Top Text Analysis Software: Features and Insights
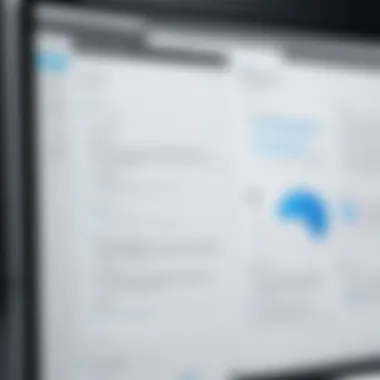
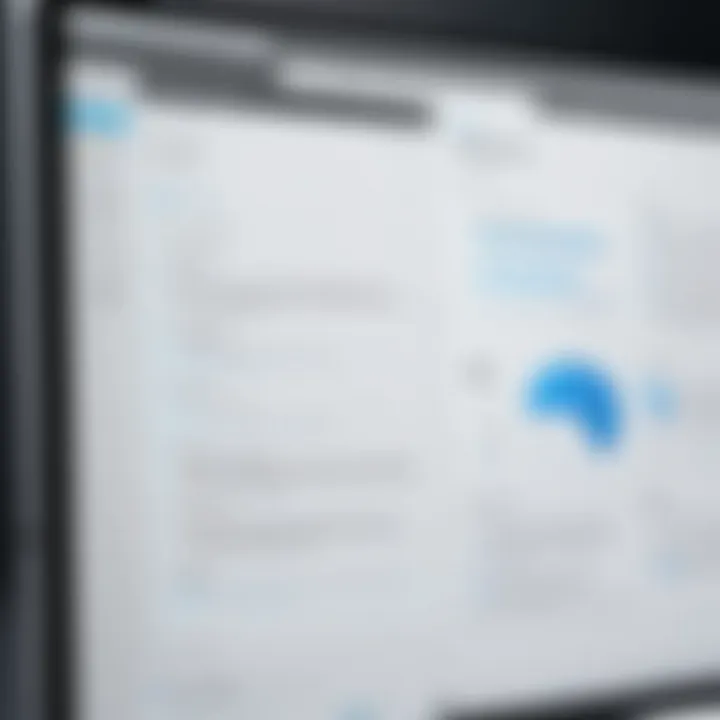
Intro
Text analysis software has become a critical tool for managing and deriving insights from the vast quantities of data generated in today's digital world. These tools facilitate the extraction of valuable information from unstructured text, allowing individuals and organizations to make data-driven decisions. As businesses and academics alike embrace the power of text analytics, it becomes essential to not only understand the functionalities of these tools but also to discern which options stand out in a crowded market.
This article aims to explore the key features, capabilities, performance metrics, and user experiences associated with various text analysis software solutions. We will examine how these tools cater to users with different needs, whether they are software developers diving deep into code, professionals in IT-related fields tackling complex projects, or students seeking to analyze texts for academic purposes.
Ultimately, this exploration seeks to equip readers with the knowledge to make informed choices when selecting a text analysis tool that aligns with their specific requirements.
Features and Capabilities
Understanding the features and capabilities of text analysis software is paramount for effective utilization. The following sections examine what these programs bring to the table, offering insight into their strengths.
Overview of Key Features
Text analysis software typically incorporates a range of core functionalities that enhance user experience and increase analytical depth. Among these are:
- Natural Language Processing (NLP): This technology allows the software to comprehend human language, enabling it to perform tasks such as sentiment analysis and entity recognition.
- Data Visualization: A strong text analysis tool often includes visual representation features, which help users interpret large data sets more intuitively.
- Integration Capabilities: The ability to integrate with other tools or platforms is critical. Many leading software options offer APIs or plugins to facilitate this functionality.
- Reporting Tools: These features enable users to generate comprehensive reports based on the analysis conducted, streamlining the communication of findings.
- Customization Options: Tailoring the analysis process to specific needs or preferences can greatly enhance the user's experience and effectiveness.
User Interface and Experience
A user-friendly interface is essential for maximizing the effectiveness of text analysis software. A well-designed UI fosters productivity and helps users to navigate through complex functionalities. Key aspects of a pleasant user experience include:
- Intuitive Design: Layouts that promote ease of use boost efficiency.
- Training Resources: Comprehensive guides or tutorial videos can quickly bridge the knowledge gap for new users.
- Community Support: Active forums or user communities allow for troubleshooting and shared knowledge.
Performance and Reliability
Performance critically influences the usability of text analysis tools. There are specific aspects to consider when evaluating how well these solutions perform in real-world scenarios.
Speed and Efficiency
The speed of text processing is particularly vital for users handling large data sets. Efficient software should be capable of:
- Processing text in a timely manner, minimizing wait times.
- Supporting batch processing to handle multiple documents simultaneously.
- Delivering results quickly, particularly in high-demand environments.
Downtime and Support
Reliability is also a fundamental concern. Uptime should be maximized to ensure productivity. In addition,
- Customer support: Access to knowledgeable support staff can help users resolve issues promptly.
- Documentation Quality: Clear and thorough documentation improves the self-sufficiency of users, reducing reliance on direct support.
Choosing the right text analysis software involves considering both features and performance. Each user will find different aspects more relevant according to their particular needs.
Understanding Text Analysis Software
Text analysis software serves a crucial role in the processing and interpretation of textual data. These tools offer diverse functionalities, driving insights from unstructured text, which is abundant in today's digital landscape. As organizations and researchers amass large volumes of text-based data, understanding the nuances of text analysis software becomes paramount.
Definition and Purpose
Text analysis software refers to applications designed to analyze, process, and extract meaningful information from textual content. The primary purpose of these tools is to transform raw text into structured data that is interpretable and actionable. They employ various techniques such as natural language processing (NLP), machine learning, and statistical analysis to dissect text, identify trends, and summarize findings. This enables users to derive insights that are often hidden within large text corpora. Effective text analysis supports informed decision-making by enabling users to understand sentiments, topics, and other key attributes of the data.
Importance in Data Science
In the context of data science, text analysis software is essential for dealing with the vast amounts of unstructured data, estimated to constitute approximately 80% of the data available today. Data scientists leverage these tools for tasks like sentiment analysis, topic modeling, and text categorization. By analyzing textual data, organizations can unveil customer sentiments, gauge brand reputation, and derive competitive insights. This process also enhances predictive analytics and helps in developing models that can anticipate market trends or consumer behaviors.
Key Features to Look For
When selecting text analysis software, certain key features should be prioritized:
- Natural Language Processing Capabilities: The software should effectively understand and manipulate human language. This includes tokenization, parsing, and named entity recognition.
- Integration Capabilities: Compatibility with other data sources or platforms is important for maximizing the software's utility.
- User-Friendly Interface: An intuitive interface facilitates ease of use, enabling users to focus on results rather than navigating complex menus.
- Visualization Tools: The ability to visualize data through charts and graphs aids in the interpretation of analytical results.
- Scalability: As data volume grows, the software should maintain performance without degradation.
"In a world awash with data, the ability to analyze text efficiently could distinguish successful organizations from those that fall behind."
Having an understanding of these facets will help users identify the right text analysis solution that aligns with their specific needs and objectives.
Types of Text Analysis Techniques
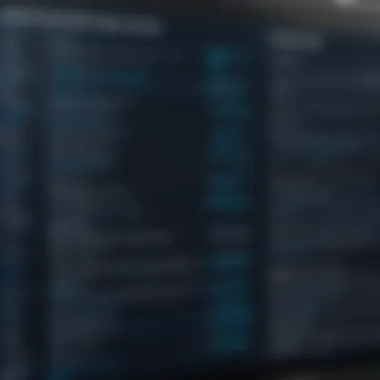
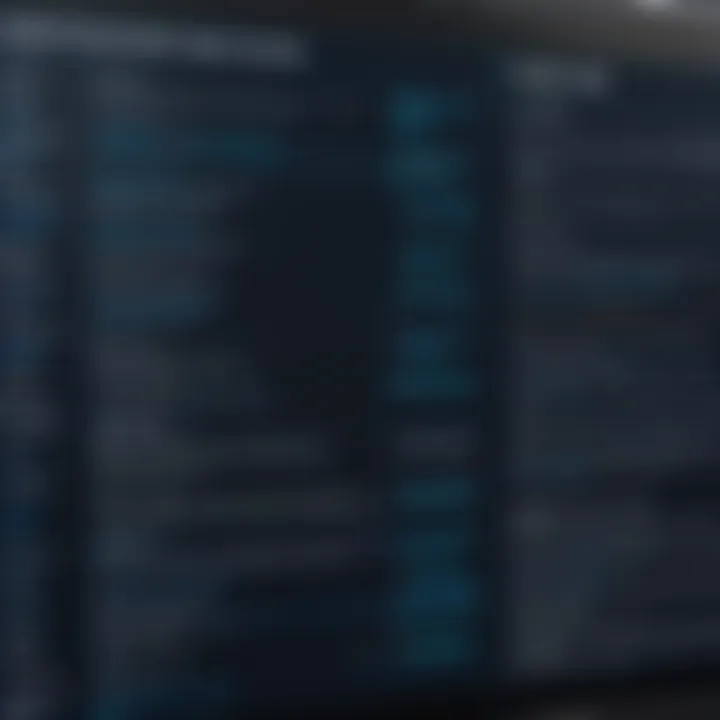
Text analysis software employs various techniques to extract meaningful data from unstructured text. Understanding these techniques is crucial, as they determine how effectively one can analyze large sets of textual information. By knowing the different methods available, users can select the technique that best suits their needs. Each technique offers unique advantages and considerations that can significantly impact the analysis outcome.
Sentiment Analysis
Sentiment analysis, often referred to as opinion mining, involves determining the emotional tone behind a body of text. This technique is essential for businesses seeking to understand customer sentiments regarding their products or services. By analyzing social media posts, customer reviews, and feedback, organizations can gauge public perception and respond accordingly.
Key benefits of sentiment analysis include:
- Real-time feedback: Companies can swiftly analyze public reactions to marketing campaigns or product launches.
- Market research: Understanding sentiments can help tailor products according to customer preferences.
- Crisis management: Rapid identification of negative sentiments enables proactive damage control.
However, challenges exist. Sentiment analysis might struggle with sarcasm or context-specific phrases. Thus, it's essential to choose robust software that can adapt to these nuances.
Topic Modeling
Topic modeling is a technique that identifies topics within a large collection of texts. It helps group similar documents by revealing underlying themes. Businesses utilize this method to organize their content or analyze trends in user-generated content.
Benefits of topic modeling include:
- Data organization: Helps in categorizing documents for better manageability.
- Trend detection: Can reveal emerging topics, assisting businesses in content strategy.
- Improved searchability: Enhances the ability to find relevant documents quickly.
Common algorithms like Latent Dirichlet Allocation (LDA) are employed in topic modeling. While effective, users must be cautious of the chosen algorithm's parameters, as these can greatly influence the results.
Text Classification
Text classification involves categorizing text into predefined groups, making it a vital component for applications like spam detection, sentiment categorization, and more. This technique is particularly useful for businesses looking to automate processes and improve efficiency.
Some key advantages are:
- Automation of workflows: Reduces manual tasks by automating document sorting.
- Enhanced accuracy: Automated systems often reduce human error in categorization.
- Scalability: Facilitates handling large volumes of text data efficiently.
Text classification can be based on supervised or unsupervised learning methods. Users must consider the volume and variability of their dataset closely to choose the right approach, ensuring effective outcomes.
Leading Text Analysis Software Solutions
Leading text analysis software solutions play a pivotal role in extracting meaningful insights from large volumes of unstructured data. In a world increasingly dominated by digital communication and vast text resources, having the right software can catalyze decision-making processes across various sectors. This section delves into top software offerings, assessing their unique functionalities and comparative advantages. Such understanding is crucial for organizations seeking to leverage text data effectively.
Overview of Top Software
When considering text analysis software, several prominent solutions come to mind. Each has distinct features tailored toward different user needs. Notable tools include:
- IBM Watson Natural Language Understanding: This tool stands out for its advanced sentiment analysis and entity recognition capabilities. It caters to businesses that require nuanced insights from customer feedback or social media interactions.
- Microsoft Azure Text Analytics: With seamless integration into broader data workflows and services, this tool is ideal for developers. It offers features like language detection and key phrase extraction, suitable for large-scale applications.
- RapidMiner: It provides a flexible environment for machine learning and text mining. RapidMiner allows users to create custom workflows, making it a favorite among data scientists.
Each of these tools serves specific use cases, enabling diverse applications from business intelligence to academic research.
Comparison of Features
Comparing features across text analysis software can help users make informed choices based on their unique requirements. Here are several parameters to consider:
- Ease of Use: Some tools prioritize user-friendliness, while others may offer more complex functionalities that require technical skill. For example, RapidMiner provides a drag-and-drop interface, making it accessible to less technical users.
- Customization: The ability to tailor the software for specific needs is critical. IBM Watson allows for extensive customization of models, ensuring that businesses can adapt the software to their unique contexts.
- Integration Capability: Integration with existing systems is vital for many organizations. Microsoft Azure facilitates easy integration with other Azure services, which can be beneficial for those already using the Azure ecosystem.
- Cost Structure: Pricing models vary. Some tools operate on a subscription basis, while others may require upfront investment. This aspect should align with budgetary considerations of the organization.
Comparing these features can shed light on which software aligns best with operational aims.
"The choice of software should consider both current and future needs, balancing feature richness with ease of implementation."
As businesses increasingly rely on textual data, selecting the appropriate text analysis software will become more critical. Understanding the available options and their features enables better decision marking and maximizes the value extracted from text data.
Reviews of Specific Text Analysis Tools
Understanding the specific text analysis tools available in the market is crucial for anyone looking to implement text analytics effectively. This section will explore individual tools in detail, focusing on their distinct characteristics, performance capabilities, use cases, and user feedback.
Tool A: Features and Performance
When assessing any text analysis software, one should prioritize the features and performance they offer. Tool A is notable for its robust functionality, which includes multiple text processing options such as entity recognition, keyword extraction, and sentiment analysis. These features enable users to derive valuable insights from large volumes of text data rapidly.
The performance metrics of Tool A are impressive, especially when handling extensive datasets. Users have reported that the software operates efficiently without significant lagging, even when processing thousands of documents simultaneously. The ability to customize the analysis parameters further enhances its usability.

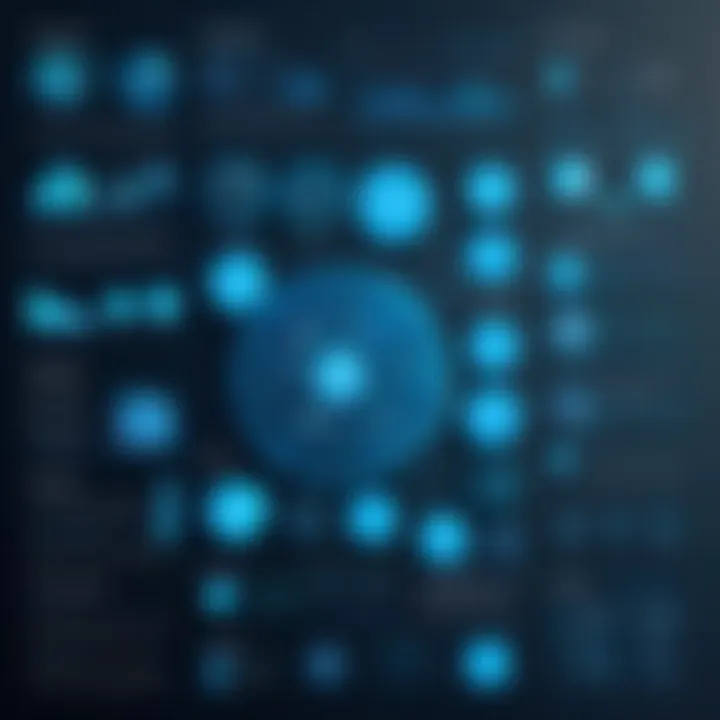
Both accuracy and speed are essential elements of any text analysis tool. In that regard, Tool A excels by providing highly precise results while maintaining a fast processing time. This balance is essential for businesses that rely on data-driven decision-making.
Tool B: Use Cases
Tool B stands out for its versatility across various sectors. It is particularly beneficial in fields such as market research, customer feedback analysis, and academic research.
- Market Research: Companies utilize Tool B to analyze consumer sentiment based on social media trends and reviews. This allows for more informed product development.
- Customer Feedback: Organizations employ this tool to sift through customer feedback and identify common themes or issues. Understanding these patterns is essential for improving service and product offerings.
- Academic Research: Researchers benefit from Tool Bโs capabilities to analyze text across numerous publications, helping to identify trends and gaps in existing studies.
The diverse applications of Tool B demonstrate its adaptability, making it a popular choice among various professionals.
Tool C: User Feedback
User feedback plays a significant role in determining the effectiveness of a text analysis tool. Tool C has received a mix of reviews, which provide valuable insights into its performance and utility.
Many users appreciate its user-friendly interface and accessibility, making it suitable for both technical and non-technical individuals. However, some have noted that the tool can be limited in terms of customization options for advanced users.
Additional comments highlighted the customer support offered by the developers behind Tool C. Quick responses to inquiries and a helpful learning resource section were frequently praised. This support system is essential for new users trying to navigate the softwareโs features.
Tool C also benefits from a strong online community. Users discuss their experiences on platforms like Reddit and share tips for maximizing the softwareโs use.
In summary, while Tool C has its limitations, constructive feedback from users offers potential insights for prospective users. Each tool discussed has its unique strengths, and understanding these helps in selecting the right text analysis software for individual needs.
Practical Applications of Text Analysis Software
Text analysis software serves numerous practical applications across various fields. Understanding these applications allows users to harness the power of data more effectively. Text analysis sheds light on trends, sentiments, and unspoken patterns within large datasets. Companies, researchers, and social media managers benefit significantly by applying text analysis tools to their workflows. The benefits stretch across diverse industries, yielding insightful data that guides decision-making processes.
In Business Intelligence
In the realm of business intelligence, text analysis software becomes a powerful ally. Companies regularly deal with vast amounts of unstructured data from emails, feedback forms, and social media. Using tools like IBM Watson or RapidMiner, businesses can analyze customer sentiments and preferences.
Sophisticated algorithms identify positive or negative trends, enabling companies to make informed strategic decisions. Consider a retail brand that uses text analysis to gauge customer reviews. By understanding customer sentiments, the brand can adapt its offerings or marketing strategies promptly. Similarly, identifying frequently mentioned themes in feedback can direct product development and improvement efforts.
- Enhances decision-making: Companies discover crucial insights that lead to more effective strategies.
- Reduces risk: Helps in understanding potential issues before they escalate.
- Improves customer satisfaction: Effectively addresses concerns and builds stronger relationships.
In Academic Research
Academic research also benefits from text analysis tools, which help break down and interpret large quantities of written material. Researchers can use software to examine scholarly articles, theses, or public records. Academic tools like NVivo or Atlas.ti are tailored for social scientists investigating patterns or trends in qualitative data.
The ability to automate the analysis of extensive texts saves researchers significant time. Instead of manually coding data, they can simply input their materials into the software and run analyses.
- Facilitates qualitative research: Text analysis streamlines the process of coding and identifying key themes.
- Encourages new insights: Automating data processing can reveal patterns that might go unnoticed.
- Supports collaboration: Shared outputs allow multiple researchers to access and discuss findings easily.
In Social Media Monitoring
Social media monitoring is a crucial application for text analysis software, as public perception often shifts rapidly. Organizations utilize platforms like Brandwatch or Hootsuite to track mentions of their brand across different social channels. Through sentiment analysis, they can assess the public's emotional response to product launches, campaigns, or major events.
This analysis guides social media strategies and helps brands engage effectively with their audience. Being able to respond to public sentiment in real time can enhance brand loyalty and mitigate negative feedback.
- Tracks brand reputation: Understands how the public perceives the brand over time.
- Informs marketing campaigns: Helps tailor messages based on audience sentiment.
- Identifies influencers: Recognizing key voices can bolster outreach efforts when promoting products or initiatives.
Remarkable insights can be gained from text analysis software. Understanding these practical applications is essential to leverage its full potential across various fields.
By utilizing text analysis software, users can unlock valuable insights that lead to better decision-making, improved strategies, and overall enhanced outcomes in their respective domains.
Challenges in Text Analysis
Text analysis is a powerful tool with vast applications, yet it does not come without challenges. Understanding these challenges is essential for users to make informed decisions when selecting software. This section addresses three significant challenges: data quality issues, language and contextual limitations, and interpretability of results. By recognizing these obstacles, users can better navigate their text analysis journeys and enhance the outcomes of their projects.
Data Quality Issues
Data quality is crucial in text analysis. The accuracy and reliability of insights gained directly depend on the quality of the input data. Low-quality data can lead to misleading results. Common problems include noisy data, incomplete information, and inconsistent formats.
- Noise in data refers to irrelevant or redundant information that skews the analysis. For instance, social media content often includes hashtags, emojis, and unconventional spellings which can complicate processing.
- Incomplete data can distort the representation of an entire dataset. Missing data fields can prevent algorithms from reaching accurate conclusions.
- Inconsistent formats pose another challenge. When input data is not standardized, it may hinder tools' ability to effectively parse and analyze it.
Addressing data quality often requires preprocessing steps like cleaning, normalization, and validation. Taking the time to ensure data quality pays off in the long run, enhancing the overall reliability of the analysis.
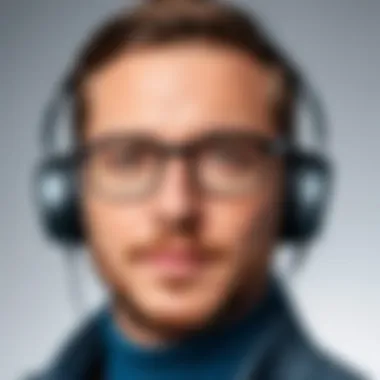
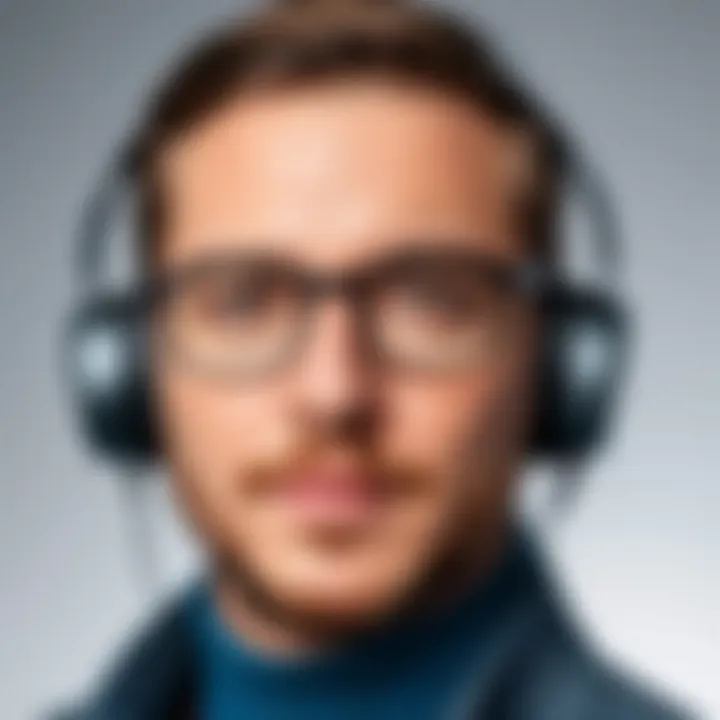
Language and Contextual Limitations
Language is a complex and evolving entity, making text analysis inherently challenging. Each language has unique characteristics, idioms, and structures, which can complicate the processing of text data.
- Polysemy, or words that have multiple meanings, can confuse analysis tools. For example, the word "bark" can refer to the sound a dog makes or the outer layer of a tree. Context is crucial for understanding which meaning applies.
- Slang and colloquial expressions can further introduce variability. Text analysis tools might not interpret these expressions correctly, leading to inaccurate sentiment readings.
- Cultural context plays a role as well. Certain statements may carry different meanings across cultures, impacting user intent interpretation.
To mitigate these limitations, it can be beneficial to choose software that incorporates advanced natural language processing capabilities. Leveraging multiple data sources and linguistic resources can also help enhance overall accuracy.
Interpretability of Results
Interpretability is vital for any analysis process. Users must understand the results generated by text analysis tools. Results that are not easily interpretable can hinder decision-making, particularly in critical fields like healthcare or finance.
- Black box models, prevalent in machine learning, can produce accurate results but often lack transparency in how they arrived at those results. Being unable to comprehend the decision-making process may lead to mistrust in the findings.
- Visualization tools can play a key role in improving interpretability. Tools that present results through visual means like charts or graphs can make complex information more digestible.
- User education is also important. Providing training and resources about how to interpret data allows users to gain confidence in the insights they derive.
Future Trends in Text Analysis Software
As the landscape of technology continuously evolves, so too does the domain of text analysis software. Keeping abreast of future trends is critical. The integration of advanced methodologies and the adaptation of existing solutions are likely to enhance both efficiency and effectiveness in text analysis. Understanding these trends enables software developers, IT professionals, and students to make informed decisions about tool selection and application. This section explores key emerging technologies, integration with machine learning, and ethical considerations that should shape the future of text analysis software.
Emerging Technologies
Emerging technologies play a significant role in transforming text analysis software. Key innovations include natural language processing (NLP), which helps in understanding and generating human language. NLP innovations can range from sentiment analysis that discerns emotional undertones in text to advanced text generation that mimics human writing.
- Deep Learning Models: These models, like transformers, have revolutionized the way text is processed and analyzed. Their ability to capture context over long passages is a game-changer in areas such as summarization and translation.
- Cloud Computing: This allows users to access powerful computing resources remotely, increasing accessibility and facilitating scalability for text analysis tasks.
- Real-Time Data Processing: With the rise of social media and continuous content creation, real-time analytics is becoming vital. This enables organizations to offer timely insights and respond quickly to issues.
Integration with Machine Learning
The integration of machine learning into text analysis software is not just a trend; it is becoming essential for achieving advanced analytics capabilities. Machine learning algorithms can learn from vast datasets, improving accuracy over time. For instance, they can be trained to classify text, identify patterns, and extract insights efficiently.
- Tailored Analytics: By leveraging machine learning, organizations can develop customized solutions. It allows for fine-tuning models to specific domains or sectors, thus improving relevance and applicability.
- Predictive Analysis: Machine learning techniques can be employed to predict trends and behaviors based on past data, allowing users to make proactive business decisions.
- Enhanced Automation: Repetitive tasks such as data entry and categorization can be automated, freeing up human resources for more strategic functions.
Ethical Considerations
With advancements in text analysis software, ethical considerations must be prioritized. As data privacy becomes a focal point for organizations, how data is handled and analyzed raises important questions.
- Data Consent and Privacy: Users must be careful with how they collect, store, and analyze data, particularly personal information. Transparency is vital in maintaining trust.
- Bias in Algorithms: Machine learning algorithms can inherit biases from training data. Continuous monitoring and adjustments are necessary to avoid perpetuating stereotypes or inaccuracies.
- Responsibility in Analytics: Professionals using text analysis tools must remain aware of the implications of their findings. Ethical use of insights, especially concerning user data, is critical for maintaining integrity in analytics.
The future of text analysis is shaped by technology, ethics, and user responsibility. Understanding these trends ensures that professionals remain prepared and equipped.
Choosing the Right Text Analysis Software
Selecting the appropriate text analysis software is a crucial step for professionals, researchers, and students in the field. This decision can directly influence the effectiveness of data processing and interpretation. The variety of options available today makes it imperative to approach this selection strategically, as the wrong choice can hinder your workflow and the insights derived from your data.
Evaluating Your Needs
Before diving into the selection process, it is vital to conduct a thorough evaluation of your specific needs. Not all text analysis tools are created equal, and they often cater to different applications, ranging from sentiment analysis to data classification.
To assess your requirements, consider the following elements:
- Objective: What are you hoping to achieve with text analysis? Define your project's goals, whether it is academic research, business intelligence, or social media monitoring.
- Data Volume: Estimate the volume of data you will be processing. Some software manages large datasets more efficiently than others.
- Technical Skills: Be realistic about your team's technical capabilities. Some tools require advanced knowledge, whereas others offer more user-friendly interfaces.
Given these factors, aligning your choice with your unique situation is key. You may prioritize features like advanced machine learning capabilities if your project is research-oriented. Alternatively, a simpler solution might be more effective for straightforward business tasks.
Cost vs. Benefit Analysis
Financial considerations play a significant role when choosing text analysis software. Conducting a cost vs. benefit analysis can provide clarity on the investment you are about to make. Here, you need to look beyond the initial costs associated with licenses or subscriptions.
- Budget Constraints: Understand your budget limitations. Some tools are free, while others can be quite costly. Determine what fits your financial parameters.
- Feature Set vs. Price: Evaluate if the software's features justify its cost. For instance, if a tool provides extensive customization options that you require, it might be worth a higher price.
- Return on Investment (ROI): Analyze how the tool will impact productivity and facilitate data-driven decisions. A higher upfront cost may be justifiable if it significantly enhances your capabilities.
A careful balance between cost and potential benefits can lead to a more informed decision.
User Experience and Support
The final consideration in choosing text analysis software revolves around user experience and the support provided. Software that is difficult to navigate can lead to frustration, thereby reducing efficiency.
Important aspects to evaluate include:
- Usability: Assess the interface design. User-friendly software often results in faster onboarding and improved output.
- Documentation: Comprehensive manuals and online resources are invaluable. They help users to troubleshoot issues efficiently.
- Support Channels: Consider the support options available, such as customer service response times, community forums, or professional support services.
"Choosing the right tool shapes your path to effective analysis and data understanding."
As you move toward making a decision, conduct thorough research and evaluate options based on the outlined criteria.