintenance to ensure a smooth experience.
To wrap it up, the features and overall performance of social media data analysis tools play a vital role in shaping how organizations and individuals interpret data. The right mix of user experience, capability, and reliability builds a solid foundation for informed decision-making.
"Social media is not just a channel; it’s a treasure chest of insights waiting to be unlocked."
As we delve deeper into this rich vein of information, let's proceed to examine the methodologies used in social media data analysis.
Preamble to Social Media Data Analysis
In an age where social media is central to communication, entertainment, and information exchange, understanding social media data analysis becomes paramount. This area of study isn't just an academic pursuit; rather, it holds practical significance for businesses, policymakers, and everyday users alike. The sheer volume of data generated across platforms is staggering. Each tweet, post, like, and share contributes to a vast ocean of information waiting to be interpreted. Thus, having a grasp on how to analyze this data can unveil insights that drive decisions in multiple domains.
Defining Social Media Data
Social media data encompasses various forms of user-generated content and interactions from platforms such as Facebook, Twitter, Instagram, and Reddit. It includes text, images, videos, and other multimedia formats, as well as metrics reflecting user engagement. These data points can be categorized broadly into qualitative and quantitative types.
- Quantitative data might involve the number of shares, likes, or comments a post receives. These metrics can quantify a user's reach and influence.
- Qualitative data, on the other hand, often seeks to capture the sentiment behind posts and comments, revealing how users feel about a particular topic or brand.
Understanding this distinction is crucial. Marketers need to analyze not just how much content is being generated but also its quality; not just the number of interactions, but the nature of those interactions. This dual approach allows for a more comprehensive picture of user behavior and sentiment.
The Importance of Data Analysis in Social Media
Data analysis in social media serves numerous purposes, playing an integral role in decision-making across different fields. Businesses utilize insights gleaned from their social media activities to make data-driven marketing decisions. For instance:
- Analyzing customer feedback helps refine products or services.
- Sentiment analysis over time can gauge brand health.
- Identifying trends can help forecast consumer demand.
Moreover, social media analysis isn't only for businesses. Political campaigns leverage these insights to understand public opinion, tailoring messages and responses to resonate more effectively with constituents. In the realm of crisis management, organizations can monitor social media to identify negative sentiment and address issues before they escalate.
Ultimately, as data becomes increasingly central to strategy in various domains, learning how to effectively analyze social media data will empower more informed, responsive, and adaptable decision-making processes in a rapidly evolving technological landscape.
"In the digital realm, data is the new currency, and understanding its value can lead to unparalleled opportunities."
By delving into these essential elements, the article aims to illuminate the importance of social media data analysis, drawing connections between methods, real-world applications, and the implications of advanced analytics practices.
The Evolution of Social Media Data Analysis
The evolution of social media data analysis provides crucial context for understanding how we interpret and leverage data from online platforms today. This evolution transcends a mere historical glimpse; it touches upon significant shifts in technology, methodologies, and perceptions regarding the importance of social media metrics for businesses, governments, and individuals alike. As the web continues to expand and adapt, so too does the necessity for refined tools to make sense of vast data sets. Understanding this journey facilitates a deeper awareness of the data's role in shaping marketing strategies, public opinion, and even social movements.
Historical Perspectives

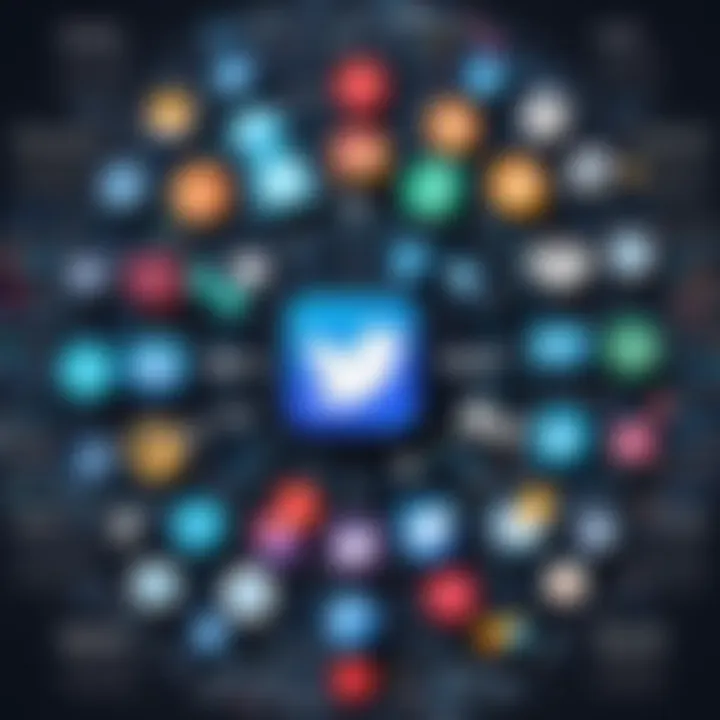
The roots of social media data analysis can be traced back to the early days of online communication platforms. Initially, metrics were rudimentary, focusing on basic engagement statistics like page hits and bounce rates. As platforms like MySpace and early iterations of Facebook emerged, a paradigm shift began to take shape.
In the late 2000s, social media began to infiltrate daily communication, and suddenly, companies started to recognize the treasure trove of user-generated content. Firms began to employ scrapers and bots to harvest public posts, comments, and overall user interactions. This was a game changer, transforming raw social interactions into quantifiable data.
Key moments in the historical development include:
- The launch of Facebook in 2004, leading to a significant increase in user interaction and data availability.
- The creation of Twitter in 2006, introducing new dimensions of real-time data extraction.
- The advent of various analytical tools in the 2010s, making it easier to process large amounts of social data from multiple sources.
These early developments laid the groundwork for more sophisticated methods employed today. People started to realize that the sentiments expressed on these platforms could serve as a barometer for consumer behavior and trends.
"The wealth of real-time data available on social media platforms is akin to holding a mirror to society, reflecting sentiments, emotions, and shifting trends."
Technological Advances Driving Change
The rapid technological advancements that have occurred since the inception of social media have significantly influenced data analysis methodologies. Initially, analysts relied on simple statistics and manual methods to interpret data, which was labor-intensive and often flawed by human error.
As technology progressed, developments in natural language processing (NLP), machine learning, and big data analytics began to transform the landscape. Here are a few pivotal technological influences:
- Natural Language Processing (NLP): This technology allows for the analysis of user-generated text, facilitating sentiment and opinion analysis at a scale never thought possible.
- Machine Learning Algorithms: These create models that can predict user behavior based on historical data, offering valuable insights for businesses hoping to tailor their marketing strategies.
- Cloud Computing: Enhanced data storage capabilities have allowed companies to store and analyze vast volumes of social media data affordably.
These innovations have not just improved the speed and efficiency of data analysis; they have dramatically shifted the types of questions researchers can ask. Analysts can delve deeper into complex layers of data, unearthing patterns and behaviors that drive engagement and loyalty.
To close the loop, the evolution of social media data analysis is an ongoing journey. As we move forward, the continuous interplay between technological advancements and analytical methodologies will surely define both the capabilities and limitations of our social media insights.
Key Techniques in Data Analysis
Data analysis in social media is not just about crunching numbers or gathering vague information. It’s about discerning patterns, understanding behaviors, and drawing insights that can shape strategies. In this section, we’ll look closely at some essential techniques that inform how we approach data analysis in platforms where millions converse daily. Mastery over these methods is critical, especially when navigating the vast ocean of social media data.
Quantitative vs. Qualitative Analysis
Quantitative analysis and qualitative analysis each holds unique strengths, and understanding the difference can lead to more accurate interpretations.
Quantitative Analysis focuses on numerical data. Metric-based information, like engagement rates, follower counts, or click-through rates, provides a bird's-eye view of trends and can easily be visualized using graphs or charts. This is often useful for businesses aiming to forecast performance or measure impact accurately. On the flip side, qualitative analysis digs deeper into contextual details, examining human behavior, opinions, and feelings conveyed in posts and comments. Sentiment analysis falls within this bracket, revealing how users feel about a brand or topic.
Some benefits to consider:
- Quantitative Data
- Qualitative Data
- Provides measurable evidence for decision-making.
- Facilitates comparative analysis over time.
- Enables quick synthesis of large datasets.
- Offers insights into user motivations and attitudes.
- Helps to contextualize quantitative metrics for richer understanding.
- Illuminates the ‘why’ behind trends observed in the numbers.
Both approaches are vital; depending on the question at hand, one might shine more than the other. Integrating both can ensure a well-rounded analysis.
Sentiment Analysis
Sentiment analysis is the crown jewel of qualitative analysis in social media data. It's about gauging the public’s mood or emotional reaction towards something, whether that's a product, service, or political issue. Using natural language processing, sentiment analysis tools can sift through user-generated content to categorize sentiments as positive, negative, or neutral.
Implementing sentiment analysis can yield profound insights:
- Consumer Behavior Understanding: The vibes around your brand can change considerably after a product launch. Pinpointing these shifts swiftly can aid in adjustments.
- Crisis Management: Quickly recognizing negative sentiment can help mitigate damage during a PR crisis before it escalates. For instance, if countless users voice frustration following a launch, grasping emotions early on allows the brand to pivot or respond.
- Influencer Collaboration: Analyzing sentiments towards influencers can influence partnership decisions. It’s beneficial to collaborate with those who not only align strategically but also resonate positively with audiences.
Moreover, tools like MonkeyLearn and Lexalytics can serve as crucial supports in implementing this analysis, offering user-friendly interfaces for nuanced interpretation.
Network Analysis
Network analysis is a less traditional yet incredibly insightful technique. It involves studying the connections and interactions that occur on social media platforms, mapping out the relationships between users and the content they share. By examining these networks, analysts can identify key figures in a community, understand information flows, and even uncover hidden groups.
This technique can yield several practical applications:
- Influencer Identification: Recognize who the main players are in your niche. Analyzing connections aids in spotting whose voices carry the most weight.
- Community Detection: Understanding clusters or communities within platforms can tailor marketing strategies or content creation. Do certain clusters largely discuss specific topics? Tailoring communication towards them can enhance relevance.
- Tracking Information Spread: Evaluate how information spreads through networks. This can shine a light on both effective and ineffective messaging.
"Connections built in social platforms can provide a treasure trove of insights examined rightly."
By leveraging network analysis thoughtfully, organizations can better understand their social media landscape, yielding greater returns on their analytical efforts.
Tools and Technologies for Social Media Data Analysis
Social media has transformed into a vast ocean of data, teeming with valuable insights waiting to be uncovered. The importance of tools and technologies in analyzing this data cannot be overstated. They serve as the bridge connecting our questions to actionable insights and decision-making. In this section, we will dive deeper into data collection tools, analysis software, and visualization tools, highlighting their roles and relevance in social media data analysis.
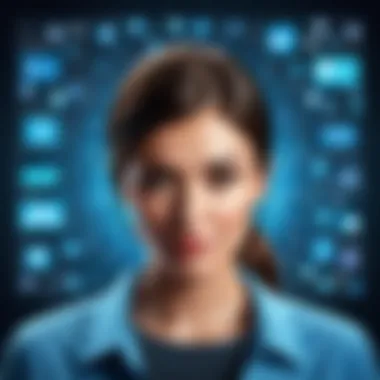
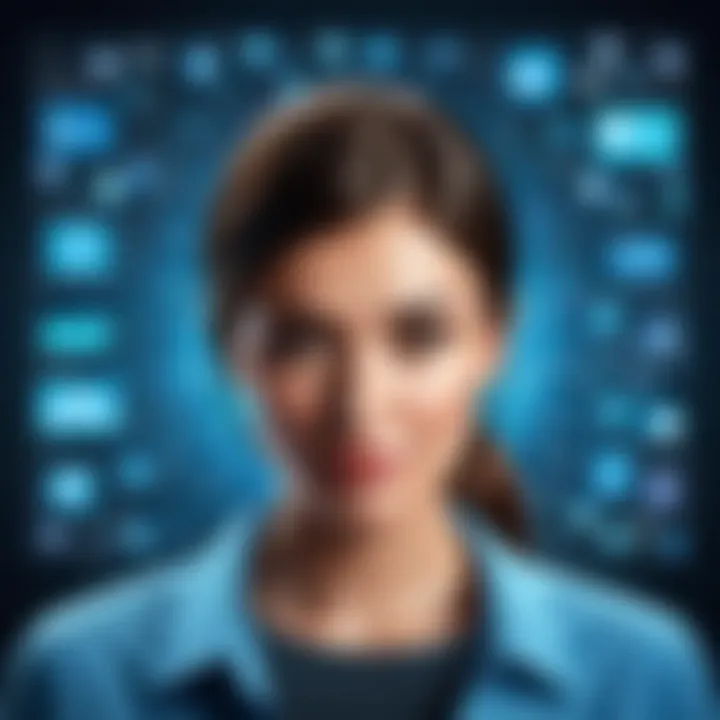
Data Collection Tools
Data collection is the foundational step in any analysis. Without the right data collection tools, the entire analysis process can be like building a house on sand; it lacks the sturdiness required for meaningful outcomes. Various tools allow data collection from social media platforms, be it Facebook, Twitter, or Instagram.
The role of these tools is to automate and simplify the gathering of data at scale. Some notable examples include:
- Hootsuite: This tool not only helps in managing multiple social media accounts but also aids in gathering data analytics across platforms.
- Brandwatch: It’s a powerful tool for monitoring brand mentions and social conversations, providing insight into what users say about a certain brand.
- Scrapy: An open-source spider framework that can be customized for web scraping, making it a good fit for precise means of data harvesting.
These tools also typically come with functionalities to schedule data collection, ensuring that insights are gathered in real-time. Nothing beats getting information as events unfold, allowing for immediate response, and enhancing situational awareness.
Analysis Software
Once the data is collected, it needs to be analyzed to derive actionable insights. Analysis software is crucial in this phase, as it equips users with the necessary tools to sift through vast datasets.
Different software options have their unique strengths:
- R: This programming language is famed for statistical analysis. It provides a great suite of packages ideal for social media data analytics, supporting complex calculations.
- Python: Another widely used language; its libraries, such as Pandas and NumPy, allow for efficient data manipulation and analysis.
- Tableau: While primarily a visualization tool, Tableau also offers analysis capabilities that let users query and delve into data easily.
Moreover, analysis software must include functionalities that facilitate exploratory data analysis and predictive analytics. Such software provides an edge in pinpointing trends and making accurate projections based on past social media behavior.
Visualization Tools
Data visualization is often the final yet essential piece of the puzzle. Presenting findings in a visually compelling way makes complex data easier to understand. This part of the analysis completes the journey, transforming raw data into formats that are digestible for stakeholders who may not have technical expertise.
Some widely acknowledged visualization tools are:
- Microsoft Power BI: This tool makes it easy to create interactive visualizations and dashboards, making the analysis more engaging.
- Google Data Studio: A free tool from Google that allows users to turn their data into informative, easy-to-read, easy-to-share, and fully customizable dashboards.
- D3.js: A JavaScript library for producing sophisticated data visualizations in web browsers, providing designers with extensive visualization flexibility.
Sometimes, the right visualization can be more illuminating than pages of text. Data becomes a narrative, highlighting key trends and patterns that may otherwise go unnoticed.
"Data isn’t just numbers; it’s the story we tell through analysis and visualization."
Data Privacy and Ethical Considerations
In an age where social media pervades everyday life, privacy and ethics are not just buzzwords; they are paramount. As businesses and organizations harness social media data for various analytical pursuits, the challenges of navigating the complex landscape of user privacy and ethical standards come to the forefront. These considerations are essential, not only for compliance with regulations but also for maintaining trust and integrity among users. The interplay between data collection and user consent shapes the effectiveness and legitimacy of social media data analysis itself.
User Consent and Privacy Policies
User consent forms the bedrock of ethical data usage. It’s not just about getting a tick box at the bottom of a page but understanding what users are agreeing to. Policies must be transparent, informative, and accessible, ensuring users know how their data will be used and stored. Here’s why this is crucial:
- Trust Building: When users feel their privacy is respected, trust flourishes, leading to open engagement.
- Legal Responsibilities: Many regions have stringent laws surrounding data privacy, such as the GDPR in Europe, obligating businesses to obtain explicit consent before data collection.
Privacy policies ought to be written in a clear, straightforward language, steering clear of legal jargon that can alienate readers. By demystifying these policies, organizations can foster a more informed user base while enhancing their own reputations.
"People don’t mind sharing their data when they see the value they get in return, but their concerns ramp up when consent feels hidden or forced."
The Role of Ethics in Data Manipulation
Ethics in data manipulation is another layer that demands attention. The power of social media analytics lies in its ability to reveal patterns and trends that can significantly influence decision-making. However, with great power comes great responsibility. Misusing data—either through selective reporting or manipulation—can lead to skewed insights, ultimately affecting stakeholders and the general public.
Some key ethical considerations include:
- Transparency of Methods: It is vital that the methods used for analysis are clear and justifiable. Users and stakeholders should understand how conclusions were reached.
- Data Integrity: Ensuring the accuracy of data used in analysis helps prevent misleading outcomes. Misrepresenting data can not only damage credibility but also have repercussions on public opinion and policy making.
Addressing these issues thoughtfully creates a safer digital environment, fostering a culture of ethics in data analysis. The path forward involves not just complying with laws but also upholding ethical standards that respect user rights. Effective data analysis is not simply about numbers; it’s about the people behind the data.
Challenges in Social Media Data Analysis
In the ever-evolving landscape of digital communication, social media data analysis presents a unique set of challenges that professionals must navigate. Understanding these challenges is not just important for ensuring accurate data interpretation, but also for leveraging this information effectively. Social media platforms generate vast amounts of data daily, making it crucial for researchers and organizations to recognize the potential hurdles. This section dives into some of the primary difficulties faced by practitioners in the realm of social media data analysis.
Data Overload
When it comes to social media data, one common issue is the sheer volume. Think about it; millions of users generate posts, replies, images, likes, shares, and videos every minute. Filtering through this ocean of content to extract meaningful insights can feel like finding a needle in a haystack.
- Volume: The amount of data is staggering and can overwhelm analysts. Every platform has its unique set of metrics—Facebook's likes and shares, Twitter's retweets, Instagram's comments, and so on. Just trying to make sense of it all can lead to analysis paralysis.
- Noise: Amidst the valuable insights lies a mountain of irrelevant noise—spam, bots, and off-topic posts can cloud the analysis. Determining what data is genuinely useful becomes challenging.
To manage data overload, organizations might turn to advanced filtering techniques and machine learning algorithms to categorize and prioritize data streams. This kind of strategic planning can help streamline the process and make data analysis more manageable.
Bias in Data Interpretation
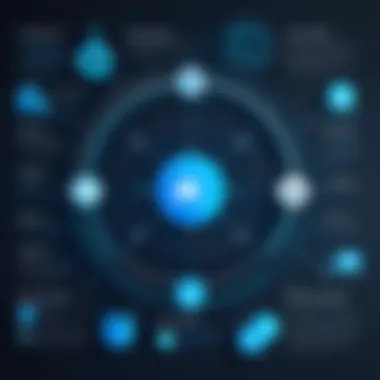
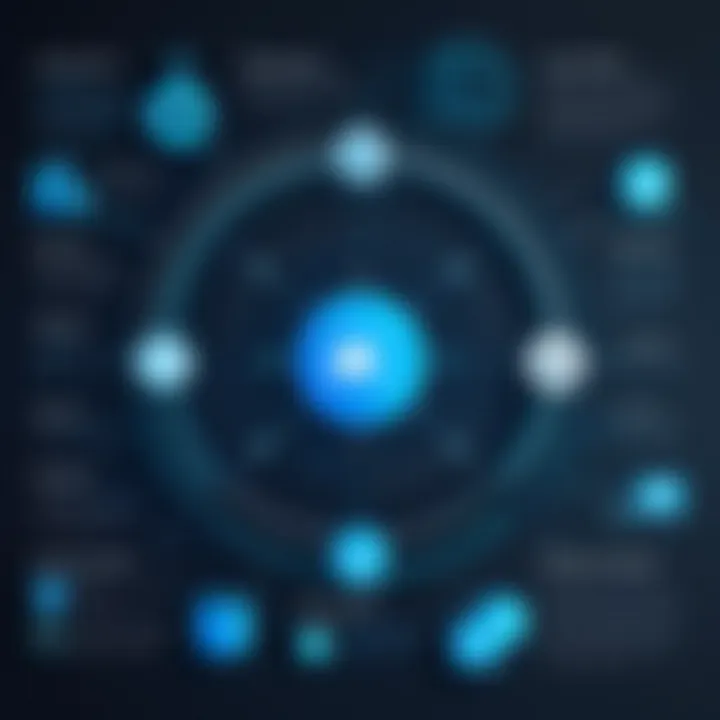
Just like a ship can be thrown off course by a slight miscalculation, bias in data interpretation can lead analysts astray. This problem is particularly prominent in social media, where various factors influence perceptions and results.
- Selection Bias: If an analysis only considers data from certain demographics or platforms, the results may not accurately represent the broader audience. This skew can yield insights that are far from reality, leading to misinformed decisions.
- Confirmation Bias: Analysts may unwittingly focus on data that supports their pre-existing beliefs while overlooking contradictory information. This tendency can distort the narrative and undermine the overall analysis.
Being aware of these biases is crucial. A more diverse data set and employing robust statistical methods can mitigate some of these issues, leading to a more balanced interpretation of social media trends.
Rapidly Changing Platforms
Social media is notorious for its fast pace—what's trendy today may be stale tomorrow. This fluidity poses unique challenges to analysts trying to keep up with the latest trends and features.
- Platform Updates: Frequent algorithm changes can affect how data is presented and what content gains visibility. For instance, Facebook occasionally tweaks its news feed algorithm, affecting how posts are prioritized. Keeping track of these changes requires continuous learning and adaptation.
- User Behavior: Social trends can shift in an instant, influenced by events, social movements, or even viral challenges. Analysts must stay vigilant to keep their interpretations relevant and up-to-date.
Both organizations and individuals must cultivate the agility to adapt along with these platforms. Staying informed about changes and being ready to adjust strategies accordingly can significantly improve the effectiveness of social media data analysis.
"Understanding the challenges of social media data analysis is crucial for effective decision-making in an information-rich environment."
Applications of Social Media Data Analysis
The domain of social media data analysis extends far beyond mere trend spotting or content tracking. It encompasses diverse applications that permeate multiple sectors, enhancing how businesses and organizations interact with their audiences. Understanding these applications is pivotal in deploying data effectively, helping professionals leverage insights to make strategic decisions that drive growth and engagement. Through methods such as predictive analytics and social listening, companies gain invaluable information about consumer behavior, preferences, and even potential crises that loom on the horizon.
Marketing and Brand Management
Influencer Marketing
The nature of influencer marketing has transformed the way brands communicate with their target audience. By harnessing the power of personalities who have cultivated trust and rapport with their followers, brands can reach potential customers in an organic and authentic manner. This aspect stands as a significant strength of influencer marketing—its ability to blend marketing with personal recommendations. Therefore, it's not just about pushing a product; it's about aligning with a voice that resonates with the audience.
A unique feature of influencer marketing is its focus on niche markets. Rather than casting a wide net, brands can connect with micro-influencers who have a smaller but highly engaged following. This significantly lowers the risk of losing touch with the audience, as micro-influencers often cater to specific interests. However, one must tread carefully here; over-reliance on influencers can backfire if the influencer's values do not align with those of the brand.
Consumer Feedback
Consumer feedback is another gem in the toolkit of social media data analysis, wielding the capacity to reshape products and services based on real customer input. This dynamic two-way street not only promotes customer engagement but also cultivates a feeling of community around a brand. A notable characteristic of consumer feedback is its immediacy. In today’s fast-paced world, customers expect brands to react swiftly to their opinions, making it a crucial element of brand management.
What sets consumer feedback apart is its capacity for transparency. In an age where consumers are increasingly skeptical about marketing messages, authentic feedback from peers often holds more weight than traditional advertisements. However, while consumer feedback provides actionable insights, managing negative reviews is a double-edged sword—navigating this landscape requires finesse and strategic planning.
Political Campaigns and Opinion Polling
The rise of social media has drastically transformed the landscape of political campaigning and opinion polling. Decisions made behind the scenes in these spheres are often influenced by social media sentiment. Campaigns now analyze social media trends to gauge public sentiment, shape campaign messages, and even to anticipate reactions to policy proposals. Moreover, the power of social media to spur grassroots movements showcases how public opinion can shift rapidly, leaving traditional polling methods scrabbling to keep up.
Crisis Management
In crisis management, social media data analysis serves as an early warning system. Brands can identify emerging crises before they escalate, allowing swift corrective actions. Whether it’s tracking brand mentions or analyzing hashtag trends, timely data interpretation helps organizations respond effectively. The unique challenge here involves sifting through the noise to determine what data is significant. Ignoring vital information could lead to missteps, while overreacting could further inflame a situation. Thus, an analytical approach, coupled with a poised response strategy, is essential for effective crisis management.
Future Trends in Social Media Data Analysis
As we look to the horizon, the landscape of social media data analysis is on the brink of transformation. The use of data to draw insights and understand user behavior is moving at breakneck speed. The significance of understanding future trends cannot be overstated; they shape not only how businesses strategize but also how society interacts with content online. The interplay between technology and data is paramount, and failure to adapt could leave organizations in the dust.
AI and Machine Learning Integration
The infusion of AI and machine learning into social media data analysis heralds a new era. Leveraging these technologies enables analysts to sift through massive datasets with an efficiency that was unthinkable a few years ago. Algorithms can recognize patterns, predict trends, and glean insights from user interactions at lightning speed.
Additionally, machine learning models are advancing in their capacity to perform sentiment analysis, allowing companies to gauge public opinion in real-time. Rather than waiting for surveys or focus groups, businesses can access immediate consumer feedback based on what users post. This shift not only shortens response time but also enriches engagement strategies with tangible data.
"In a fast-paced digital environment, being proactive rather than reactive is increasingly crucial for success."
However, the implementation of AI isn’t without its hurdles. Concerns over data privacy and ethical standards loom large, pushing developers to ensure algorithms operate transparently and responsibly. Analysts must work hand-in-hand with ethicists to provide frameworks that respect user privacy while driving business goals. The interplay of legislation, such as the GDPR in Europe, means players in this field must stay alert and agile.
Emerging Social Media Platforms
As new social media platforms emerge—or existing platforms evolve—data analysts must stay anticipatory. Take TikTok, for instance. Unlike traditional platforms, TikTok capitalizes on short-form video content and its algorithm favors engagement based on user behavior rather than chronological posting. This new model shifts the way data is collected and interpreted.
Analysts need to rethink their strategies to capture user interactions on these platforms. The dynamism of trends like challenges or viral events can lead to sudden spikes in data that require immediate analytical approaches. In adapting to these platforms, it is crucial to incorporate unique metrics that reflect the underlying user behaviors instead of relying on historical data alone.
Additionally, platforms prioritizing privacy and user control could redefine data access. Future guidelines may prioritize consent or allow users to manage the type of data they share. This would necessitate a more ethical approach to data interpretation while challenging analysts to work creatively within new parameters.
Closure
Reflecting on the comprehensive exploration of social media data analysis, it becomes clear that this realm holds significant importance in today’s digital landscape. The evolution of technology has transformed the way individuals and organizations perceive data, making it a cornerstone for informed decision-making. In our discussion, several specific elements underscore the relevance of conclusion.
Firstly, key insights drawn from various methods demonstrate how robust data analysis can unveil trends and behaviors that were previously obscured. Not only does it enhance marketing strategies, but it also allows businesses to harness customer feedback in real-time. This agility can make the difference between leading the pack or falling behind in a competitive market.
Secondly, considerations around data privacy and ethics have been illuminated as critical points of discussion. As we venture further into an interconnected world, understanding the balance between data utilization and user privacy will become paramount. It’s no longer enough to simply analyze data; accountability is an essential element that must accompany every byte collected and interpreted.
Moreover, one cannot overlook the implications of challenges inherent within social media data analysis. The hurdles posed by data overload and bias in interpretation necessitate ongoing education and the development of novel approaches. These challenges are real, but they are not insurmountable, requiring both innovation and critical thinking from professionals in this field.
In summary, the field of social media data analysis is poised to evolve further. Its importance cannot be understated—it brings methodologies that enable deeper insights, guides ethical standards, and informs the research direction for the future. As it continues to grow and adapt, remaining responsive to technological changes and societal expectations will be vital.